Hypothesis Testing
Introduction
Hypothesis testing is a fundamental concept in statistics that allows researchers to make inferences or predictions about a population based on a sample of data. It is a method of making decisions using data, whether from a controlled experiment or an observational study.
Concept and Definition
In hypothesis testing, two statements about a population are compared: the null hypothesis (denoted by H0) and the alternative hypothesis (denoted by H1 or Ha). The null hypothesis is a statement of no effect or no difference and is assumed true until evidence indicates otherwise. The alternative hypothesis is a statement of an effect or difference.
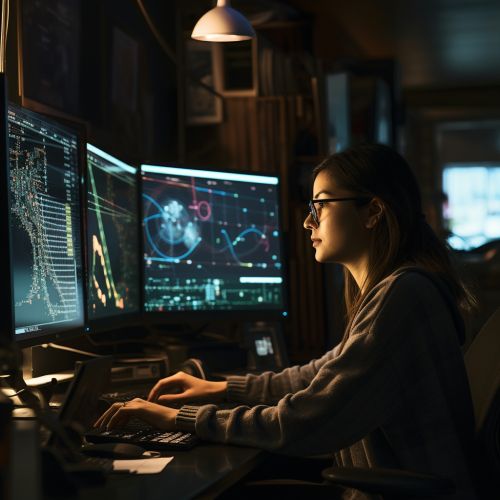
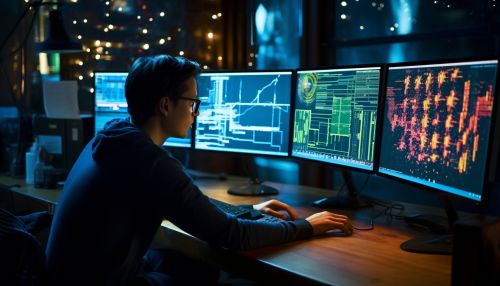
Steps in Hypothesis Testing
Hypothesis testing involves several steps:
- Formulate the null hypothesis (H0) and the alternative hypothesis (H1).
- Choose the significance level (α), the probability of rejecting the null hypothesis when it is true. The most commonly used α is 0.05.
- Determine the appropriate statistical test.
- Compute the test statistic.
- Make a decision: if the test statistic falls in the critical region, reject the null hypothesis.
Types of Errors in Hypothesis Testing
In hypothesis testing, there are two types of errors that can occur:
- Type I error (α): This occurs when the null hypothesis is true, but is rejected. It is the level of significance of the test.
- Type II error (β): This occurs when the null hypothesis is false, but is not rejected.
Types of Hypothesis Tests
There are several types of hypothesis tests, including:
- Z-tests: Used when the population standard deviation is known and the sample size is large.
- T-tests: Used when the population standard deviation is unknown and the sample size is small.
- Chi-square tests: Used to test the association between two categorical variables.
- Analysis of variance (ANOVA): Used to compare the means of more than two groups.
Conclusion
Hypothesis testing is a critical tool in statistics for determining whether a result is statistically significant. It allows researchers to make inferences about a population based on a sample of data. By understanding the process and the potential errors, one can make more informed decisions based on the results of a hypothesis test.