The Role of Geostatistics in Predicting Rainfall Patterns
Introduction
Geostatistics is a branch of statistics focusing on spatial or spatiotemporal datasets. Developed originally to predict probability distributions of ore grades for mining operations, it is currently applied in diverse disciplines including petroleum geology, hydrogeology, hydrology, meteorology, oceanography, geochemistry, geometallurgy, geography, forestry, environmental control, landscape ecology, soil science, and agriculture (crop yield estimation). Among these fields, the role of geostatistics in predicting rainfall patterns is particularly significant. This article explores the principles, methodologies, and applications of geostatistics in rainfall prediction.
Geostatistics: An Overview
Geostatistics is a statistical methodology that deals with the analysis, interpretation, and prediction of spatial phenomena. It involves the application of statistical methods to geographically referenced data to predict values at unobserved locations. Geostatistics is based on the concept of spatial correlation, which assumes that data points located close to each other are more likely to have similar values than those located farther apart. This concept is encapsulated in the principle of spatial autocorrelation, which is a key component of geostatistical analysis.
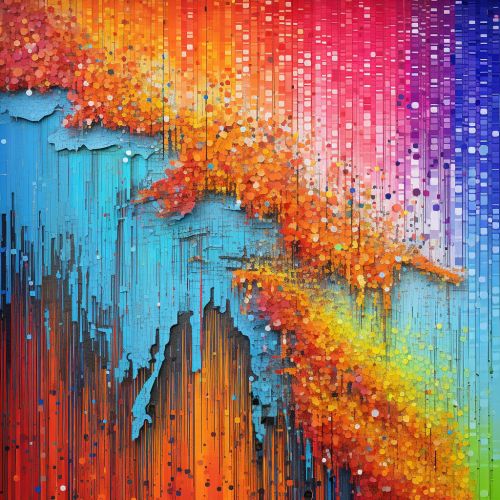
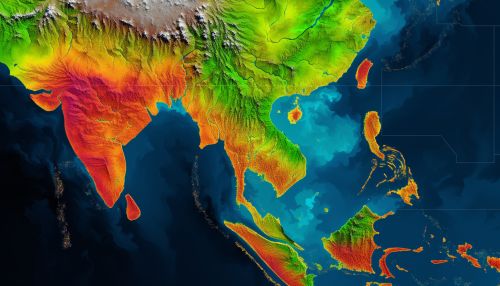
Principles of Geostatistics
Geostatistics operates on several key principles. First, it acknowledges the spatial dependence of geospatial data, recognizing that measurements taken at nearby locations are not independent. This principle is often referred to as the First Law of Geography, which states that "everything is related to everything else, but near things are more related than distant things". This principle is quantified in a measure known as the semivariogram, which is a function of the distance and direction separating two locations.
Second, geostatistics employs a method known as kriging, named after the South African mining engineer Danie G. Krige. Kriging is a linear, unbiased statistical method that provides a best linear unbiased prediction (BLUP) of missing values in a spatial dataset. Kriging uses the semivariogram to determine the spatial dependence and applies this information to predict values at unobserved locations.
Geostatistics in Rainfall Prediction
Rainfall prediction is a critical aspect of weather forecasting and climate modeling. Accurate rainfall prediction can help mitigate the impacts of severe weather events, assist in water resource management, and contribute to agricultural planning. Geostatistics plays a vital role in rainfall prediction by providing a statistical framework for analyzing and interpreting spatially referenced rainfall data.
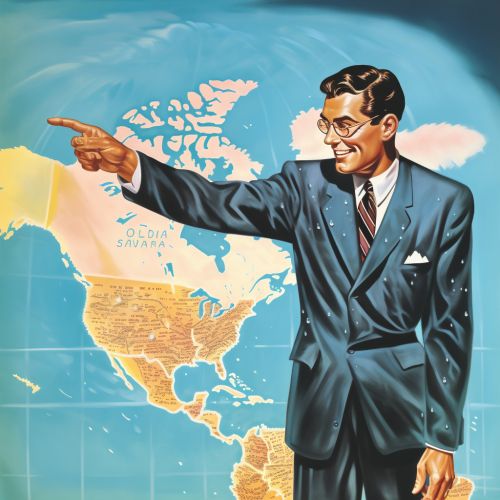
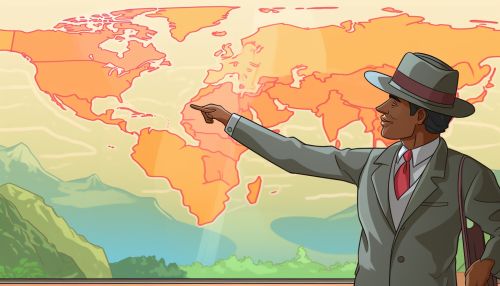
Data Collection
The first step in geostatistical rainfall prediction is data collection. Rainfall data is typically collected from a network of rain gauges, which are instruments used to measure the amount of rain that falls over a specific period. The spatial distribution of rain gauges can greatly influence the accuracy of rainfall prediction. Therefore, the design of the rain gauge network is a crucial aspect of rainfall data collection.
