Semivariogram Property
Introduction
The Semivariogram is a fundamental tool in spatial statistics, specifically in the field of geostatistics. It is a function describing the degree of spatial dependence of a spatial random field or stochastic process. The semivariogram is defined for a pair of locations as half the average squared difference of the variable values at these locations.
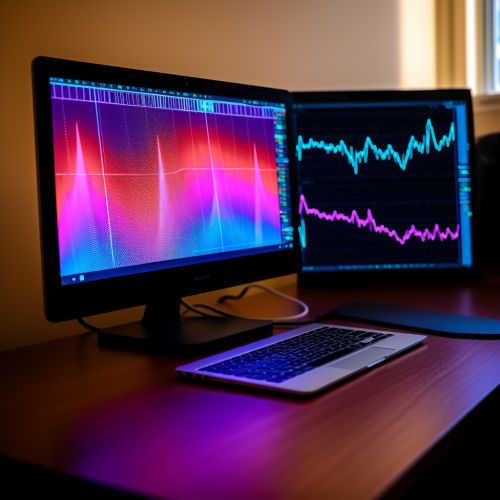
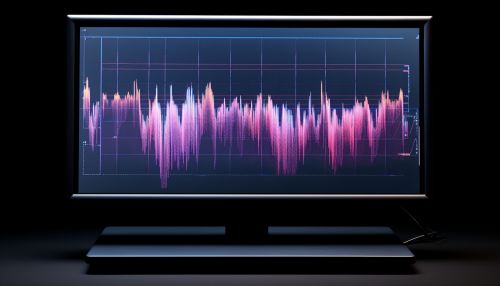
Definition
The semivariogram, denoted as γ(h), for a spatial random field Z(x), where x is a location in a d-dimensional space, and h is a vector lag, is defined as:
γ(h) = 0.5 * E{[Z(x + h) - Z(x)]²}
where E denotes the expectation operator. This definition is based on the second-order stationarity assumption, which assumes that the mean and variance are constant over space, and that the covariance depends only on the distance and direction between locations, not on their absolute positions.
Properties
The semivariogram has several important properties that are used in spatial analysis and modeling. These properties are derived from the definition and the assumptions of the spatial random field.
Positive Definiteness
The semivariogram is a positive definite function. This means that for any set of locations {x1, x2, ..., xn} and any set of real numbers {a1, a2, ..., an}, the following inequality holds:
∑i ∑j ai * aj * γ(xi - xj) ≥ 0
This property ensures that the semivariogram matrix used in kriging, a geostatistical estimation technique, is always invertible.
Symmetry
The semivariogram is a symmetric function. This means that the semivariogram value for a lag h is the same as for the lag -h:
γ(h) = γ(-h)
This property reflects the assumption of isotropy, which means that the spatial dependence does not depend on the direction of the lag vector.
Continuity
The semivariogram is a continuous function at the origin. This means that as the lag h approaches zero, the semivariogram value approaches zero:
lim h→0 γ(h) = 0
This property reflects the assumption of intrinsic stationarity, which means that the variance of the difference between values at two locations depends only on the distance between them, not on their absolute positions.
Boundedness
The semivariogram is a bounded function. This means that there is a finite value, called the sill, beyond which the semivariogram does not increase:
lim h→∞ γ(h) = C
where C is the sill. This property reflects the assumption of stationarity, which means that the spatial dependence does not increase indefinitely with distance.
Estimation
The semivariogram is typically estimated from data using the method of moments. The empirical semivariogram, denoted as γ*(h), is defined as:
γ*(h) = 1/(2N(h)) * ∑i=1 to N(h) [Z(xi + h) - Z(xi)]²
where N(h) is the number of pairs of locations separated by the lag h. The empirical semivariogram is a biased estimator of the true semivariogram, but it is consistent, which means that as the sample size increases, the estimate converges to the true value.
Modeling
The semivariogram is often modeled using a parametric function, such as the exponential, spherical, or Gaussian function. The choice of the model depends on the characteristics of the data and the assumptions of the spatial random field. The parameters of the model, including the range, sill, and nugget, are typically estimated using the method of least squares or maximum likelihood.
Applications
The semivariogram is used in various applications in geostatistics, including spatial prediction, spatial interpolation, and spatial simulation. The most common application is kriging, a geostatistical estimation technique that uses the semivariogram to quantify the spatial dependence and to estimate values at unsampled locations.