Statistical Models
Introduction
Statistical models are a key component in the field of statistics and data analysis. They provide a mathematical representation of the stochastic processes that generate data. Statistical models are used to understand the underlying structure of the data, make predictions, and test hypotheses.
Types of Statistical Models
There are several types of statistical models, each with its own set of assumptions and applications.
Parametric Models
Parametric models assume that the data follows a certain distribution. The parameters of this distribution, such as the mean and variance, are estimated from the data. Examples of parametric models include the normal distribution, exponential distribution, and Poisson distribution.
Non-Parametric Models
Non-parametric models make fewer assumptions about the data's distribution. They are more flexible than parametric models and can be used when the distribution of the data is unknown. Examples of non-parametric models include the kernel density estimator and the K-nearest neighbors algorithm.
Semi-Parametric Models
Semi-parametric models are a compromise between parametric and non-parametric models. They make some assumptions about the data's distribution, but these assumptions are less restrictive than those made by parametric models. Examples of semi-parametric models include the Cox proportional hazards model and the generalized additive model.
Bayesian Models
Bayesian models incorporate prior knowledge about the parameters into the model. The parameters are treated as random variables, and their distribution is updated as new data is observed. Examples of Bayesian models include the Bayesian linear regression model and the Bayesian network.
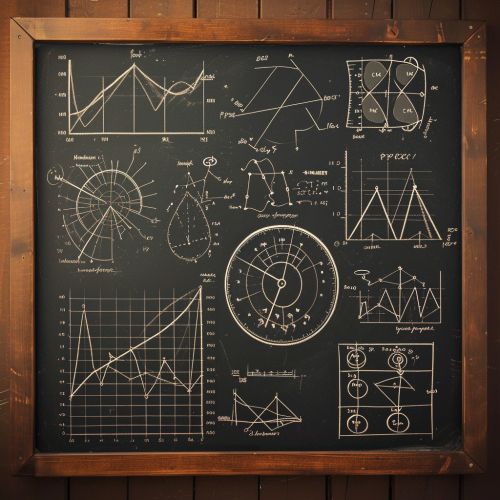
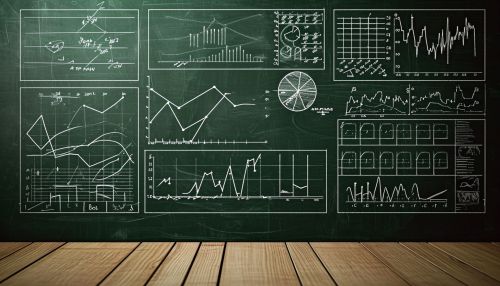
Model Selection
Model selection is an important step in the statistical modeling process. It involves choosing the model that best fits the data and meets the objectives of the analysis. Criteria for model selection include goodness of fit, simplicity, and predictive accuracy.
Goodness of Fit
Goodness of fit refers to how well the model describes the data. It is usually measured by a statistic that compares the observed data to the data predicted by the model. Common goodness of fit measures include the likelihood ratio, the Akaike information criterion, and the Bayesian information criterion.
Simplicity
Simplicity refers to the complexity of the model. A simpler model is preferable if it fits the data almost as well as a more complex model. This principle is known as Occam's razor.
Predictive Accuracy
Predictive accuracy refers to how well the model predicts new data. It is usually measured by a statistic that compares the observed outcomes to the outcomes predicted by the model. Common measures of predictive accuracy include the mean squared error and the area under the receiver operating characteristic curve.
Applications of Statistical Models
Statistical models are used in a wide range of fields, including economics, sociology, psychology, biology, and computer science. They are used to analyze data, make predictions, and test hypotheses.
Conclusion
Statistical models are a fundamental tool in statistics and data analysis. They provide a mathematical representation of the stochastic processes that generate data, and they are used to understand the underlying structure of the data, make predictions, and test hypotheses. The choice of model depends on the nature of the data and the objectives of the analysis.