The Role of Geostatistics in Predicting Air Quality
Introduction
Geostatistics is a branch of statistics that deals with spatial or spatiotemporal datasets. Developed originally to predict probability distributions of ore grades for mining operations, it is currently applied in diverse disciplines including petroleum geology, hydrogeology, hydrology, meteorology, oceanography, geochemistry, geographical information systems, remote sensing, epidemiology, climate change, air quality management, soil science, and forest management.
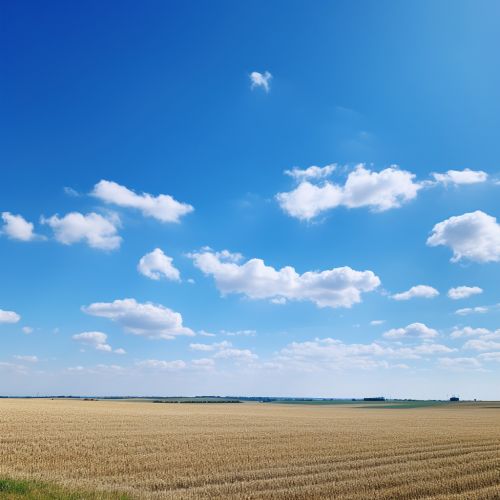
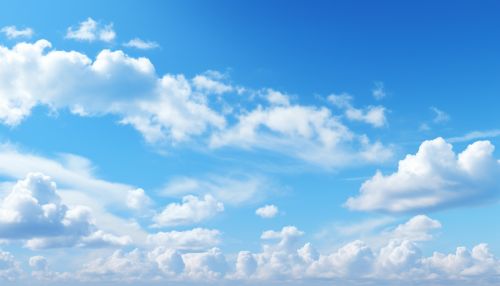
The Role of Geostatistics in Air Quality Prediction
Geostatistics plays a crucial role in predicting air quality by providing a statistical framework for spatial and temporal analysis. This is achieved through the application of various statistical methods and models that allow for the analysis of spatially referenced data. These methods include kriging, spatial autocorrelation, and spatial regression among others.
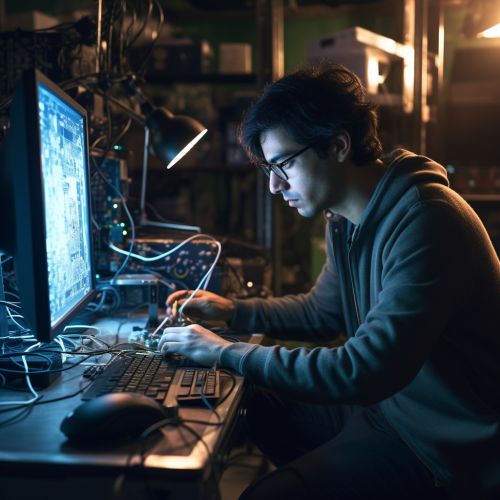
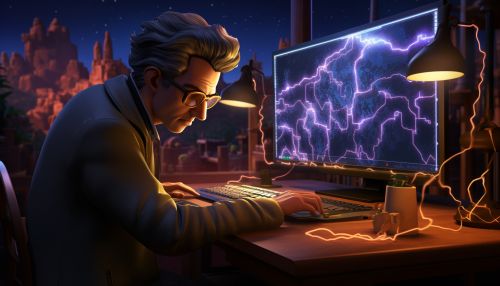
Kriging
Kriging is a geostatistical method used to interpolate or predict the value of a variable at an unmeasured location from observations made at nearby locations. In the context of air quality prediction, kriging can be used to estimate the concentration of pollutants at unmonitored locations based on measurements taken at nearby air quality monitoring stations.
Spatial Autocorrelation
Spatial autocorrelation is a measure of the degree to which a set of spatial features and their associated values tend to be clustered together in space. In terms of air quality prediction, spatial autocorrelation can be used to identify areas where air pollution levels are consistently high or low.
Spatial Regression
Spatial regression is a statistical method used to model spatial relationships. It is often used in air quality prediction to understand the relationship between air pollution levels and various factors such as traffic volume, industrial activity, and meteorological conditions.
