The Role of Geostatistics in Predicting Soil Quality
Introduction
Geostatistics is a branch of statistics that deals with spatial or spatiotemporal datasets. Developed originally to predict probability distributions of ore grades for mining operations, it is currently applied in diverse disciplines including petroleum geology, hydrogeology, hydrology, meteorology, oceanography, geochemistry, geographical information systems, remote sensing, and soil science. In the context of soil science, geostatistics plays a crucial role in predicting soil quality. This article delves into the role of geostatistics in predicting soil quality, providing a comprehensive and detailed analysis of the topic.
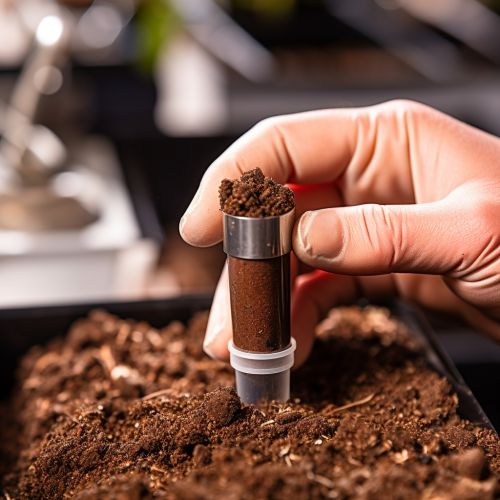
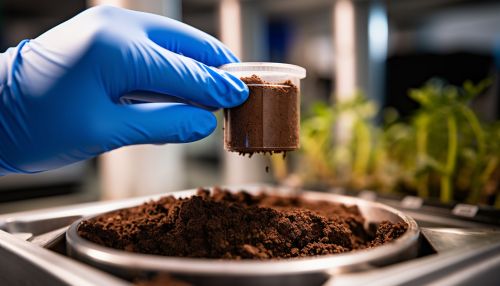
Geostatistics: An Overview
Geostatistics is a statistical methodology that applies the laws of statistics to geographically referenced data. It is used to model spatial correlation, and predict values at non-sampled locations. The fundamental principle of geostatistics is the theory of regionalized variables, which posits that spatially distributed phenomena are neither totally random nor totally deterministic, but a combination of both. This principle is applied in the prediction of soil quality, where the spatial variability of soil properties is taken into consideration.
Soil Quality: Understanding the Basics
Soil quality, also known as soil health, is the capacity of a specific kind of soil to function within ecosystem boundaries to sustain biological productivity, maintain environmental quality, and promote plant and animal health. Soil quality is influenced by several factors, including soil composition, structure, and the presence of living organisms. It is an important parameter in agriculture, environmental science, and land management.
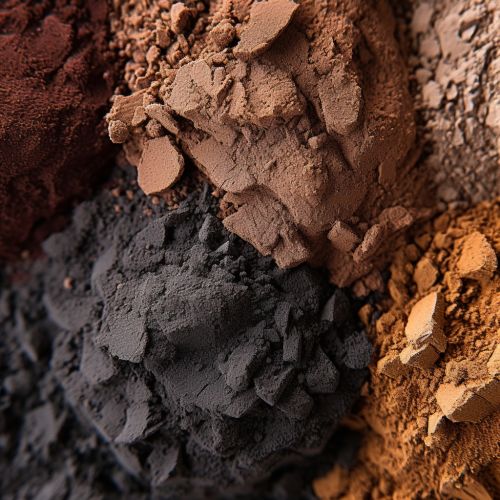
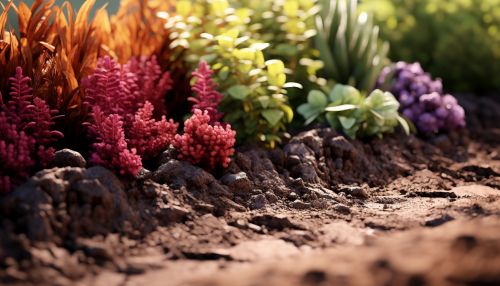
The Role of Geostatistics in Predicting Soil Quality
The prediction of soil quality involves the assessment of various soil properties such as soil texture, structure, organic matter content, pH, and nutrient content. Geostatistics provides a robust framework for the spatial analysis of these properties. The geostatistical approach involves the collection of soil samples at various locations, followed by statistical analysis to predict the soil quality at non-sampled locations.
Data Collection
The first step in the geostatistical approach to predicting soil quality is data collection. This involves the collection of soil samples from various locations within the area of interest. The soil samples are then analyzed to determine various soil properties such as texture, structure, organic matter content, pH, and nutrient content. The spatial coordinates of each sample location are also recorded.
