Univariate Analysis
Introduction
Univariate analysis is the simplest form of quantitative (statistical) analysis. It involves the examination across cases of one variable at a time (univariate). With several variables, each is looked at in isolation. For each variable, a frequency distribution (or histogram), or a summary statistic (like an average), is produced. The analysis of univariate data is thus the simplest form of analysis since the information deals with only one quantity that changes. It does not deal with causes or relationships and the main purpose of the analysis is to describe the data and find patterns that exist within it.
Overview
Univariate analysis is the most basic form of statistical data analysis. When researchers conduct univariate analysis, they are running statistical tests on one variable. This is in contrast to bivariate analysis, which involves two variables, and multivariate analysis, which involves more than two variables. Univariate analysis can yield misleading results in cases where multivariate analysis is more appropriate.
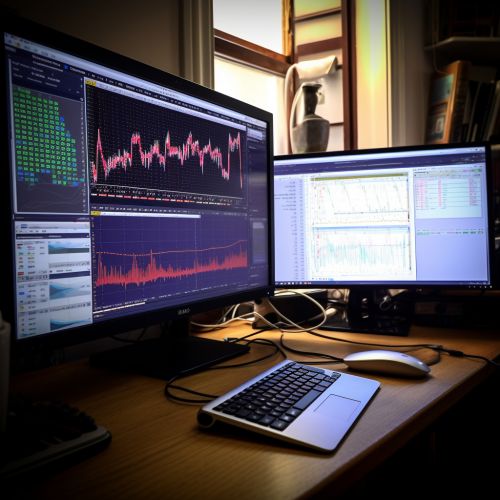
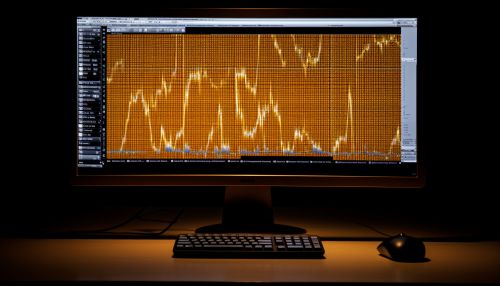
Types of Univariate Analysis
There are two main types of univariate analysis: descriptive and inferential.
Descriptive Univariate Analysis
Descriptive univariate analysis is the simplest form of analysis you can perform on data. In this type of analysis, you are describing, or summarizing your data. This is done through statistics such as measures of central tendency (mean, median, and mode) and measures of dispersion (range, variance, standard deviation, quartiles, and interquartile range).
Inferential Univariate Analysis
Inferential univariate analysis is a statistical method that is used to test hypotheses. The goal of inferential statistics is to draw conclusions from a sample and generalize them to a population. Examples of inferential univariate analysis include the one-sample z-test, one-sample t-test, binomial test, and chi-square goodness of fit test.
Applications of Univariate Analysis
Univariate analysis is used in a variety of fields, including psychology, business, and social sciences. It is often used in exploratory data analysis to highlight the main features of a large and complex dataset. Despite its simplicity, univariate analysis can often yield deep insights into a subject area.
Advantages and Disadvantages of Univariate Analysis
Like any statistical method, univariate analysis has its advantages and disadvantages.
Advantages
Univariate analysis is simple to perform and interpret, making it accessible to researchers of all backgrounds. It is also computationally efficient, which can be a major advantage with large datasets.
Disadvantages
The main disadvantage of univariate analysis is that it does not take into account the interactions between variables. This can lead to misleading results if there are strong correlations between variables in your data.
Conclusion
Univariate analysis is a powerful tool for summarizing and understanding data. However, like all statistical methods, it should be used with caution. Researchers should be aware of the limitations of univariate analysis and consider using more complex methods when appropriate.