The Role of Geostatistics in Predicting Deforestation
Introduction
Geostatistics is a branch of statistics focusing on spatial or spatiotemporal datasets. Developed originally to predict probability distributions of ore grades for mining operations, it is currently applied in diverse disciplines including petroleum geology, hydrology, meteorology, and environmental science. Among these fields, the role of geostatistics in predicting deforestation has emerged as a critical area of study, given the increasing global concern over environmental sustainability and climate change.
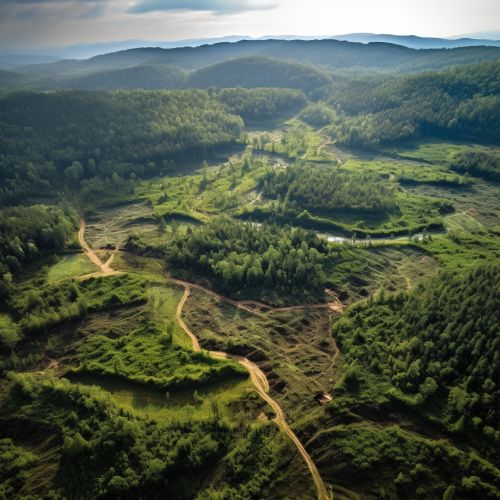
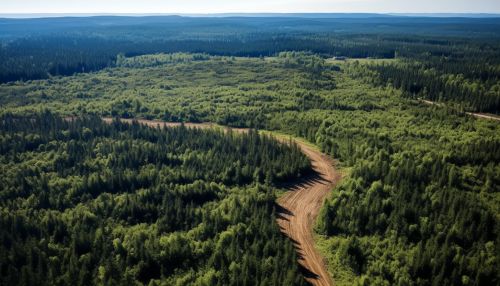
Geostatistics: An Overview
Geostatistics is predicated on the concept of spatial correlation and the principle that things that are close together are more alike than those that are further apart, a concept known as spatial autocorrelation. This principle is encapsulated in Tobler's First Law of Geography, which states that "everything is related to everything else, but near things are more related than distant things."
Geostatistics employs various statistical techniques to analyze spatial or spatiotemporal phenomena, including variograms, kriging, and spatial regression. These techniques are used to estimate values at unsampled locations, based on the values at sampled locations, and to model spatial patterns and relationships.
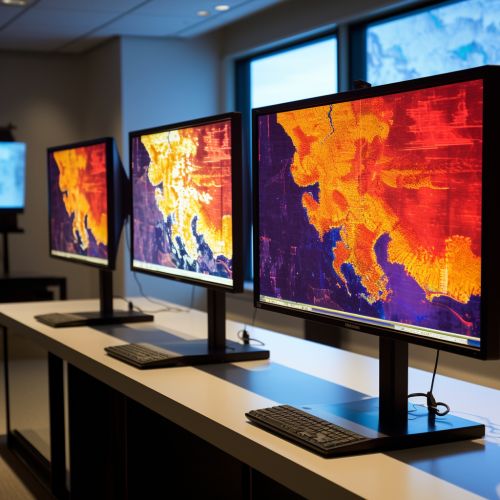
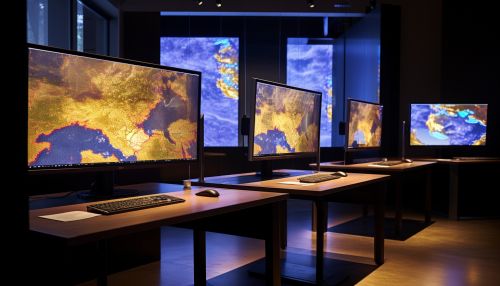
Deforestation: A Global Challenge
Deforestation, the permanent removal of trees to make room for something other than forest, is a global environmental challenge. It contributes to climate change, loss of biodiversity, and disruption of water cycles, among other adverse effects. Predicting deforestation is crucial for proactive environmental management and policy-making.
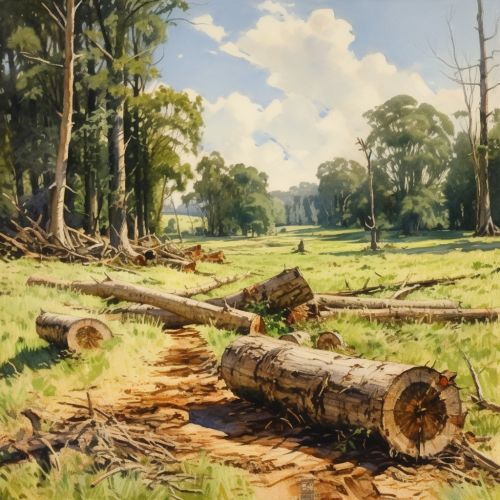
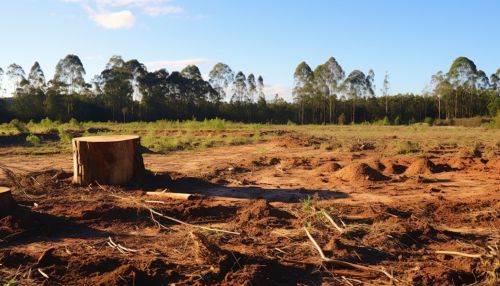
Geostatistics in Predicting Deforestation
Geostatistics plays a vital role in predicting deforestation by providing a statistical framework for analyzing spatial patterns and trends of deforestation and for estimating future deforestation rates and locations. This is achieved through the use of geostatistical models that incorporate various factors influencing deforestation, such as population density, road accessibility, land use policies, and economic factors.
