The Role of Geostatistics in Predicting Wind Patterns
Introduction
Geostatistics is a branch of statistics that deals with spatial or spatiotemporal datasets. It is a key tool in geographical information systems (GIS) and in the earth sciences. More recently, it has been increasingly applied in a range of diverse fields such as wind energy, environmental science, and agriculture. This article will focus on the role of geostatistics in predicting wind patterns, an important aspect of wind energy production and weather forecasting.
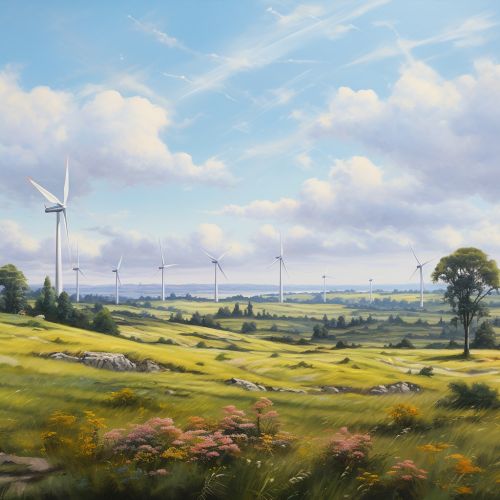

Geostatistics: An Overview
Geostatistics originated from the mining and petroleum industries, where it was used to predict the likelihood of finding valuable resources based on limited samples. The key concept in geostatistics is spatial dependence, which is the statistical dependence between values at different locations in space. This concept is often expressed through the semivariogram, a function that describes the degree of spatial dependence as a function of distance.
Wind Patterns and Their Importance
Wind patterns refer to the way wind moves across the earth's surface. They are influenced by a variety of factors, including the rotation of the earth, the distribution of land and sea, and the earth's topography. Understanding wind patterns is crucial for a number of applications, including weather forecasting, climate modeling, and the siting of wind turbines for energy production.


Geostatistics in Predicting Wind Patterns
Geostatistics offers a powerful set of tools for predicting wind patterns. These include kriging, a method of interpolation that takes into account the spatial dependence of wind speeds, and spatial autocorrelation, a measure of the degree to which wind speeds at one location are related to those at nearby locations.
Kriging and Wind Prediction
Kriging is a method of interpolation that was first developed in the mining industry. It is named after the South African mining engineer Danie Krige. In the context of wind prediction, kriging can be used to estimate wind speeds at unmeasured locations based on measurements at nearby locations. The method takes into account the spatial dependence of wind speeds, which means that wind speeds at nearby locations are more likely to be similar than those at distant locations.


Spatial Autocorrelation and Wind Prediction
Spatial autocorrelation is a measure of the degree to which wind speeds at one location are related to those at nearby locations. It is a key concept in geostatistics and is often used in conjunction with kriging to predict wind patterns. A positive spatial autocorrelation indicates that wind speeds at nearby locations are likely to be similar, while a negative spatial autocorrelation indicates that they are likely to be dissimilar.
Challenges and Future Directions
While geostatistics provides a powerful set of tools for predicting wind patterns, there are also challenges. One of the main challenges is the need for accurate and comprehensive wind speed data. Future directions in the field may include the development of new methods for collecting and analyzing wind speed data, as well as the integration of geostatistics with other predictive methods.

