Geostatistics
Introduction
Geostatistics is a branch of statistics focused on spatial or spatiotemporal datasets. Developed originally to predict probability distributions of ore grades for mining operations, it is currently applied in diverse disciplines including petroleum geology, hydrogeology, hydrology, meteorology, oceanography, geochemistry, geometallurgy, geography, forestry, environmental control, landscape ecology, soil science, and agriculture (agronomy, animal science, plant science).
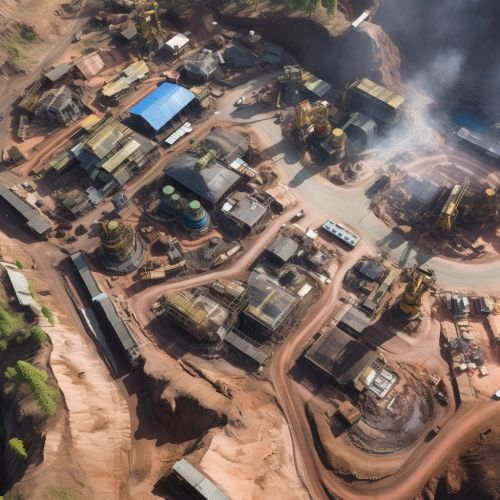
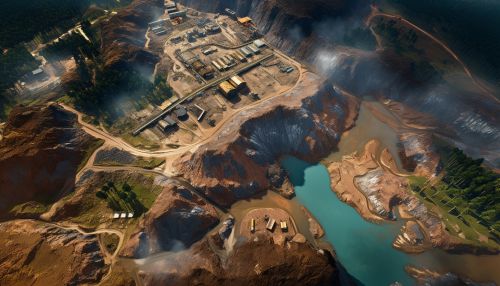
History
The development of geostatistics as a distinct discipline originated from the work of the South African geologist D. G. Krige in the 1950s, and later, French engineer Georges Matheron in the 1960s. Krige sought a method to estimate the most probable distribution of mineral grades based on samples from a mining area, which led to the development of a technique known as kriging. Matheron expanded on Krige's work, eventually laying the groundwork for the formulation of the theory of regionalized variables.
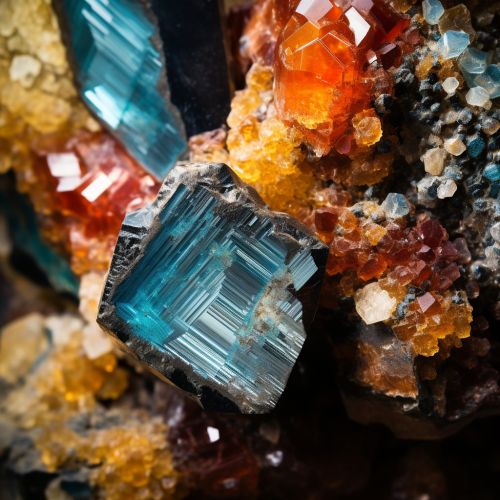
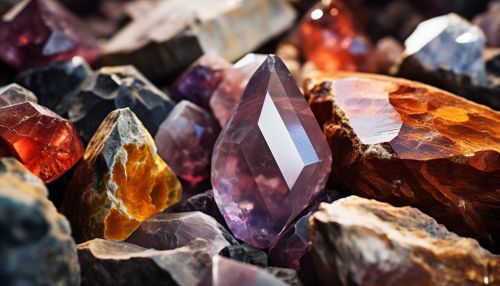
Fundamental Concepts
Geostatistics operates under the premise that spatial variation in any variable (e.g., the concentration of a certain mineral in an ore body, the porosity or permeability of a reservoir, the fertility of a soil, etc.) is not random, but follows a certain degree of spatial correlation. This spatial correlation is quantified and described through the use of semivariograms and correlograms, which are graphical representations of the degree of spatial correlation as a function of distance.
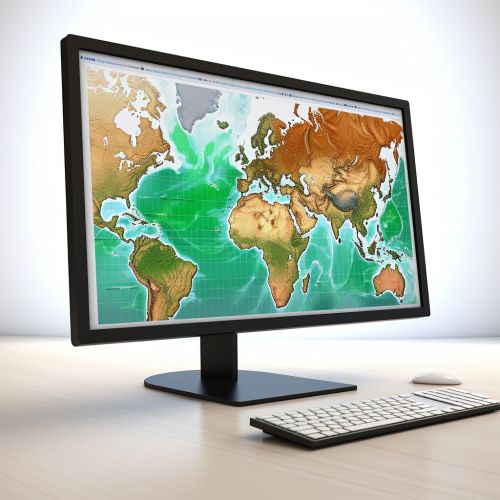
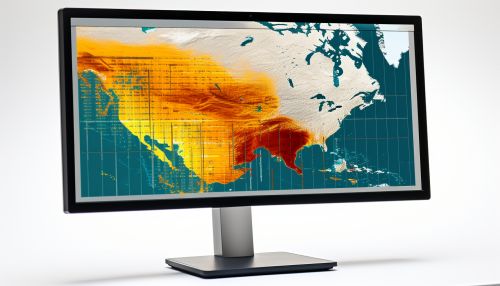
Regionalized Variable Theory
The theory of regionalized variables, as proposed by Matheron, is a key concept in geostatistics. It posits that every variable studied in geostatistics is a regionalized variable, i.e., a variable that varies continuously over space. This is in contrast to the classical statistical view of variables as being independent of each other.
Semivariogram
The semivariogram is a function describing the degree of spatial dependence of a regionalized variable. It is defined as the half of the average of the squared differences between all pairs of data points separated by a certain lag distance.
Kriging
Kriging is a method of interpolation used in geostatistics to predict unknown values of a regionalized variable at unsampled locations, based on the values of the variable at sampled locations. It is named after its inventor, Danie Krige.
