Geostatistical simulation
Introduction
Geostatistical simulation is a discipline within the field of geostatistics that employs mathematical models to generate multiple, equally probable, realizations of spatially distributed phenomena. These simulations are used to assess the uncertainty associated with spatial prediction and to aid in decision-making processes in various fields such as mining, hydrogeology, environmental science, and petroleum engineering.
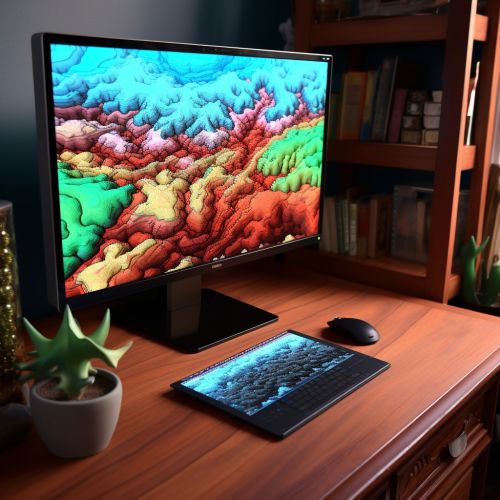
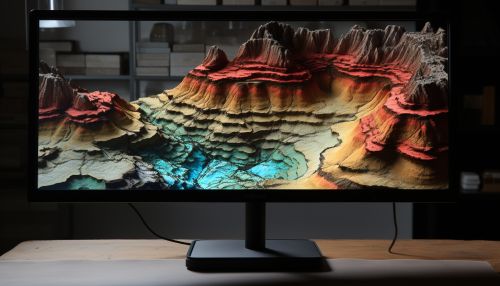
Principles of Geostatistical Simulation
Geostatistical simulation is based on the principles of spatial statistics, which involves the analysis and interpretation of data that are spatially distributed. The key principles include:
Spatial Continuity
Spatial continuity refers to the degree of correlation or dependence between values at different locations in space. This is quantified using a semivariogram, a function that describes the spatial continuity of a regionalized variable.
Random Function Theory
Random function theory is a fundamental principle in geostatistical simulation. It considers the spatially distributed variable as a realization of a random function. This allows for the incorporation of uncertainty in the spatial prediction.
Conditional Simulation
Conditional simulation is a method that generates multiple realizations of a spatially distributed variable, given a set of known data. Each realization honors the data and the spatial continuity as defined by the semivariogram.
Techniques in Geostatistical Simulation
There are several techniques used in geostatistical simulation, each with its own advantages and limitations. The choice of technique depends on the nature of the data, the complexity of the spatial continuity, and the purpose of the simulation.
Sequential Gaussian Simulation
Sequential Gaussian Simulation (SGS) is a widely used technique in geostatistical simulation. It involves transforming the data to a Gaussian distribution, simulating at unsampled locations sequentially, and transforming back to the original distribution.
Sequential Indicator Simulation
Sequential Indicator Simulation (SIS) is used when the variable of interest is categorical. It involves transforming the data into binary indicators, simulating each category sequentially, and combining the results to obtain the final realization.
Multiple-Point Statistics
Multiple-Point Statistics (MPS) is a relatively new technique that uses a training image to define the spatial continuity. It is particularly useful when the spatial continuity is complex and cannot be adequately described by a semivariogram.
Applications of Geostatistical Simulation
Geostatistical simulation is used in various fields to assess the uncertainty associated with spatial prediction and to aid in decision-making processes. Some of the applications include:
Mining
In mining, geostatistical simulation is used to generate multiple realizations of orebody models. These models are used to assess the uncertainty in mineral resources and reserves estimation, and to optimize mine planning and design.
Hydrogeology
In hydrogeology, geostatistical simulation is used to generate multiple realizations of hydrogeological parameters such as hydraulic conductivity and porosity. These realizations are used to assess the uncertainty in groundwater flow and contaminant transport modeling.
Environmental Science
In environmental science, geostatistical simulation is used to generate multiple realizations of environmental variables such as soil properties, air quality indices, and pollutant concentrations. These realizations are used to assess the uncertainty in environmental risk assessment and to design optimal monitoring networks.
Petroleum Engineering
In petroleum engineering, geostatistical simulation is used to generate multiple realizations of reservoir properties such as porosity, permeability, and oil saturation. These realizations are used to assess the uncertainty in reservoir performance prediction and to optimize reservoir development and management.