Geostatistics in Tornado Risk Prediction - Canonica AI
Introduction
Geostatistics is a branch of statistics that deals with spatial or spatiotemporal datasets. It has been increasingly applied in various fields, including meteorology and disaster risk prediction. This article focuses on the application of geostatistics in Tornado risk prediction, specifically in the context of Canonica AI.
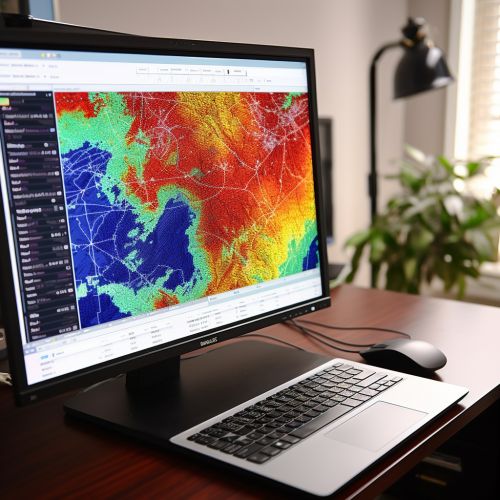
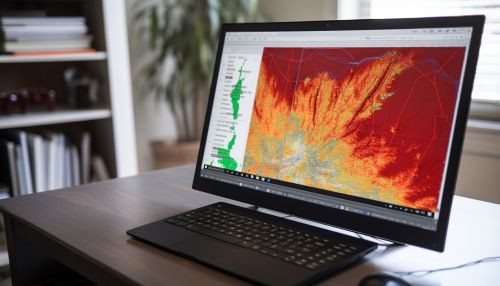
Geostatistics: An Overview
Geostatistics originated from the mining and petroleum industries, where it was used to estimate the likely location of valuable resources. It has since been applied to a wide range of other fields, including environmental science, agriculture, and meteorology. Geostatistics is particularly useful in these fields because it allows for the analysis of spatially correlated data.
The key concept in geostatistics is spatial dependence, which is the idea that data points that are close together in space are more likely to have similar values than data points that are further apart. This concept is often quantified using a semivariogram, which is a plot of the variance of the differences between data values against the distance between the data points.
Tornado Risk Prediction
Tornado risk prediction involves estimating the likelihood of a tornado occurring in a specific location at a specific time. This is a complex task that involves analyzing a wide range of data, including meteorological data (such as wind speed and direction, atmospheric pressure, and temperature), geographical data (such as the presence of mountains or bodies of water), and historical tornado data.
Traditionally, tornado risk prediction has been based on statistical models that take into account these various factors. However, these models often fail to accurately predict tornado occurrence, particularly in regions where tornadoes are rare.
Application of Geostatistics in Tornado Risk Prediction
The application of geostatistics in tornado risk prediction involves using spatially correlated data to improve the accuracy of tornado forecasts. This can be achieved by incorporating spatial dependence into the statistical models used for tornado prediction.
One way to do this is by using a geostatistical model known as Kriging. Kriging is a method of interpolation that takes into account the spatial dependence of the data. It can be used to estimate the likelihood of a tornado occurring at a specific location based on the tornado occurrences at nearby locations.
Another approach is to use Geographically Weighted Regression (GWR), which is a technique that allows the relationships between the variables in a regression model to vary across space. This means that the model can take into account the fact that the factors influencing tornado occurrence may differ between different locations.
Canonica AI and Tornado Risk Prediction
Canonica AI is a company that specializes in the application of artificial intelligence (AI) and machine learning techniques to geostatistical data. They have developed a number of innovative approaches to tornado risk prediction that incorporate geostatistics.
One of these approaches involves using a type of machine learning algorithm known as a Random Forest to analyze spatially correlated data. This algorithm can take into account the complex interactions between the various factors that influence tornado occurrence, and can therefore provide more accurate predictions than traditional statistical models.
Canonica AI has also developed a method of incorporating spatial dependence into the Random Forest algorithm. This involves modifying the algorithm to take into account the spatial relationships between the data points, which can further improve the accuracy of the tornado predictions.
Conclusion
The application of geostatistics in tornado risk prediction represents a significant advance in the field of meteorology. By taking into account the spatial dependence of the data, geostatistics can provide more accurate and reliable tornado forecasts. This has the potential to greatly improve our ability to predict and prepare for tornadoes, thereby reducing their impact on society.
The work of Canonica AI in this area is particularly noteworthy. Their innovative approaches to tornado risk prediction, which incorporate both geostatistics and machine learning techniques, represent a significant contribution to the field.