Deep Learning in Medical Imaging
Introduction
Deep learning, a subset of machine learning, has emerged as a revolutionary tool in the field of medical imaging. It utilizes artificial neural networks with multiple layers (hence the term "deep") to model and understand complex patterns in data. The application of deep learning in medical imaging has shown promising results in improving diagnostic accuracy, predicting treatment response, and personalizing treatment plans.
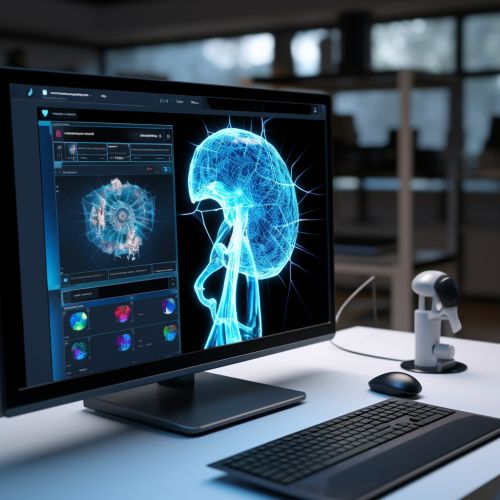
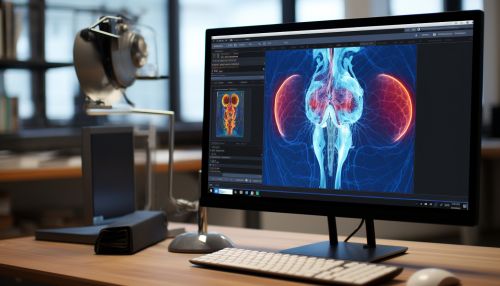
Deep Learning: A Brief Overview
Deep learning is a machine learning technique that teaches computers to do what comes naturally to humans: learn by example. Deep learning is a key technology behind driverless cars, enabling them to recognize a stop sign or to distinguish a pedestrian from a lamppost. It is the key to voice control in consumer devices like phones, tablets, TVs, and hands-free speakers.
Deep learning models are built using neural networks that contain several layers of nodes (or "neurons"), mimicking the neural structure of the human brain. Each layer of nodes processes the input data, passes on the processed information to the next layer, and so on, until the final layer produces the output. This layered architecture allows deep learning models to learn complex patterns and relationships in the input data.
Medical Imaging: An Essential Diagnostic Tool
Medical imaging refers to the techniques and processes used to create images of the human body for clinical purposes or medical science. It is a crucial aspect of modern medicine and plays an essential role in the diagnosis, treatment, and monitoring of diseases. Medical imaging encompasses various techniques such as x-ray radiography, MRI, CT, ultrasound, and PET.
Deep Learning in Medical Imaging
The application of deep learning in medical imaging, also known as radiomics, has shown significant potential in enhancing healthcare delivery. Deep learning algorithms can be trained to recognize patterns and anomalies in medical images, aiding in the detection and diagnosis of diseases. These algorithms can also be used to predict patient outcomes, guide treatment planning, and monitor disease progression.
Disease Detection and Diagnosis
Deep learning algorithms can be trained to detect and diagnose various diseases from medical images. For example, convolutional neural networks (CNNs), a type of deep learning model, have been used to detect lung nodules in CT scans, diagnose breast cancer from mammograms, and identify brain tumors in MRI scans. These algorithms can analyze an image and produce a diagnosis in a matter of seconds, significantly reducing the time taken for manual interpretation.
Treatment Planning
Deep learning can also assist in treatment planning. For instance, in radiation therapy for cancer treatment, deep learning algorithms can be used to segment the tumor and surrounding organs at risk in the medical images. This segmentation is crucial for planning the radiation dose distribution to maximize the dose to the tumor while minimizing the dose to the surrounding healthy tissues.
Disease Monitoring
Deep learning can be used to monitor the progression of diseases. By analyzing a series of medical images taken over time, deep learning algorithms can detect subtle changes that might indicate disease progression or response to treatment. This can provide valuable information to clinicians and help guide treatment decisions.
Challenges and Future Directions
Despite the promising results, the application of deep learning in medical imaging is not without challenges. These include the need for large amounts of annotated training data, the lack of interpretability of deep learning models, and the need for validation and regulatory approval.
Looking ahead, the integration of deep learning with medical imaging holds great promise for improving patient care. Future research directions include the development of more efficient and interpretable deep learning models, the integration of imaging data with other types of medical data, and the application of deep learning in personalized medicine.