Inferential Statistics
Introduction
Inferential statistics is a branch of statistics that involves drawing conclusions about a population based on a sample. This form of statistics is used to make inferences or predictions about a larger group based on data collected from a smaller group, or sample. Inferential statistics can be contrasted with descriptive statistics, which focuses on summarizing the observed data.
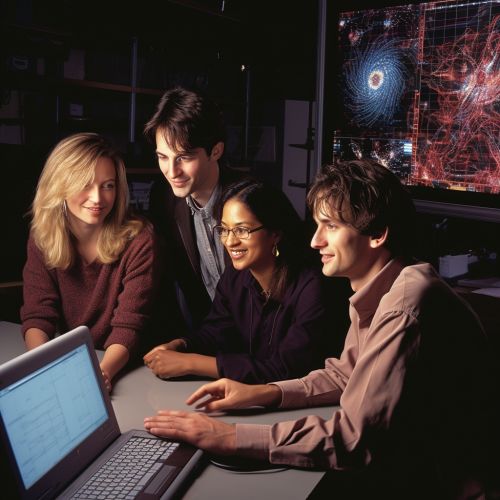
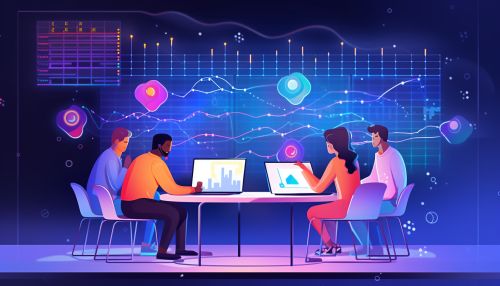
Principles of Inferential Statistics
The primary goal of inferential statistics is to make inferences about populations based on samples. This involves using statistical tests to determine whether a hypothesis about the population is supported by the sample data. The two main types of statistical inference are estimation and hypothesis testing.
Estimation
Estimation involves using sample data to estimate a population parameter. There are two types of estimation in inferential statistics: point estimation and interval estimation.
Point estimation involves using sample data to calculate a single value which is to serve as a 'best guess' or 'best estimate' of an unknown (fixed or random) population parameter. For example, the sample mean is a point estimate of the population mean.
Interval estimation involves using sample data to calculate an interval of possible values of an unknown population parameter. This is often characterized by the confidence level, which is the probability that the interval estimate will contain the parameter. For example, a 95% confidence interval for a population mean is an interval estimate that, in repeated sampling, would contain the population mean 95% of the time.
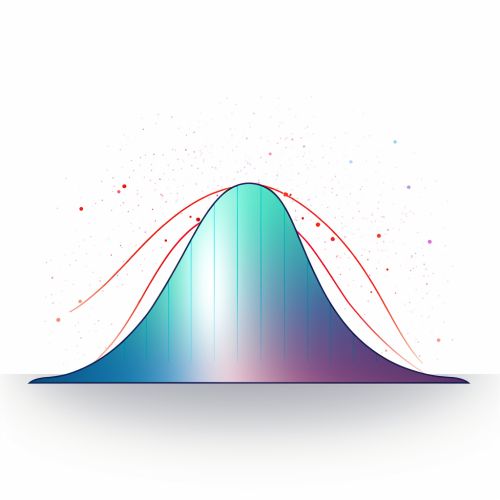
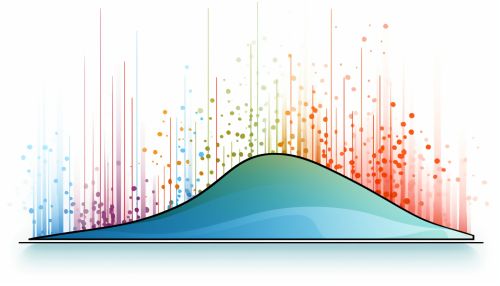
Hypothesis Testing
Hypothesis testing is a formal procedure for comparing observed data with a claim (also called a hypothesis) about the population. The goal of hypothesis testing is to make a decision about the validity of the claim.
The first step in hypothesis testing is to specify the null hypothesis and the alternative hypothesis. The null hypothesis is a statement about the population that will be accepted if the sample data do not provide sufficient evidence that it is false. The alternative hypothesis is a statement that will be accepted only if the sample data provide convincing evidence of its truth.
