Spatial Statistics
Introduction
Spatial statistics is a branch of statistics that deals with the analysis and interpretation of spatial or spatiotemporal data. It involves the application of statistical concepts and methods to data that are not independent, but rather, are spatially correlated. This field of study is essential in various disciplines such as geography, environmental science, epidemiology, and urban planning, among others.
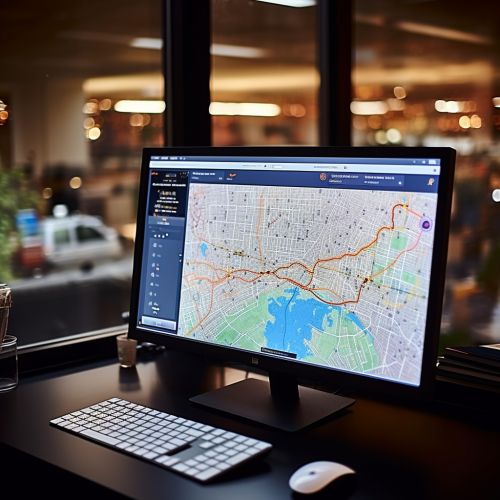
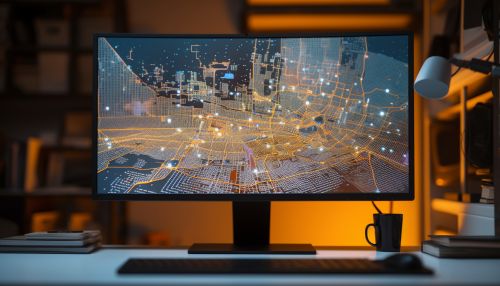
Concept and Importance
Spatial statistics is a unique field of study that focuses on the spatial or geographical aspect of data. It is concerned with the location, distribution, pattern, and process of spatial data. The primary objective of spatial statistics is to understand and explain the spatial variability of a particular phenomenon. It employs various statistical techniques to analyze spatial data, which can be point-referenced data, areal data, or spatial point process data.
The importance of spatial statistics lies in its ability to provide significant insights into spatial processes and patterns. It allows researchers and scientists to make predictions and inferences about spatial data, which can be crucial in decision-making processes in various fields.
Types of Spatial Data
Spatial data can be classified into three main types: point-referenced data, areal data, and spatial point process data.
Point-Referenced Data
Point-referenced data, also known as geostatistical data, are observations associated with a specific location in space. Examples of point-referenced data include the location of a disease outbreak, the concentration of a pollutant at a specific location, or the elevation of a place.
Areal Data
Areal data, also known as lattice data or areal unit data, are observations related to a specific area or region. These data are usually aggregated over an area, such as the population of a city, the average income of a county, or the total rainfall of a region.
Spatial Point Process Data
Spatial point process data are observations of events that occur in space. These data are often represented as points in a spatial domain. Examples of spatial point process data include the location of trees in a forest, the occurrence of earthquakes, or the distribution of crime incidents in a city.
Spatial Dependence and Autocorrelation
A key concept in spatial statistics is spatial dependence, which refers to the tendency of data points that are close together in space to have similar values. This is also known as spatial autocorrelation. Spatial autocorrelation can be positive, where nearby locations have similar values, or negative, where nearby locations have dissimilar values.
Spatial autocorrelation is an important concept because it violates the assumption of independence, which is a fundamental principle in traditional statistics. In spatial statistics, the assumption of independence is replaced by the First Law of Geography, which states that "everything is related to everything else, but near things are more related than distant things."
Methods and Techniques
Spatial statistics employs a wide range of methods and techniques to analyze spatial data. These include exploratory spatial data analysis (ESDA), spatial regression, spatial interpolation, and spatial point pattern analysis.
Exploratory Spatial Data Analysis (ESDA)
Exploratory spatial data analysis (ESDA) is a set of techniques used to describe and visualize spatial distributions, identify spatial patterns, and detect spatial outliers. ESDA techniques include spatial autocorrelation measures, such as Moran's I and Geary's C, and spatial visualization tools, such as spatial scatterplots and choropleth maps.
Spatial Regression
Spatial regression is a statistical method used to model spatial relationships. It extends traditional regression models by incorporating spatial dependence and spatial heterogeneity. There are two main types of spatial regression models: spatial lag models and spatial error models.
Spatial Interpolation
Spatial interpolation is a technique used to estimate values at unobserved locations based on observed data. Common spatial interpolation methods include kriging, inverse distance weighting (IDW), and spline interpolation.
Spatial Point Pattern Analysis
Spatial point pattern analysis is a set of techniques used to analyze the spatial arrangement of points in a spatial domain. These techniques include nearest neighbor analysis, Ripley's K function, and point process models.
Applications
Spatial statistics has a wide range of applications in various fields. In geography, it is used to analyze spatial patterns and processes. In environmental science, it is used to study the distribution and concentration of pollutants. In epidemiology, it is used to investigate the spatial distribution of diseases. In urban planning, it is used to analyze spatial patterns of urban development.