Applications
Geostatistics has found application in a wide range of fields, from mining and petroleum geology to agriculture and meteorology. In each of these fields, it is used to predict the spatial distribution of a certain variable, based on a limited number of sample data points.
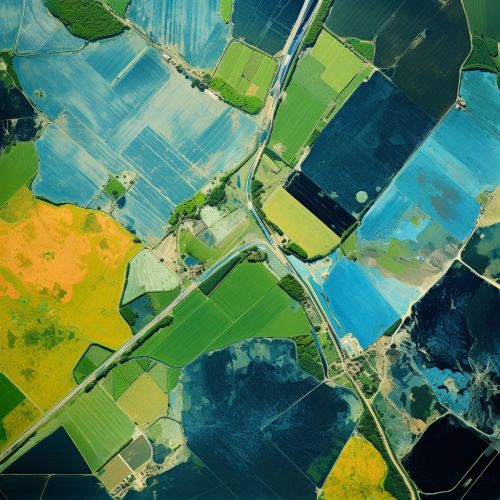
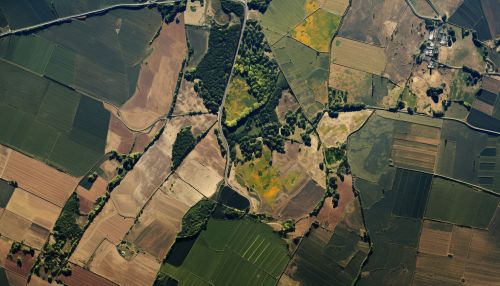
Mining and Petroleum Geology
In mining and petroleum geology, geostatistics is used to estimate the distribution of ore grades or hydrocarbon accumulations in the subsurface. This is crucial in the planning of mining or drilling operations, as it allows for the optimization of resource extraction.
Agriculture
In agriculture, geostatistics can be used to map the spatial distribution of various soil properties (e.g., fertility, moisture content, etc.), or the occurrence of pests and diseases. This information can then be used to guide precision farming practices, such as variable rate fertilization or targeted pest control.
Meteorology and Oceanography
In meteorology and oceanography, geostatistics is used to interpolate meteorological or oceanographic parameters (e.g., temperature, pressure, wind speed, etc.) from a network of observation points. This is crucial in weather forecasting and climate modeling.
Limitations and Criticisms
Despite its wide application, geostatistics is not without its limitations and criticisms. One of the main criticisms is that it assumes stationarity, i.e., the statistical properties of the variable being studied do not change over space. This assumption is often violated in natural systems, leading to potential inaccuracies in the predictions made by geostatistical models.
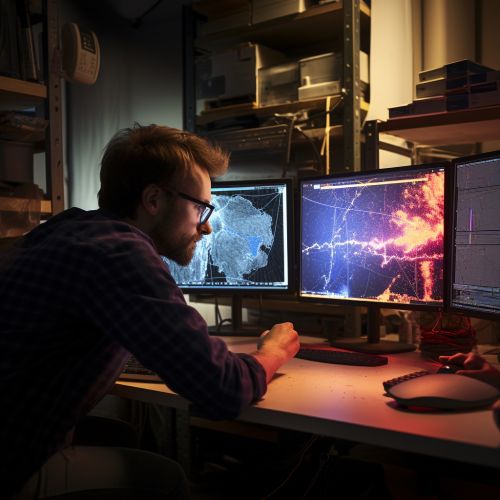
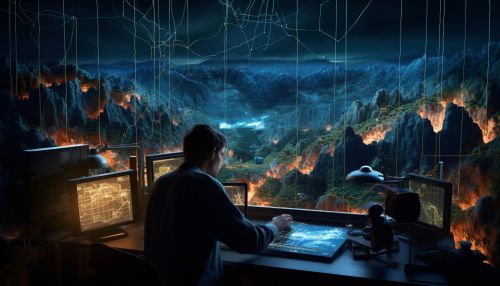
Future Directions
With the advent of new technologies such as remote sensing and geographic information systems (GIS), the potential applications of geostatistics are expanding. In particular, the integration of geostatistics with GIS has opened up new possibilities for spatial data analysis and modeling. Furthermore, the development of non-stationary geostatistical models is an active area of research, aimed at overcoming one of the main limitations of traditional geostatistics.
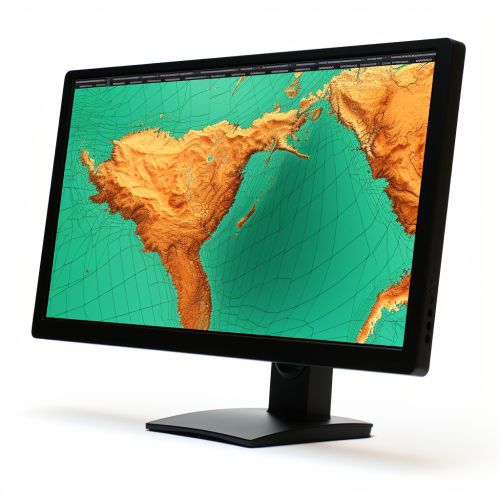
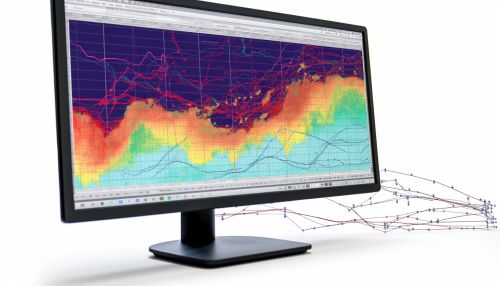
See Also
- Spatial Analysis
- Geographic Information System
- Remote Sensing
- Precision Agriculture
- Environmental Statistics