The Role of Geostatistics in Predicting Landslide Risk
Introduction
Geostatistics is a branch of statistics that deals with the analysis, interpretation, and prediction of spatial phenomena. It is a vital tool in many fields, including geology, environmental science, and natural hazard assessment. One of the key applications of geostatistics is in the prediction of landslide risk. Landslides are a major natural hazard that can cause significant damage to property and loss of life. By using geostatistical methods, it is possible to predict the likelihood of a landslide occurring in a particular area, allowing for better planning and risk management Geostatistics.
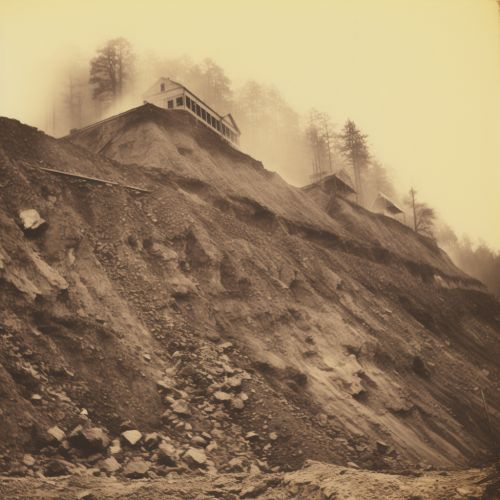
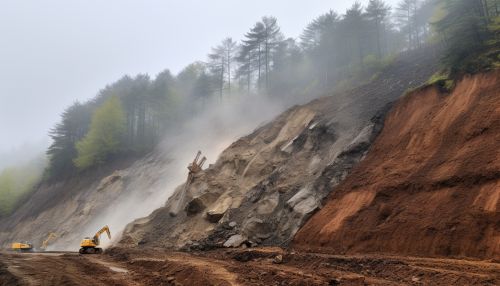
Geostatistics and Landslide Risk
Geostatistics is particularly well-suited to predicting landslide risk due to its ability to handle spatial data. Landslides are inherently spatial phenomena, occurring at specific locations and often influenced by the characteristics of the surrounding landscape. Geostatistical methods can take into account these spatial characteristics, providing a more accurate prediction of landslide risk than traditional statistical methods Spatial Data.
The key to geostatistical analysis is the concept of spatial autocorrelation. This is the idea that measurements taken at locations close to each other are more likely to be similar than measurements taken at locations further apart. In the context of landslide risk, this might mean that areas with similar topography, soil type, and rainfall patterns are more likely to experience landslides than areas with different characteristics Spatial Autocorrelation.
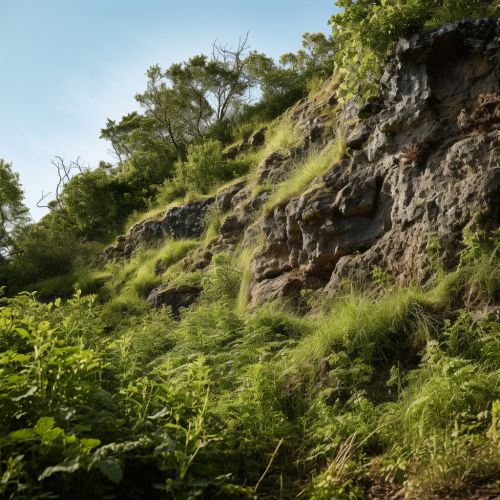
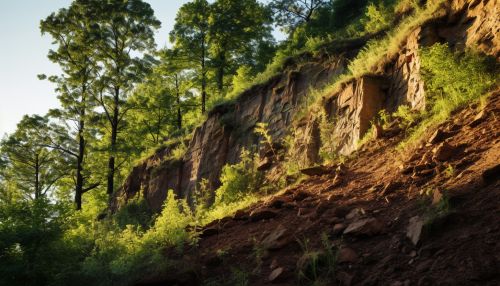
Methods of Geostatistical Analysis
There are several key methods of geostatistical analysis that are commonly used in landslide risk prediction. These include variogram analysis, kriging, and spatial regression.
Variogram analysis is a method used to quantify spatial autocorrelation. It involves plotting the variance of differences between measurements against the distance between the locations where the measurements were taken. The resulting plot, known as a variogram, can provide valuable insights into the spatial structure of the data, which can in turn inform the prediction of landslide risk Variogram Analysis.
Kriging is a method of spatial interpolation that uses variogram analysis to estimate values at unmeasured locations. In the context of landslide risk, kriging can be used to predict the likelihood of a landslide occurring at a particular location based on measurements taken at nearby locations Kriging.
Spatial regression is a method of statistical analysis that incorporates spatial autocorrelation into the regression model. This can provide a more accurate prediction of landslide risk by taking into account the spatial structure of the data Spatial Regression.
