Nearest Neighbor Analysis
Introduction
Nearest Neighbor Analysis (NNA) is a method used in spatial analysis and geography to determine the degree of clustering in a set of points. It is a technique that allows researchers to measure the expected and observed distances between neighboring points in a particular area. This method is commonly used in fields such as geostatistics, ecology, and urban planning.
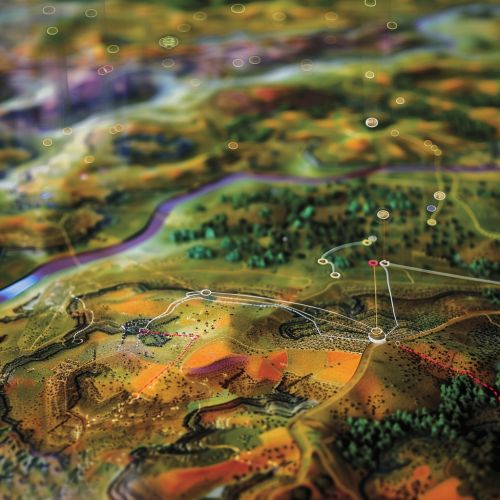
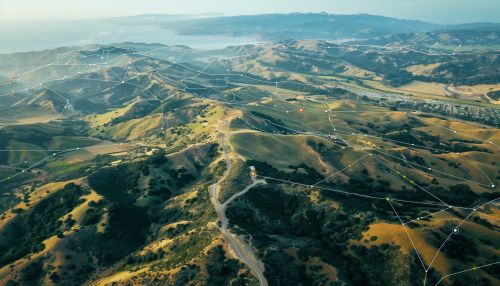
Theory
The theory behind NNA is rooted in the concept of spatial distribution. This refers to how objects, features, or phenomena are distributed in space. The method uses the principles of probability theory and statistics to calculate the expected distances between points if they were randomly distributed. The observed distances are then compared to these expected distances to determine whether the points are clustered, randomly distributed, or uniformly distributed.
Methodology
The methodology of NNA involves several steps. First, the researcher must define the study area and the points of interest. These points could represent anything from individual organisms in an ecological study to buildings in an urban planning context.
Next, the distances between each point and its nearest neighbor are calculated. This is typically done using a Geographic Information System (GIS) or similar software. The average of these distances is then calculated to give the observed mean distance.
The expected mean distance is calculated based on the assumption of a random distribution. This is done using the formula: E(D) = 0.5/√(n/A), where n is the number of points and A is the area of the study region.
Finally, the Nearest Neighbor Index (NNI) is calculated using the formula: NNI = Dobs / Dexp, where Dobs is the observed mean distance and Dexp is the expected mean distance. If the NNI is less than 1, the points are considered to be clustered. If it is greater than 1, they are considered to be uniformly distributed. If it is approximately 1, they are considered to be randomly distributed.
Applications
NNA has a wide range of applications in various fields. In ecology, it can be used to study the distribution of organisms in a habitat. In urban planning, it can help in understanding the distribution of features such as buildings or parks. In public health, it can be used to identify clusters of disease occurrence.
Limitations
While NNA is a powerful tool, it has its limitations. It assumes that the study area is homogeneous, which may not always be the case. It also assumes that all points are independent of each other, which may not be true in cases where there is spatial autocorrelation. Furthermore, it can only analyze point data, and cannot be used for line or area data.