Spatial regression
Introduction
Spatial regression is a statistical technique that is used to model spatial or geographic data. It is a type of regression analysis that is specifically designed to deal with spatial dependence and spatial heterogeneity, two common issues in analyzing spatial data. Spatial regression methods are widely used in fields such as geography, regional science, real estate economics, and epidemiology.
Spatial Dependence
Spatial dependence is a fundamental concept in spatial regression analysis. It refers to the phenomenon where the value of a variable at a certain location is dependent on the values of the variable at other locations. This is often due to the inherent spatial structure of the data, where nearby locations are more likely to have similar values than distant locations. This violates the assumption of independence in traditional regression analysis, leading to biased and inefficient estimates.
Spatial Heterogeneity
Another important concept in spatial regression analysis is spatial heterogeneity. This refers to the variation in the relationships between variables across space. In other words, the relationship between the dependent variable and the independent variables may not be the same in all locations. This also violates the assumption of homogeneity in traditional regression analysis, leading to biased and inefficient estimates.
Types of Spatial Regression Models
There are several types of spatial regression models, each designed to handle different types of spatial dependence and spatial heterogeneity.
Spatial Lag Model
The spatial lag model is a type of spatial regression model that incorporates spatial dependence in the dependent variable. In this model, the value of the dependent variable at a certain location is influenced not only by the values of the independent variables at that location, but also by the values of the dependent variable at neighboring locations.
Spatial Error Model
The spatial error model is another type of spatial regression model that incorporates spatial dependence, but in the error term. In this model, the error term at a certain location is influenced by the error terms at neighboring locations.
Geographically Weighted Regression
Geographically weighted regression (GWR) is a type of spatial regression model that incorporates spatial heterogeneity. In GWR, the relationship between the dependent variable and the independent variables is allowed to vary across space, allowing for local variations in the relationships.
Estimation of Spatial Regression Models
The estimation of spatial regression models is typically more complex than the estimation of traditional regression models. This is due to the presence of spatial dependence and spatial heterogeneity, which require special estimation methods.
Maximum Likelihood Estimation
Maximum likelihood estimation (MLE) is a common method for estimating the parameters of spatial regression models. MLE is a statistical method that finds the parameter values that maximize the likelihood of the observed data given the model.
Generalized Method of Moments
The generalized method of moments (GMM) is another method for estimating the parameters of spatial regression models. GMM is a statistical method that uses moment conditions to estimate the parameters.
Applications of Spatial Regression
Spatial regression methods are widely used in various fields to model spatial data. Some examples of applications include:
- In geography, spatial regression is used to model spatial patterns in variables such as climate, vegetation, and land use. - In regional science, spatial regression is used to model regional economic variables such as income, employment, and housing prices. - In real estate economics, spatial regression is used to model housing prices based on spatial characteristics such as location and neighborhood quality. - In epidemiology, spatial regression is used to model the spatial distribution of diseases and health outcomes.
See Also
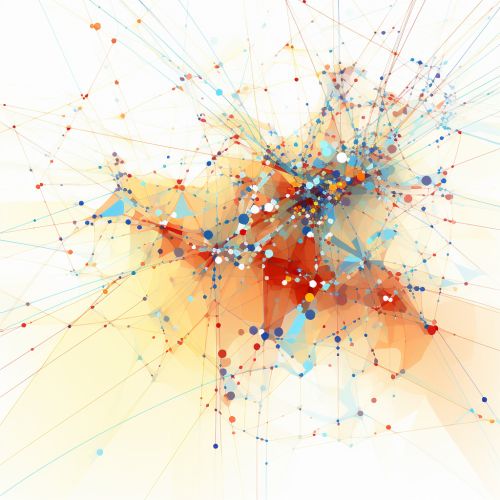
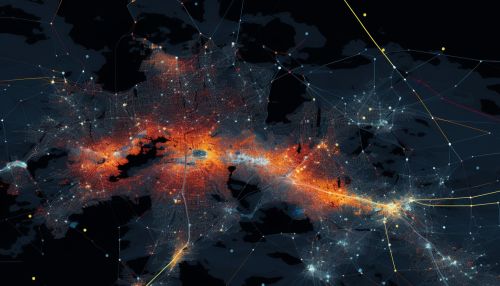