Visual Analytics
Overview
Visual Analytics is the science of analytical reasoning facilitated by interactive visual interfaces. It can be viewed as the integration of two disciplines: visualization and data analysis. Visualization provides a high-level, easy-to-understand view of data, while data analysis provides the methods and techniques to extract insights from data. The goal of visual analytics is to provide insights that are otherwise difficult to obtain due to the complexity and volume of the data.
History
The field of visual analytics was formally established in 2005 with the publication of the report "Illuminating the Path: The Research and Development Agenda for Visual Analytics" by the National Visualization and Analytics Center. This report identified the need for new science to combine visualization and analytics to deal with the increasing amounts and complexity of data.
Data Visualization
Data Visualization is a key component of visual analytics. It involves the creation and study of the visual representation of data, meaning "information that has been abstracted in some schematic form, including attributes or variables for the units of information". It is an important tool in the arsenal of a visual analyst as it allows them to see trends and patterns that are not immediately obvious in raw, textual data.
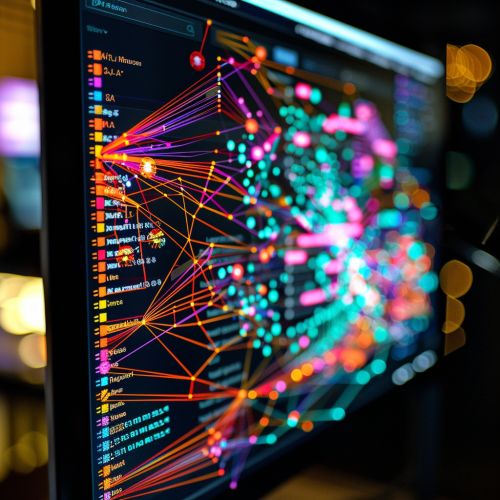
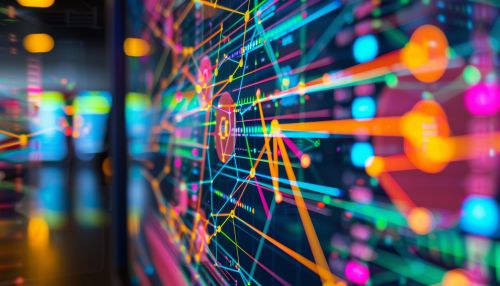
Data Analysis
The other key component of visual analytics is Data Analysis. This involves inspecting, cleaning, transforming, and modeling data with the goal of discovering useful information, informing conclusions, and supporting decision-making. Data analysis has multiple facets and approaches, encompassing diverse techniques under a variety of names, and is used in different business, science, and social science domains.
Techniques
There are many techniques in visual analytics which can be broadly classified into two categories: visualization techniques and analytical techniques. Visualization techniques include Information Visualization, Scientific Visualization, and Geographic Information Systems. Analytical techniques include Data Mining, Machine Learning, and Statistics.
Applications
Visual analytics has a wide range of applications, from business intelligence to social media analysis, from network security to bioinformatics. In each of these areas, visual analytics helps analysts to discover insights, make sense of complex situations, and make informed decisions.
Challenges
Despite its potential, visual analytics faces several challenges. These include dealing with large and complex datasets, integrating diverse data sources, developing effective visual representations, and ensuring the usability of visual analytics tools.
Future Directions
The future of visual analytics lies in addressing these challenges and expanding its applications. Research is ongoing in areas such as interactive visualization, automated analysis, collaborative analytics, and the integration of machine learning with visual analytics.