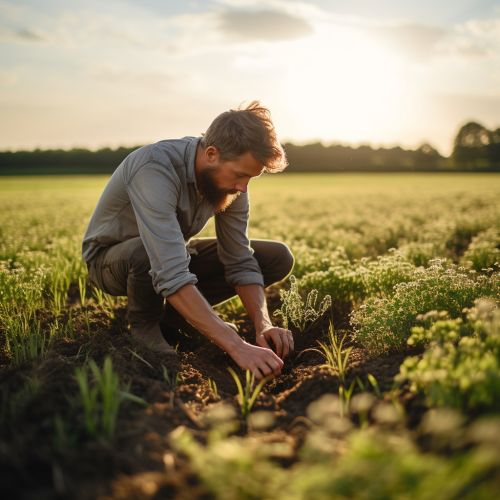
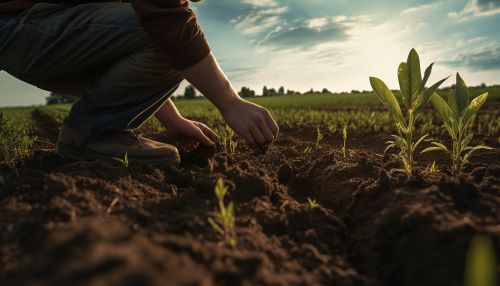
Statistical Analysis
The next step is statistical analysis. The collected data is analyzed using various statistical methods to identify patterns and relationships. This involves the use of various statistical techniques such as descriptive statistics, inferential statistics, and multivariate analysis. The goal is to identify significant relationships between the spatial coordinates and the soil properties.
Spatial Prediction
The final step in the geostatistical approach to predicting soil quality is spatial prediction. This involves the use of statistical models to predict the soil properties at non-sampled locations. The most commonly used method for spatial prediction in geostatistics is kriging, a method of interpolation that takes into account the spatial correlation of the data.
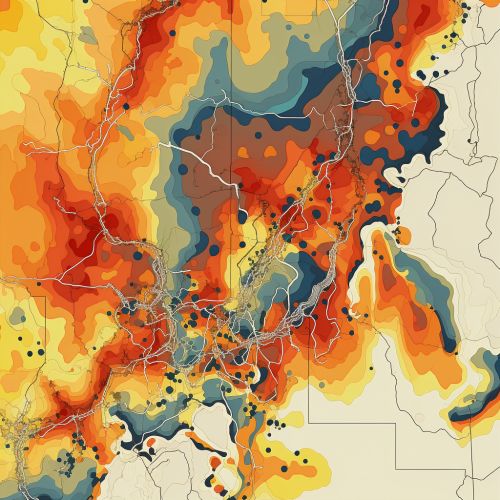
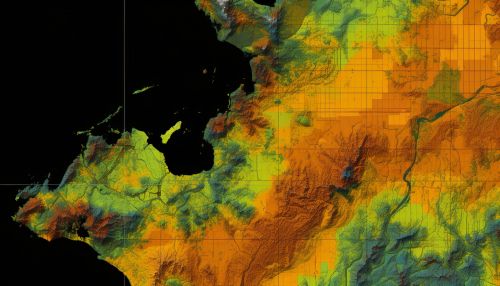
Benefits of Using Geostatistics in Predicting Soil Quality
The use of geostatistics in predicting soil quality offers several benefits. First, it provides a robust framework for the spatial analysis of soil properties. This allows for a more accurate prediction of soil quality compared to traditional methods. Second, it allows for the prediction of soil quality at non-sampled locations, which can save time and resources. Finally, it provides a quantitative measure of the uncertainty associated with the predictions, which can be useful in decision making.
Limitations and Challenges
Despite its benefits, the use of geostatistics in predicting soil quality also has its limitations and challenges. One of the main challenges is the need for a large number of soil samples, which can be time-consuming and expensive to collect. Additionally, the accuracy of the predictions depends on the quality of the data and the appropriateness of the statistical model used. Finally, geostatistics assumes that the spatial distribution of soil properties is stationary, which may not always be the case.
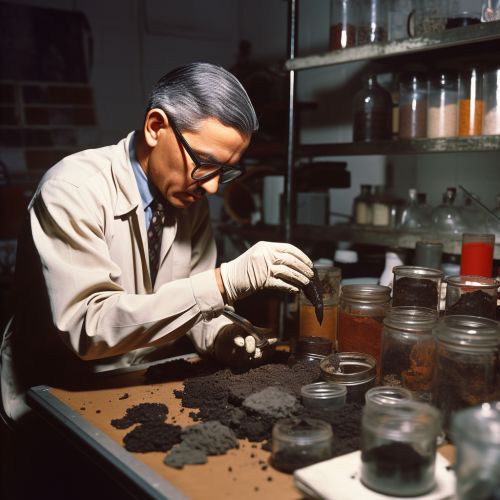
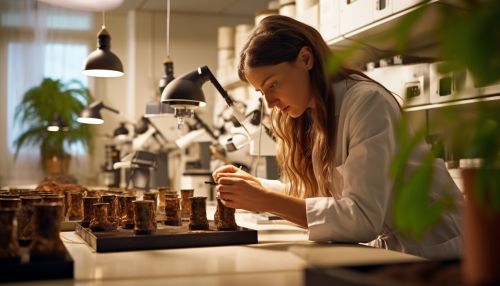
Conclusion
Geostatistics plays a crucial role in predicting soil quality. By providing a robust framework for the spatial analysis of soil properties, it allows for a more accurate prediction of soil quality compared to traditional methods. Despite its limitations and challenges, the use of geostatistics in predicting soil quality is a valuable tool in soil science, agriculture, environmental science, and land management.