Spatial Interpolation
Once the rainfall data is collected, it is subjected to spatial interpolation, a process that estimates rainfall values at unobserved locations based on observed data. Kriging is the most commonly used spatial interpolation method in geostatistics. It uses the spatial correlation structure of the data, as represented by the semivariogram, to predict rainfall values at unobserved locations.
Uncertainty Quantification
Geostatistics also provides a means to quantify the uncertainty associated with rainfall predictions. This is achieved through a process known as stochastic simulation, which generates multiple equally probable realizations of the rainfall field. These realizations can be used to estimate the uncertainty of the predicted rainfall values.
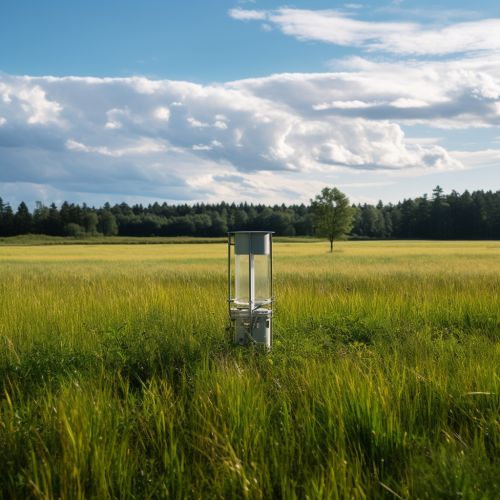
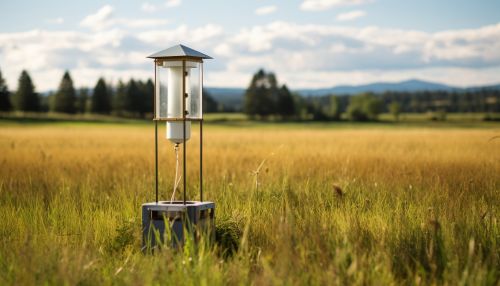
Applications of Geostatistics in Rainfall Prediction
Geostatistical methods have been widely applied in rainfall prediction in various contexts. In weather forecasting, geostatistics is used to interpolate rainfall data from weather radar and rain gauge networks to produce high-resolution rainfall maps. In climate modeling, geostatistics is used to downscale coarse-resolution climate model outputs to finer scales that are more relevant for local impact assessments.
In hydrology, geostatistics is used to estimate spatial rainfall patterns for input into hydrological models, which are used to predict river flows and flood risks. In agriculture, geostatistical rainfall predictions are used to inform irrigation scheduling and crop yield estimation.
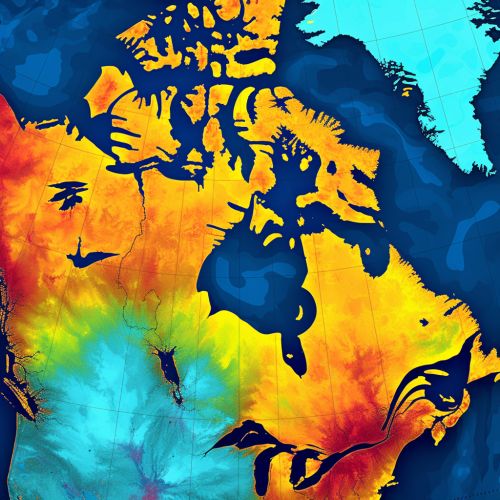
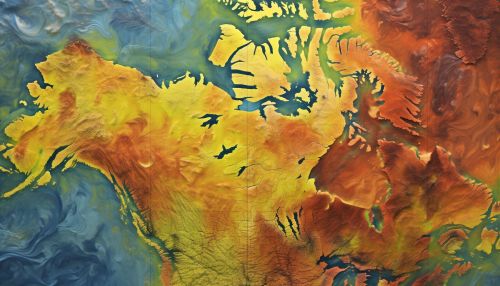
Conclusion
The role of geostatistics in predicting rainfall patterns is significant and wide-ranging. By providing a robust statistical framework for analyzing and interpreting spatially referenced rainfall data, geostatistics enables more accurate and reliable rainfall predictions. These predictions are crucial for a variety of applications, from weather forecasting and climate modeling to hydrology and agriculture.