Sequential Gaussian simulation
Introduction
Sequential Gaussian simulation (SGS) is a geostatistical technique used in the field of geostatistics for generating multiple, equally probable, realizations of a spatially correlated variable. The method is based on the principle of spatial correlation, where the value of a variable at a particular location is influenced by the values at nearby locations. This method is widely used in various fields such as hydrogeology, petroleum geology, and environmental science.
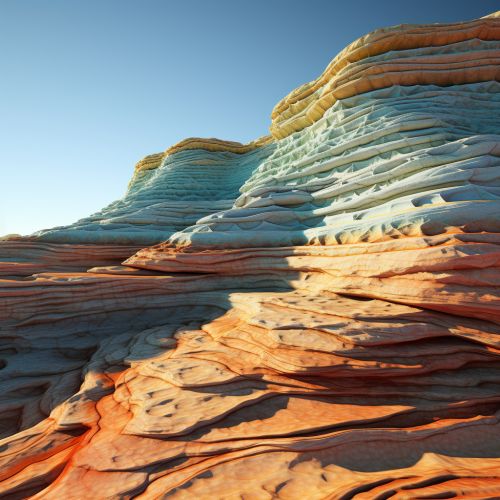
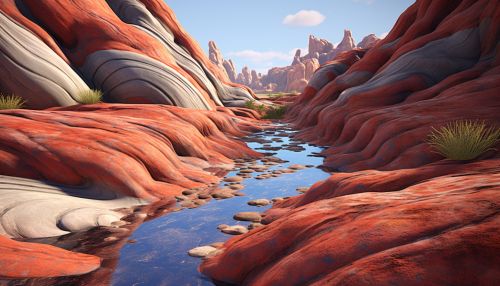
Methodology
The Sequential Gaussian Simulation method involves several steps, including data normalization, variogram modeling, sequential simulation, and back-transformation. The process begins with the collection of sample data from the field, which is then normalized to follow a Gaussian distribution. This is followed by the modeling of the spatial correlation structure using a variogram, which quantifies the degree of spatial dependence between data points.
The actual simulation process involves visiting each unsampled location in a random order and estimating the value at that location based on the values at the already simulated or sampled locations. This is done using a conditional cumulative distribution function (CCDF), which is constructed using the variogram model and the values at the nearby locations. A value is then drawn from this CCDF and assigned to the location. This process is repeated until all locations have been visited and assigned a value.
The final step in the process is the back-transformation of the simulated values to their original scale. This is necessary because the simulation was carried out on the normalized data, which may not reflect the actual range of values in the field.
Applications
Sequential Gaussian Simulation has a wide range of applications in various fields. In hydrogeology, it is used for modeling the spatial distribution of hydraulic properties such as permeability and porosity, which are crucial for understanding groundwater flow and contaminant transport. In petroleum geology, SGS is used for modeling the distribution of reservoir properties such as porosity, permeability, and oil saturation. This information is vital for reservoir characterization and production forecasting.
In environmental science, SGS is used for mapping the spatial distribution of pollutants in soil or groundwater. This helps in identifying hotspots of contamination and planning remediation strategies. SGS is also used in the field of meteorology for spatial interpolation of weather variables such as temperature and precipitation.
Advantages and Limitations
One of the main advantages of Sequential Gaussian Simulation is that it provides multiple realizations of the spatial variable, each honoring the sample data and the spatial correlation structure. This allows for a better understanding of the uncertainty associated with the spatial prediction. Another advantage is that SGS can handle non-stationary data, i.e., data with a spatial correlation structure that changes over space.
However, SGS also has some limitations. The method assumes that the data follows a Gaussian distribution, which may not always be the case. Also, SGS can be computationally intensive, especially for large datasets. Furthermore, the quality of the simulation results heavily depends on the quality of the input data and the variogram model.