Spatial Autocorrelation and Deforestation
Spatial autocorrelation, a fundamental concept in geostatistics, is particularly relevant in deforestation studies. Deforestation is not a random process; it is influenced by various factors that exhibit spatial patterns. For example, deforestation tends to be more intense near roads and populated areas. By recognizing and quantifying these spatial patterns, geostatistical analysis can provide valuable insights into the drivers of deforestation and help predict future deforestation trends.
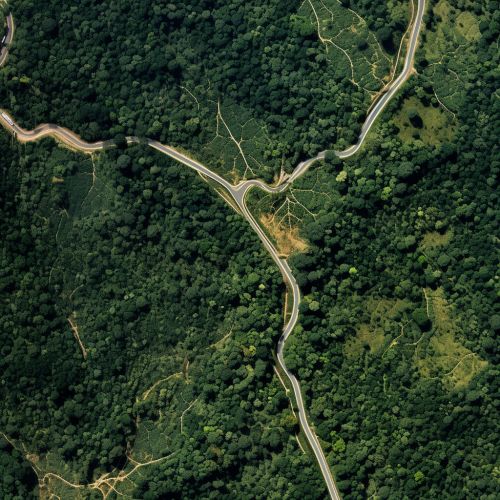
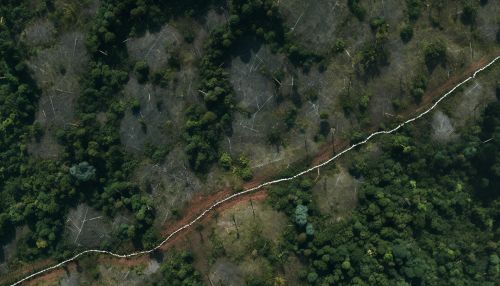
Geostatistical Models for Deforestation Prediction
Geostatistical models for deforestation prediction typically involve the use of variograms and kriging. Variograms are used to quantify the spatial autocorrelation of deforestation, while kriging is used to estimate deforestation rates at unsampled locations, based on the observed deforestation rates at sampled locations. These models can incorporate various factors influencing deforestation, such as distance to roads, population density, land tenure, and economic factors.
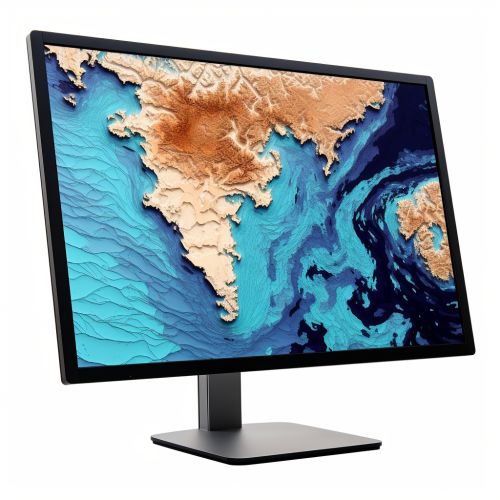
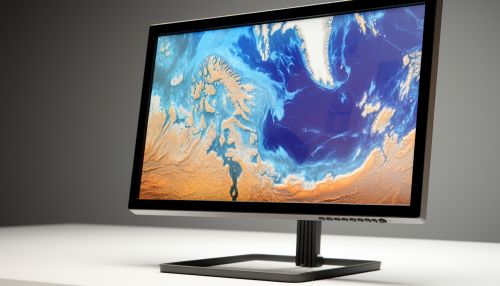
Case Studies
Several studies have demonstrated the effectiveness of geostatistics in predicting deforestation. For example, a study in the Brazilian Amazon used geostatistical models to predict deforestation rates based on factors such as road accessibility, population density, and land use policies. The model accurately predicted the spatial pattern and intensity of deforestation, providing valuable information for environmental management and policy-making.
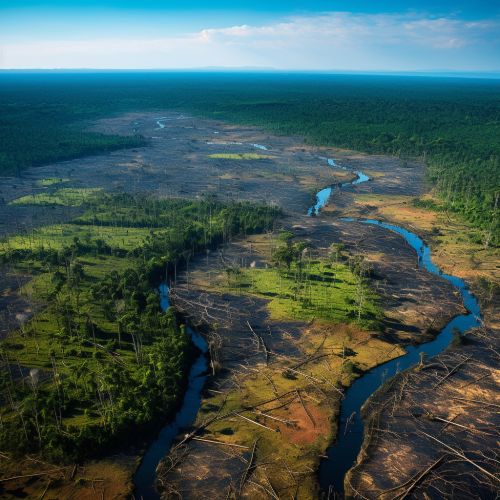
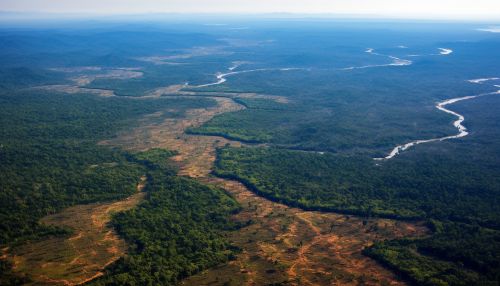
Conclusion
The role of geostatistics in predicting deforestation is increasingly recognized as crucial in the face of ongoing environmental challenges. By providing a statistical framework for analyzing spatial patterns and trends of deforestation and for estimating future deforestation rates and locations, geostatistics offers a powerful tool for proactive environmental management and policy-making.