Semi-Parametric Models
Introduction
Semi-parametric models are a type of statistical model that includes both parametric and non-parametric components. These models are used in various fields such as economics, biostatistics, and machine learning. They provide a flexible approach to data analysis, allowing for the modeling of complex relationships without making strong assumptions about the functional form of the data.
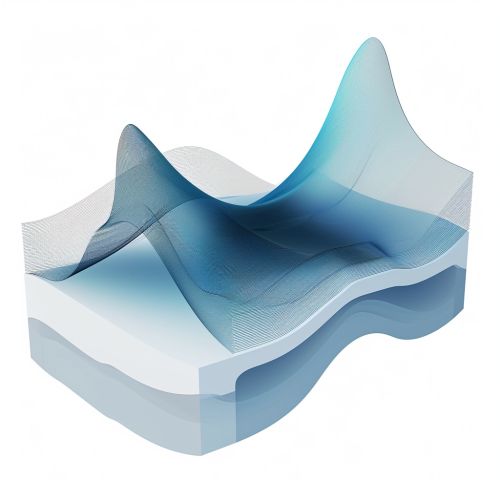
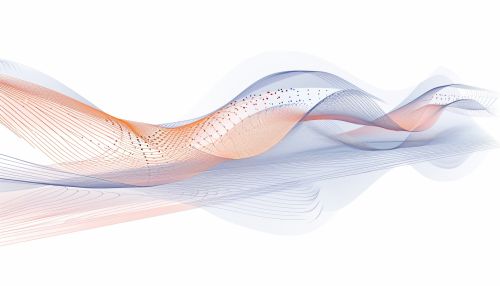
Parametric and Non-parametric Models
Before delving into semi-parametric models, it is important to understand the concepts of parametric and non-parametric models. Parametric models assume that the data follows a specific distribution, such as the normal distribution. They are defined by a finite set of parameters. Non-parametric models, on the other hand, do not make any assumptions about the data's distribution. They are defined by an infinite set of parameters, providing more flexibility but also increasing the complexity of the model.
Semi-parametric Models
Semi-parametric models combine the best of both worlds. They include a parametric component, which provides a simple and interpretable model for the data, and a non-parametric component, which allows for flexibility and the ability to model complex relationships. This combination allows semi-parametric models to provide a more accurate representation of the data than either parametric or non-parametric models alone.
Types of Semi-parametric Models
There are various types of semi-parametric models, including but not limited to:
Generalized Additive Models
Generalized additive models (GAMs) are a type of semi-parametric model that allows for non-linear relationships between the predictor variables and the response variable. They are an extension of generalized linear models (GLMs), where the linear predictor is replaced by a sum of smooth functions.
Cox Proportional Hazards Model
The Cox proportional hazards model is a semi-parametric model used in survival analysis. It assumes that the hazard rate is a function of the covariates and a baseline hazard that can take any form.
Partially Linear Models
Partially linear models are a type of semi-parametric model where the relationship between the predictor variables and the response variable is partially linear and partially non-linear.
Advantages and Disadvantages
Like any statistical model, semi-parametric models have their advantages and disadvantages.
Advantages
The main advantage of semi-parametric models is their flexibility. They can model complex relationships without making strong assumptions about the functional form of the data. They also provide a balance between bias and variance, leading to more accurate predictions.
Disadvantages
On the downside, semi-parametric models can be more complex and computationally intensive than parametric models. They also require more data to estimate the non-parametric component accurately.
Applications
Semi-parametric models are used in various fields, including economics, biostatistics, and machine learning. They are particularly useful when the relationship between the predictor variables and the response variable is complex and cannot be accurately modeled using a simple parametric model.
Conclusion
Semi-parametric models provide a flexible and powerful tool for data analysis. By combining the simplicity and interpretability of parametric models with the flexibility of non-parametric models, they allow for the modeling of complex relationships without making strong assumptions about the functional form of the data.