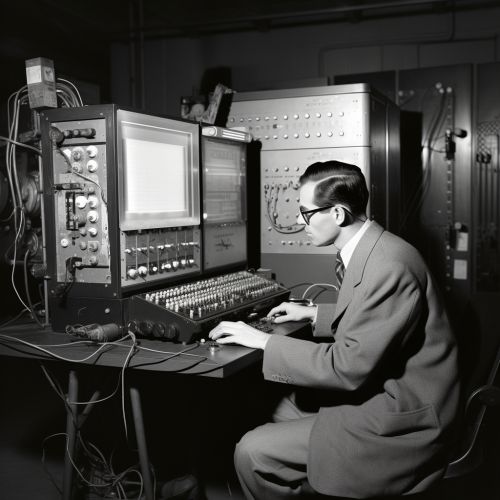
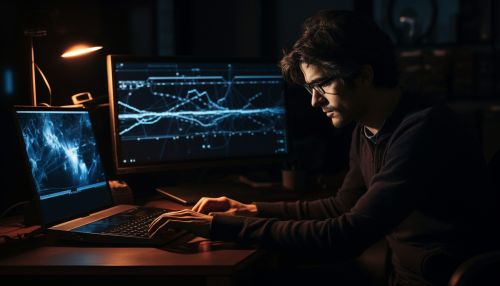
Methods of Inferential Statistics
There are numerous methods used in inferential statistics. These methods can be broadly categorized into parametric and non-parametric methods.
Parametric Methods
Parametric methods in inferential statistics are methods that assume the data follow a certain distribution. The most common distribution assumed is the normal distribution, but other distributions such as the binomial distribution or the Poisson distribution can also be assumed.
Parametric methods include t-tests, analysis of variance (ANOVA), regression analysis, and more. These methods are often more powerful than non-parametric methods, meaning they are more likely to detect a significant effect if one exists.
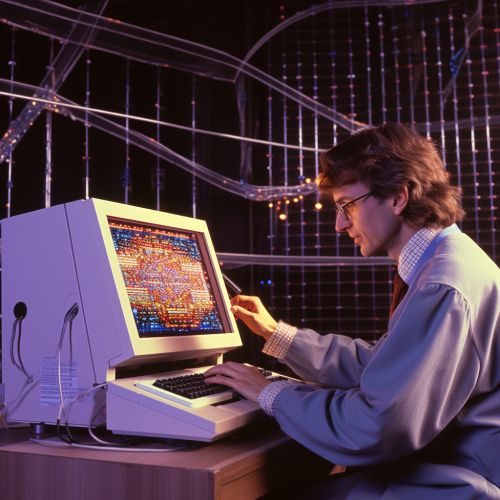
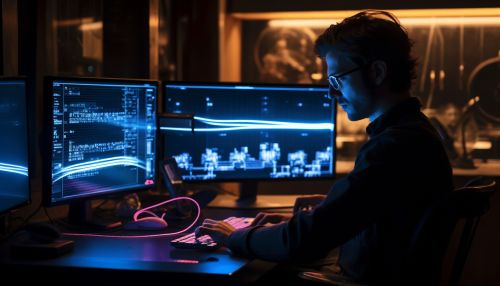
Non-Parametric Methods
Non-parametric methods in inferential statistics are methods that do not assume the data follow a certain distribution. These methods are often used when the data are not normally distributed and cannot be transformed to a normal distribution.
Non-parametric methods include the Wilcoxon signed-rank test, the Mann-Whitney U test, the Kruskal-Wallis test, and more. These methods are often less powerful than parametric methods, but they are more robust, meaning they are less sensitive to violations of assumptions.
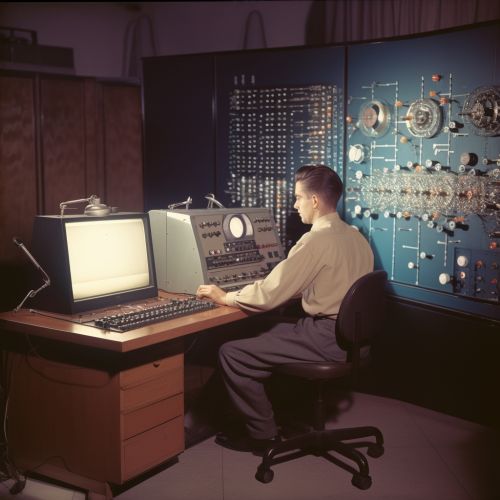
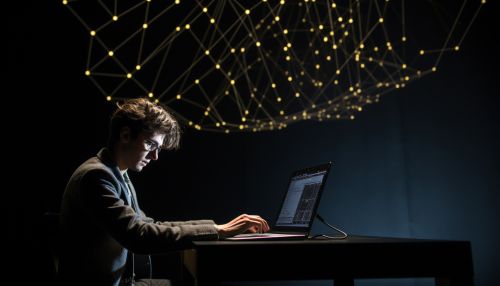
Applications of Inferential Statistics
Inferential statistics has a wide range of applications in various fields. It is used in fields such as psychology, medicine, economics, and more to make inferences about populations based on sample data.
