Statistical Analysis
Introduction
Statistical analysis is a component of data analysis that involves the collection, organization, interpretation, presentation, and modeling of data. It is used in various fields, including economics, business, engineering, and sciences, to make informed decisions based on data.
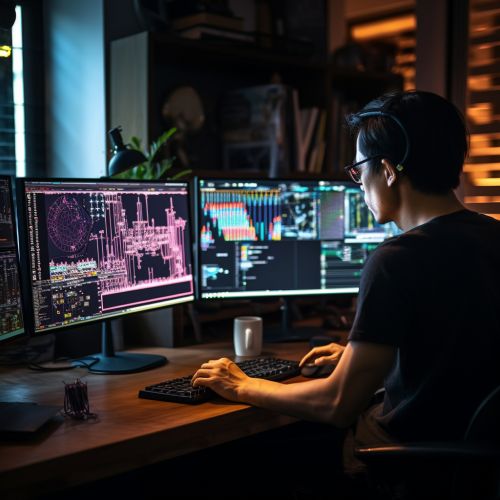
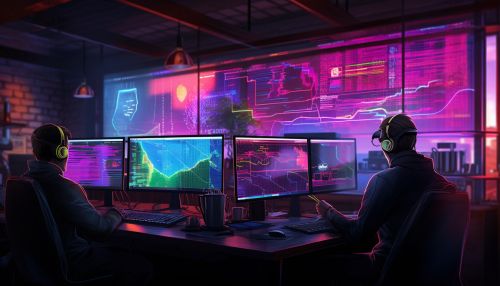
Types of Statistical Analysis
There are two main types of statistical analysis: descriptive statistics and inferential statistics.
Descriptive Statistics
Descriptive statistics summarize and organize characteristics of a data set. A data set could represent a population or a sample of the population. Descriptive statistics are broken down into measures of central tendency and measures of variability (or spread).
Measures of Central Tendency
Measures of central tendency include the mean, median, and mode. The mean is the average of a set of numbers, calculated by adding all numbers in the data set and then dividing by the number of values in the set. The median is the middle value when a data set is ordered from least to greatest. The mode is the value that appears most frequently in a data set.
Measures of Variability
Measures of variability include the range, interquartile range (IQR), variance, and standard deviation. The range is the difference between the highest and lowest values in a data set. The IQR is a measure of statistical dispersion, being equal to the difference between the upper and lower quartiles. Variance is the expectation of the squared deviation of a random variable from its mean. The standard deviation is a measure of the amount of variation or dispersion of a set of values.
Inferential Statistics
Inferential statistics make predictions or inferences about a population based on a sample of data taken from the population in question. Inferential statistics are valuable when examination of each member of an entire population is not convenient or possible.
Hypothesis Testing
hypothesis testing is a statistical method that is used in making statistical decisions using experimental data. It is basically an assumption that we make about the population parameter.
Statistical Significance
Statistical significance is the likelihood that the difference in conversion rates between a given variation and the baseline is not due to random chance. A result of an experiment is said to have statistical significance, or be statistically significant, if it is likely not caused by chance for a given statistical significance level.
Confidence Intervals
A confidence interval gives an estimated range of values which is likely to include an unknown population parameter, the estimated range being calculated from a given set of sample data.
Applications of Statistical Analysis
Statistical analysis has widespread applications in fields as diverse as economics, medicine, and engineering. It is used to understand and interpret phenomena in any area that involves data collection and interpretation.
Economics
In economics, statistical analysis is used to understand and describe phenomena such as inflation, economic growth, and unemployment. Economists use statistical methods to collect and analyze data, and to test hypotheses about economic behavior and policy.
Medicine
In medicine, statistical analysis is used in clinical trials to assess the efficacy of new treatments. It is also used in epidemiology to understand the spread and control of diseases.
Engineering
In engineering, statistical analysis is used in quality control and process control. It is also used in reliability engineering to predict and prevent failures.
Conclusion
Statistical analysis is a powerful tool that helps us understand and interpret the world around us. It is used in a wide range of fields and can help us make informed decisions based on data.