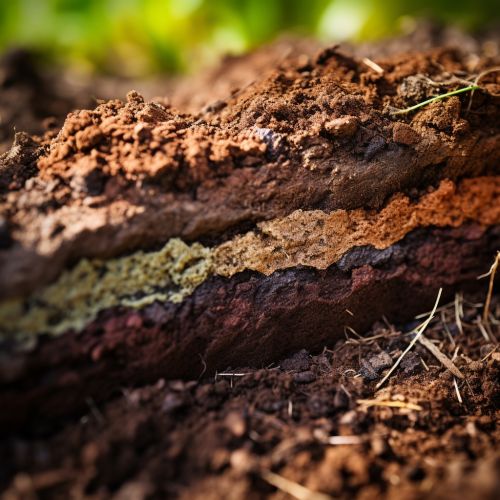
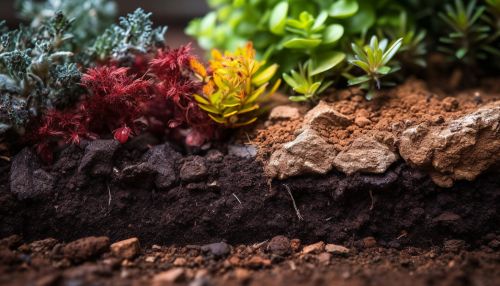
Applications of Geostatistics in Landslide Risk Prediction
Geostatistics has been successfully applied in a number of studies to predict landslide risk. These studies have used a variety of geostatistical methods, including variogram analysis, kriging, and spatial regression, to analyze spatial data and predict the likelihood of landslides occurring.
One of the key benefits of using geostatistics in landslide risk prediction is its ability to handle large datasets. Many studies of landslide risk involve collecting data from a large number of locations, which can be difficult to analyze using traditional statistical methods. Geostatistical methods, on the other hand, are designed to handle large datasets, making them an ideal tool for landslide risk prediction Large Datasets.
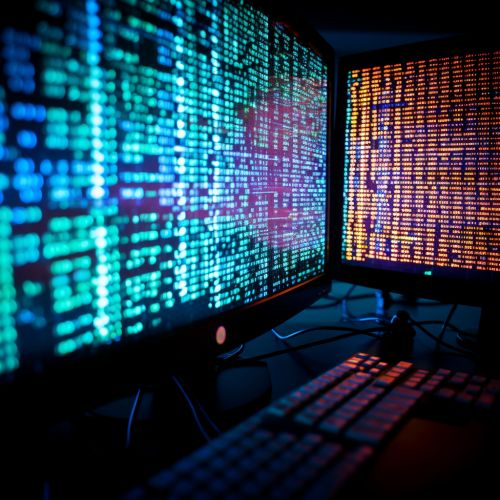
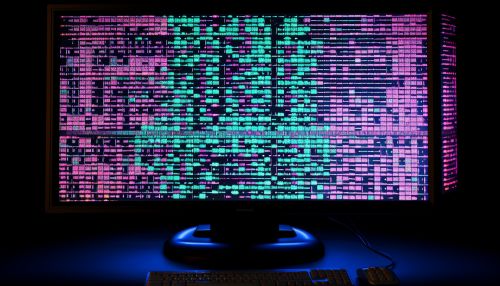
Limitations and Challenges
While geostatistics offers many advantages in predicting landslide risk, it also presents some challenges. One of the main limitations is the assumption of stationarity, which assumes that the spatial structure of the data is constant over the study area. This assumption may not hold true in all cases, particularly in complex landscapes with varying topography and soil types Stationarity.
Another challenge is the need for high-quality spatial data. The accuracy of geostatistical predictions depends on the quality of the input data, and collecting accurate spatial data can be time-consuming and expensive Spatial Data Quality.
Despite these challenges, geostatistics remains a powerful tool for predicting landslide risk, and ongoing research continues to improve the methods and techniques used in geostatistical analysis.
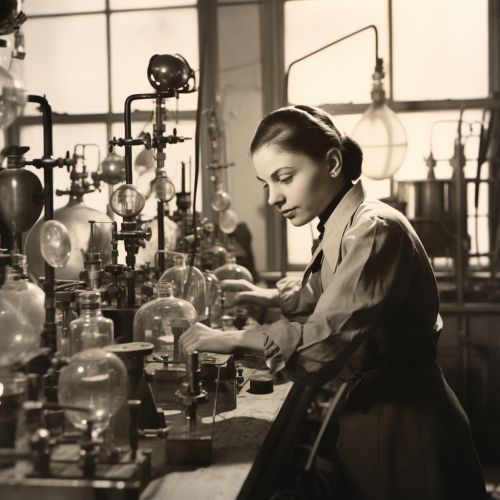
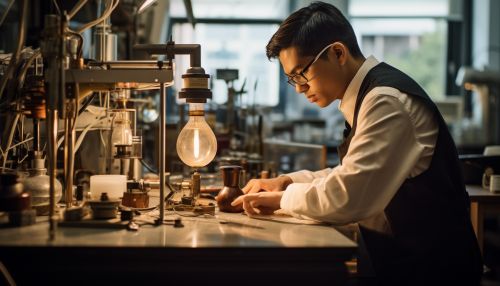