Spatial Analysis in Urban Planning
Introduction
Spatial analysis in urban planning involves the study of geographic phenomena in terms of their locations, relationships, trends, and patterns. In urban planning, spatial analysis is used to manage growth and development, to understand the urban environment, and to assess the impact of urban plans on the community and the environment. It is a broad field that encompasses many different techniques and methods, including GIS, remote sensing, and statistical analysis.
Spatial Analysis Techniques
Spatial analysis techniques in urban planning can be broadly divided into three categories: descriptive, predictive, and prescriptive.
Descriptive Techniques
Descriptive techniques aim to summarize and visualize spatial data. They include mapping, spatial autocorrelation analysis, and spatial interpolation. Mapping is the most basic form of spatial analysis and involves the creation of maps to represent geographic data. Spatial autocorrelation analysis is used to measure the degree to which a set of spatial features and their associated values tend to be clustered together in space. Spatial interpolation is a method used to estimate values of a variable at unobserved locations based on observed values at nearby locations.
Predictive Techniques
Predictive techniques aim to model spatial patterns or predict spatial phenomena. They include spatial regression analysis, spatial interaction models, and geographically weighted regression. Spatial regression analysis is used to model the relationships between a dependent variable and one or more independent variables, taking into account the spatial autocorrelation. Spatial interaction models are used to predict the flow of people, goods, or information between locations in a geographic space. Geographically weighted regression is a local form of spatial regression analysis that allows the relationships between the dependent and independent variables to vary over space.
Prescriptive Techniques
Prescriptive techniques aim to optimize spatial decisions or solve spatial problems. They include location-allocation models, spatial optimization models, and multi-criteria decision analysis. Location-allocation models are used to determine the best locations for facilities such as schools, hospitals, and fire stations, given the locations of the demand points and the capacity of the facilities. Spatial optimization models are used to find the optimal solution to a spatial problem, such as the shortest path between two locations or the maximum coverage of a service area. Multi-criteria decision analysis is used to evaluate and rank alternative spatial decisions based on multiple criteria.
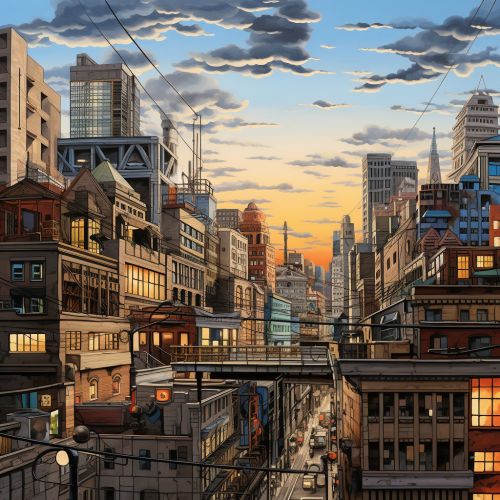
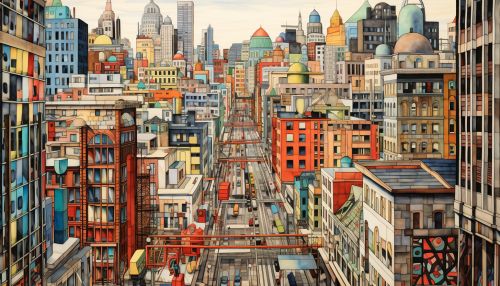
Applications of Spatial Analysis in Urban Planning
Spatial analysis is used in many aspects of urban planning, including land use planning, transportation planning, environmental planning, and social planning.
Land Use Planning
In land use planning, spatial analysis is used to assess the suitability of land for various uses, to analyze the impacts of land use changes, and to develop land use plans. For example, a GIS-based land suitability analysis can be used to identify the most suitable locations for residential, commercial, industrial, and agricultural uses based on a set of criteria such as soil type, slope, proximity to roads, and proximity to services. A spatial impact analysis can be used to assess the impacts of a proposed land use change on the environment, the community, and the economy.
Transportation Planning
In transportation planning, spatial analysis is used to analyze transportation networks, to model transportation demand, and to plan transportation infrastructure. For example, a network analysis can be used to analyze the connectivity, accessibility, and efficiency of a transportation network. A spatial interaction model can be used to predict the demand for transportation between different locations based on the population, employment, and travel costs. A location-allocation model can be used to plan the locations of transportation facilities such as bus stops, train stations, and airports.
Environmental Planning
In environmental planning, spatial analysis is used to assess environmental conditions, to analyze environmental impacts, and to plan environmental conservation. For example, a remote sensing-based land cover analysis can be used to assess the condition of natural resources such as forests, wetlands, and wildlife habitats. A spatial impact analysis can be used to analyze the impacts of urban development on the environment, such as air pollution, water pollution, and habitat loss. A spatial optimization model can be used to plan the conservation of biodiversity hotspots and the restoration of degraded ecosystems.
Social Planning
In social planning, spatial analysis is used to analyze social patterns, to model social phenomena, and to plan social services. For example, a spatial autocorrelation analysis can be used to analyze the spatial patterns of social phenomena such as crime, poverty, and health. A spatial regression analysis can be used to model the relationships between social phenomena and their spatial determinants, such as the relationship between crime rates and neighborhood characteristics. A location-allocation model can be used to plan the locations of social services such as schools, hospitals, and social housing.
Challenges and Future Directions
While spatial analysis has become an essential tool in urban planning, it also faces several challenges. These include the complexity of urban systems, the uncertainty of spatial data, the limitations of spatial analysis techniques, and the ethical issues related to spatial analysis.
The complexity of urban systems poses a challenge to spatial analysis because it requires the integration of multiple types of data and the use of complex models. The uncertainty of spatial data, such as the accuracy, precision, and reliability of the data, can affect the results of spatial analysis. The limitations of spatial analysis techniques, such as the assumptions, simplifications, and approximations of the techniques, can also affect the results.
The ethical issues related to spatial analysis include the privacy and confidentiality of spatial data, the equity and justice of spatial decisions, and the participation and empowerment of communities in spatial analysis. These issues require careful consideration and management in the practice of spatial analysis.
The future directions of spatial analysis in urban planning include the development of new techniques and methods, the integration of spatial analysis with other disciplines, and the application of spatial analysis in new areas. The development of new techniques and methods, such as spatial big data analysis, spatial machine learning, and spatial artificial intelligence, can enhance the capabilities of spatial analysis. The integration of spatial analysis with other disciplines, such as urban design, urban sociology, and urban ecology, can broaden the scope of spatial analysis. The application of spatial analysis in new areas, such as smart cities, sustainable cities, and resilient cities, can expand the relevance of spatial analysis.