Stationarity
Introduction
Stationarity, in the context of time series analysis, refers to a statistical concept that a process generating a time series data does not change over time. This means that the properties of the system, such as mean, variance, and autocorrelation, remain constant over time.
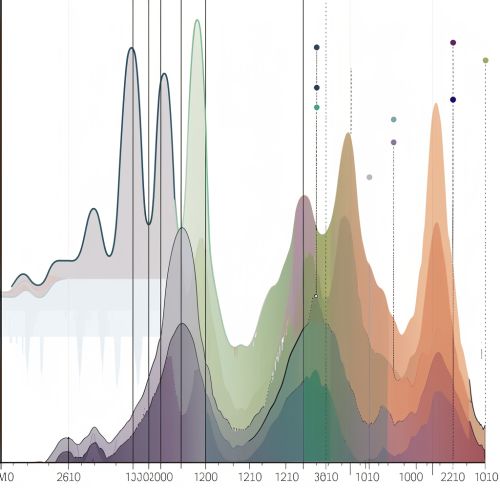
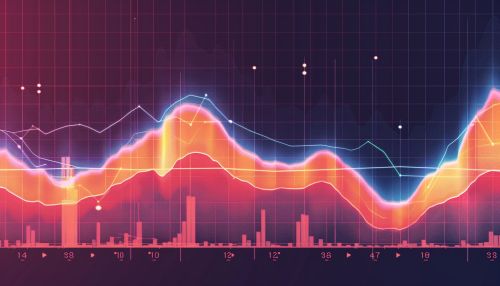
Definition
A time series is said to be stationary if its statistical properties do not change over time. In other words, it has constant mean and variance, and the covariance between two time periods depends only on the distance or gap or lag between the two time periods and not the actual time at which the series is observed. This is known as strict or strong stationarity.
However, in practice, a weaker form of stationarity, known as weak or wide-sense stationarity, is often used. In this case, the series need only have constant mean, variance and autocorrelation over time.
Importance of Stationarity
Stationarity is an important assumption in many statistical and econometric models. If a time series is stationary, it is easier to model and make predictions. This is because the structure of the series does not change over time, so the model can focus on learning patterns rather than adapting to changing structures.
Non-stationary series, on the other hand, are more difficult to model and can lead to unreliable and misleading statistics. This is because the underlying parameters of the series can change over time, leading to a model that is constantly trying to adapt to these changes.
Testing for Stationarity
There are several statistical tests that can be used to determine whether a time series is stationary or not. These include the Augmented Dickey-Fuller test, the Kwiatkowski-Phillips-Schmidt-Shin test, and the Phillips-Perron test. These tests have null hypotheses that the series is non-stationary. If the test statistic is less than the critical value, we reject the null hypothesis and infer that the series is stationary.
Making a Series Stationary
If a time series is found to be non-stationary, there are several techniques that can be used to make it stationary. These include differencing, where the difference between consecutive observations is calculated, and transformations such as logarithms, square roots, or reciprocals, which can stabilize the variance of a series.
Applications of Stationarity
Stationarity is a key concept in many areas of applied statistics and econometrics, including finance, economics, and environmental science. It is used in the development of models for stock prices, economic indicators, and climate data, among others.