Bivariate Analysis
Introduction
Bivariate analysis is a statistical method that helps to understand the relationship between two different variables. It involves the analysis of two variables (often denoted as X, Y), for the purpose of determining the empirical relationship between them[1]. This type of analysis is simpler compared to multivariate analysis, which deals with the study of more than two variables.
Overview
Bivariate analysis is one of the simplest forms of quantitative (statistical) analysis. It involves the analysis of two variables for the purpose of determining the empirical relationship between them. This analysis is used to find out if there is a relationship between two sets of values. It usually involves the variables X and Y.
Types of Bivariate Analysis
There are three main types of bivariate analysis:
- Correlation Analysis: This is used to measure the strength of association between two variables.
- Regression Analysis: This is used to predict the value of one variable based on the value of another variable.
- Contingency Table Analysis: This is used to study the distribution of categorical variables.
Correlation Analysis
Correlation analysis is a method of bivariate analysis used to measure the strength of association between two variables. The correlation coefficient (often denoted by r) is a measure that determines the degree to which two variables' movements are associated. The correlation coefficient ranges from -1 to +1. A value of +1 implies that a linear equation describes the relationship perfectly with all data points lying on a line for which Y increases as X increases. A value of -1 implies that all data points lie on a line for which Y decreases as X increases. A value of 0 implies that there is no linear correlation between the variables.
Regression Analysis
Regression analysis is a form of predictive modelling technique which investigates the relationship between a dependent (target) and independent variable (s) (predictor). This technique is used for forecasting, time series modelling and finding the causal effect relationship between the variables. For example, relationship between rash driving and number of road accidents by a driver is best studied through regression.
Contingency Table Analysis
A contingency table is a type of table in a matrix format that displays the (multivariate) frequency distribution of the variables. They provide a basic picture of the interrelation between two variables and can help find interactions between them. The simplest form of contingency table is a 2x2 contingency table, but it can be of any dimension.
Applications of Bivariate Analysis
Bivariate analysis is widely used in the field of statistics, marketing, finance, research, and in many other fields. It helps to identify and understand the relationships between different variables. Some of the key applications of bivariate analysis include:
- In marketing research, bivariate analysis is used to identify the factors that influence consumer behavior.
- In finance, it is used to understand the relationship between different economic indicators.
- In medical research, bivariate analysis is used to identify the factors that increase the risk of certain diseases.
- In social science research, it is used to understand the relationship between different social indicators.
Limitations of Bivariate Analysis
While bivariate analysis can provide valuable insights, it also has its limitations. Some of the key limitations include:
- Bivariate analysis can only analyze the relationship between two variables at a time. It cannot be used to analyze the relationship between more than two variables.
- Bivariate analysis does not imply causation. Even if there is a strong relationship between two variables, it does not mean that one variable causes the other.
- Bivariate analysis is sensitive to outliers. An outlier can significantly affect the result of the analysis.
Conclusion
Bivariate analysis is a powerful tool in statistical analysis. It provides valuable insights into the relationship between two variables. However, it is important to be aware of its limitations and use it in conjunction with other statistical methods to get a comprehensive understanding of the data.
See Also
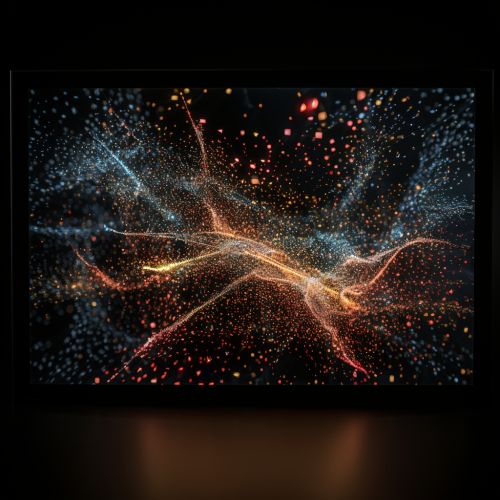
