Point Pattern Analysis
Introduction
Point Pattern Analysis (PPA) is a branch of spatial statistics that focuses on the spatial arrangement of points in a given space. It is a powerful tool used in various fields such as ecology, geography, epidemiology, and urban planning, among others. The primary goal of PPA is to identify patterns within a set of data points and determine if these patterns occur randomly or exhibit some form of structure.
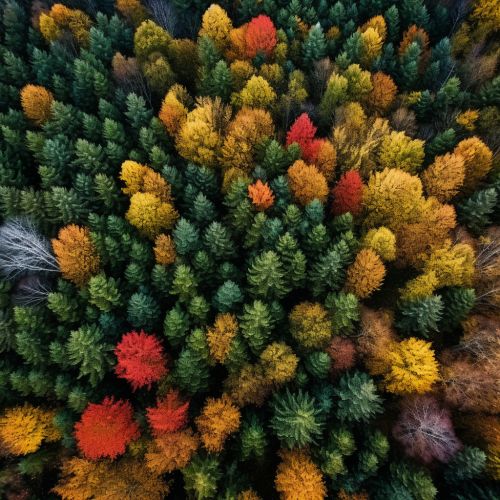
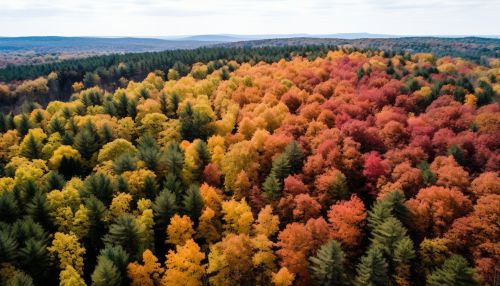
Theoretical Background
PPA is rooted in the mathematical field of stochastic geometry, which studies random spatial patterns. The basic premise of PPA is that the spatial distribution of points can provide significant insights into the underlying processes that generated them. For instance, the distribution of trees in a forest can reveal information about the ecological processes at play, such as competition for resources or the spread of diseases.
Types of Point Patterns
Point patterns can be broadly classified into three types:
- Complete Spatial Randomness (CSR): This is the simplest type of point pattern, where each point is independently and randomly distributed across the space. CSR is often used as a null model in PPA to test if an observed pattern deviates from randomness.
- Clustered: In a clustered point pattern, points tend to group together. This type of pattern is often observed in nature, such as the distribution of trees in a forest or the spread of a contagious disease in a population.
- Regular or Uniform: In a regular or uniform point pattern, points are evenly distributed across the space. This type of pattern is less common in nature but can be observed in human-made structures, such as the grid-like layout of a city.
Methods of Point Pattern Analysis
There are several methods used in PPA, each with its strengths and limitations. Some of the most commonly used methods include:
- Quadrat Analysis: This is a simple and intuitive method that involves dividing the study area into equally sized squares (quadrats) and counting the number of points in each quadrat. The resulting counts are then compared to what would be expected under CSR.
- Nearest Neighbor Analysis: This method involves measuring the distances between each point and its nearest neighbor. The average of these distances is then compared to what would be expected under CSR.
- Ripley's K-function: This is a more sophisticated method that considers all pairwise distances between points. The K-function provides a measure of the cumulative number of other points within a given distance of each point, providing a more comprehensive view of the point pattern.
Applications of Point Pattern Analysis
PPA has a wide range of applications across various fields. Some examples include:
- Ecology: Ecologists use PPA to study the spatial distribution of species in an ecosystem. This can provide insights into species interactions, habitat preferences, and ecological processes.
- Epidemiology: In epidemiology, PPA can be used to study the spread of diseases. For instance, it can help identify disease clusters and provide insights into the transmission dynamics of infectious diseases.
- Urban Planning: Urban planners use PPA to analyze the spatial distribution of various urban features, such as buildings, parks, and roads. This can inform decisions about urban development and resource allocation.