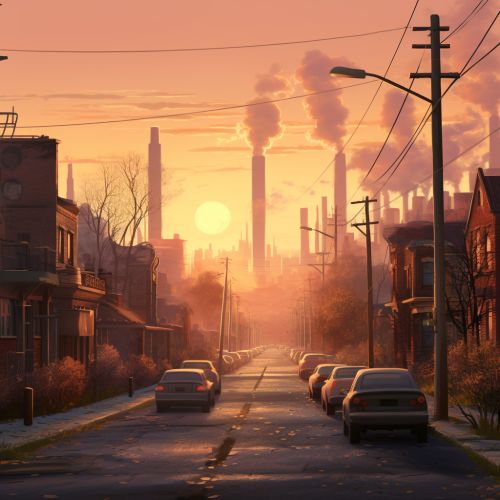
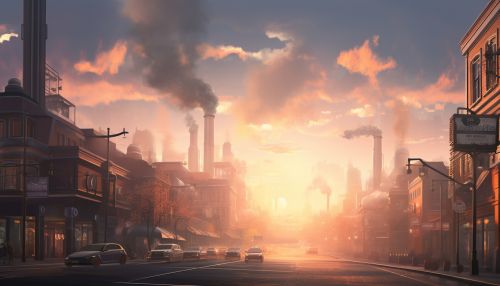
Applications of Geostatistics in Air Quality Prediction
Geostatistics is widely used in air quality prediction for various applications. These include air quality monitoring, air pollution exposure assessment, and air quality forecasting.
Air Quality Monitoring
Geostatistics is used in air quality monitoring to design optimal monitoring networks and to interpolate air pollution levels at unmonitored locations. This is crucial for identifying areas of high pollution and for informing air quality management strategies.
Air Pollution Exposure Assessment
In air pollution exposure assessment, geostatistics is used to estimate individual exposure to air pollution. This is often done by combining air quality data with information on individual activity patterns and residential locations.
Air Quality Forecasting
Geostatistics is also used in air quality forecasting to predict future air pollution levels. This is important for issuing air quality alerts and for planning air pollution control measures.
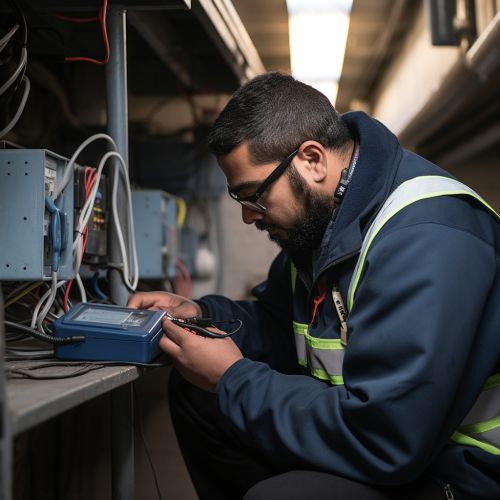
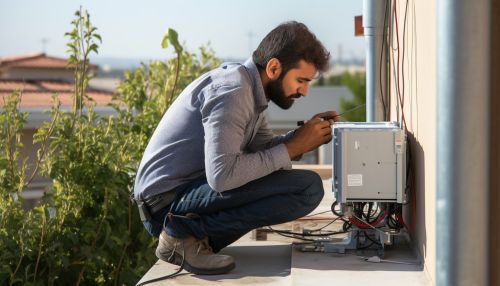
Challenges and Future Directions
Despite its wide applications, the use of geostatistics in air quality prediction also presents several challenges. These include the need for high-quality spatial data, the complexity of geostatistical models, and the uncertainty associated with geostatistical predictions.
Looking ahead, the field of geostatistics is likely to continue evolving with advancements in spatial data collection and analysis techniques. This will further enhance its role in predicting air quality and in informing air quality management strategies.
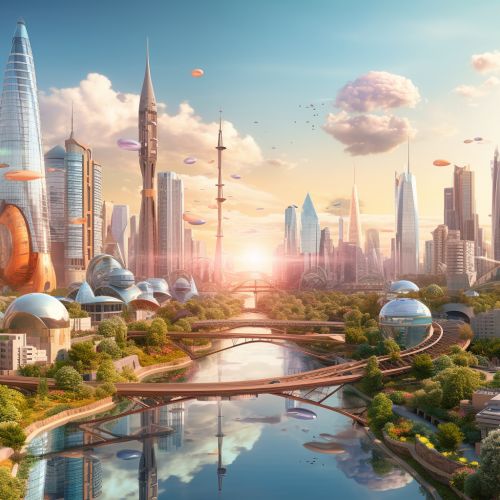
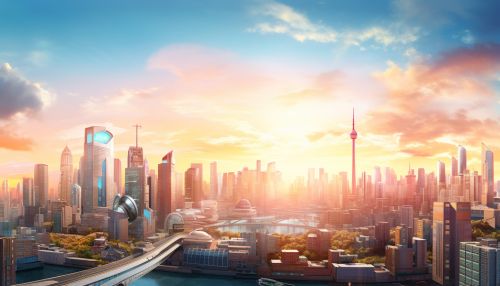