In psychology, inferential statistics might be used to test hypotheses about the effects of a treatment on a sample of patients, and then make inferences about the effects of the treatment on the population of all patients.
In medicine, inferential statistics might be used to estimate the effectiveness of a new drug based on a clinical trial, and then make inferences about the effectiveness of the drug in the population.
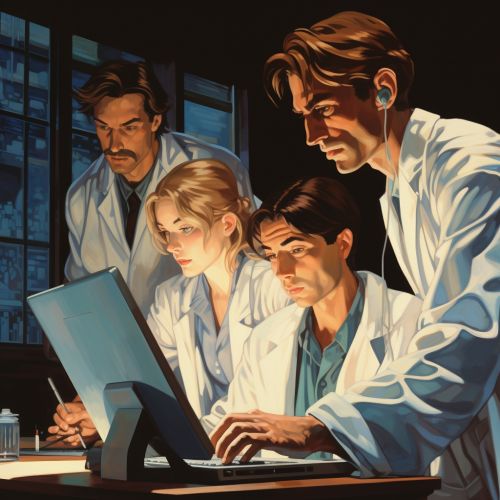
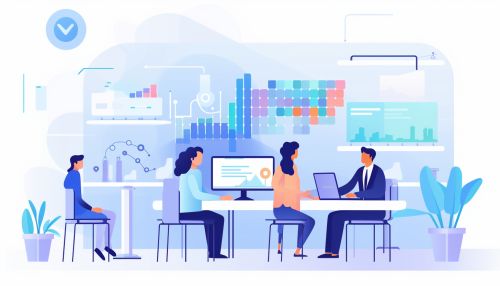
Limitations of Inferential Statistics
While inferential statistics is a powerful tool, it is not without its limitations. One of the main limitations is that it relies on the quality of the sample data. If the sample is not representative of the population, the inferences made may not be valid.
Another limitation is that inferential statistics can only provide probabilities, not certainties. For example, a 95% confidence interval does not mean that there is a 95% chance that the population parameter is within the interval. Rather, it means that if we were to take many samples and calculate a confidence interval for each one, about 95% of these intervals would contain the population parameter.
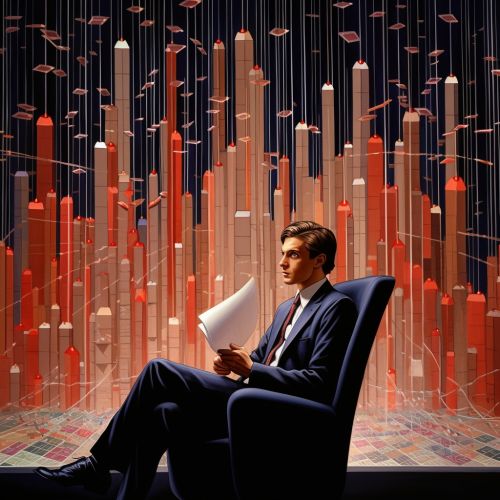
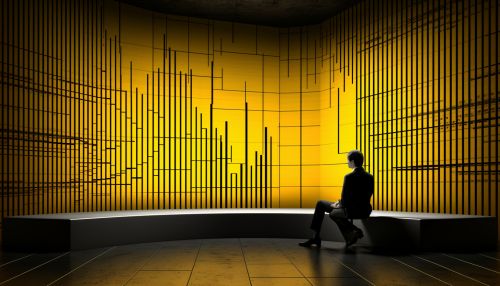
Conclusion
Inferential statistics is a key component of statistical analysis, allowing researchers to make inferences about populations based on sample data. Despite its limitations, it is a powerful tool that is widely used in a variety of fields.