Estimation Theory
Introduction
Estimation theory is a branch of statistics that deals with estimating the values of parameters based on measured empirical data that has a random component. The parameters describe an underlying physical or social phenomenon. In performing estimation, it is customary to make a probability distribution assumption for the observations.
Background
The field of estimation theory was developed in the context of mathematics, statistics, and probability theory. It has found important applications in numerous fields, including physics, engineering, and economics. The goal of estimation theory is to approximate the values of parameters in a statistical model, which are not directly observable, based on observed data.
Types of Estimation
There are two types of estimation in statistics: point estimation and interval estimation.
Point Estimation
In point estimation, the goal is to provide a single best prediction of some quantity of interest. The most common method of point estimation is the method of maximum likelihood. This method involves specifying a likelihood function and then finding the parameter values that maximize this likelihood function.
Interval Estimation
Interval estimation is the use of sample data to calculate an interval of possible (or probable) values of an unknown population parameter. The most common method of interval estimation is the construction of confidence intervals.
Estimators
An estimator is a rule for calculating an estimate of a given quantity based on observed data. In estimation theory, two types of estimators are commonly used: unbiased estimators and consistent estimators.
Unbiased Estimators
An unbiased estimator is an estimator whose expected value is equal to the true value of the parameter being estimated. Unbiased estimators are desirable because they provide accurate estimates of the parameters.
Consistent Estimators
A consistent estimator is an estimator that, as the sample size increases, converges in probability to the true value of the parameter being estimated. Consistency of an estimator is a key property that ensures that the estimates improve as more data is collected.
Methods of Estimation
There are several methods of estimation used in estimation theory. These include the method of moments, maximum likelihood estimation, and Bayesian estimation.
Method of Moments
The method of moments involves equating the sample moments to the population moments and solving for the parameters.
Maximum Likelihood Estimation
Maximum likelihood estimation is a method that determines the parameter values that maximize the likelihood function, given the observed data.
Bayesian Estimation
Bayesian estimation is a method that combines prior information about the parameter with the observed data to produce the posterior distribution of the parameter.
Applications
Estimation theory is widely used in various fields such as engineering, physics, economics, and social sciences. In engineering, it is used in signal processing and control systems. In economics, it is used to estimate economic models and in econometrics.
See Also

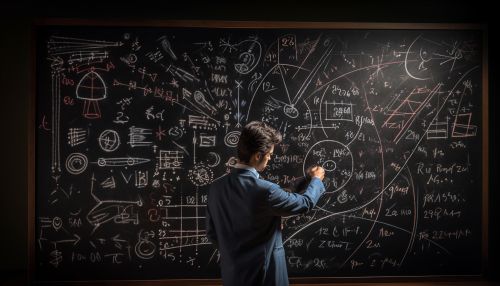