The Role of Geostatistics in Predicting Climate Change Impacts
Introduction
Geostatistics is a branch of statistics that deals with spatial or spatiotemporal datasets. It has been increasingly utilized in the prediction of climate change impacts. This article explores the role of geostatistics in predicting climate change impacts, providing a comprehensive and detailed analysis of the subject.
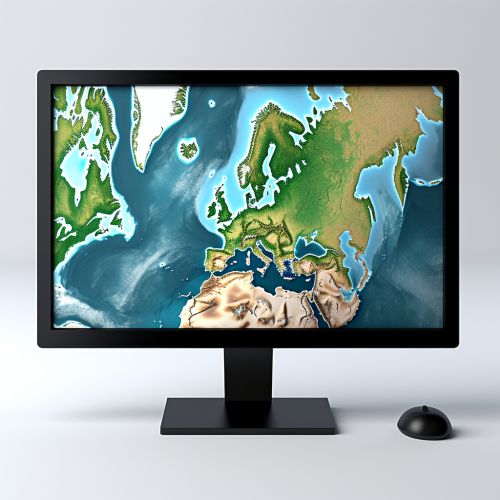
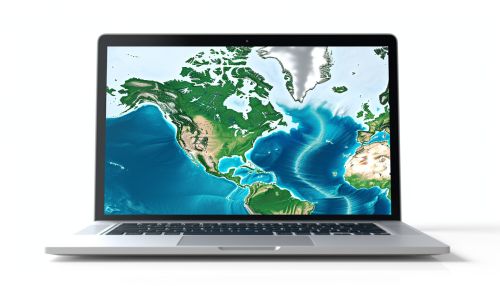
Geostatistics: An Overview
Geostatistics originated from the mining and petroleum industries, where it was used to predict the likely locations of valuable resources. Today, it is used in a wide range of fields, including environmental science, agriculture, public health, and climate change research.
Geostatistics is based on the concept of spatial autocorrelation, which posits that measurements taken at locations close to each other are more likely to be similar than measurements taken at locations far apart. This principle is encapsulated in Tobler's First Law of Geography: "Everything is related to everything else, but near things are more related than distant things."
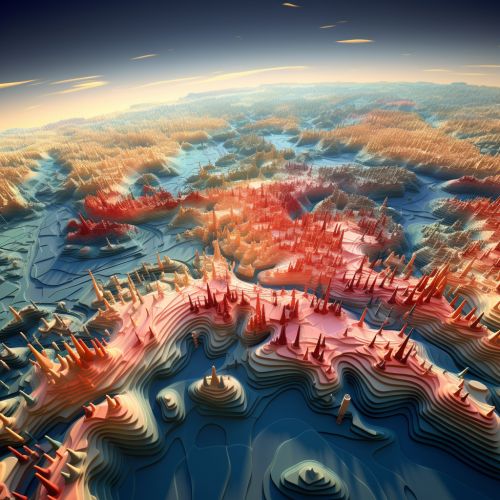
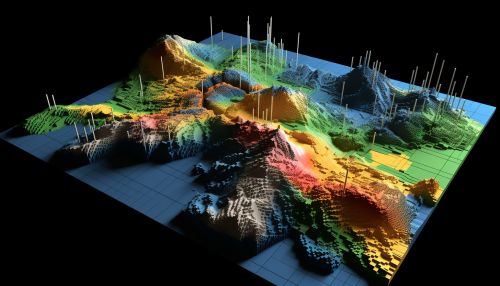
Geostatistics and Climate Change
Climate change is a complex phenomenon that involves changes in temperature, precipitation, wind patterns, and other climatic factors over time. Predicting the impacts of climate change requires sophisticated statistical tools that can handle the spatial and temporal complexity of climate data.
Geostatistics provides such tools. By accounting for spatial autocorrelation, geostatistics allows researchers to make more accurate predictions about the impacts of climate change. For example, geostatistical models can be used to predict how rising temperatures will affect the distribution of plant and animal species, or how changes in precipitation patterns will impact water availability.
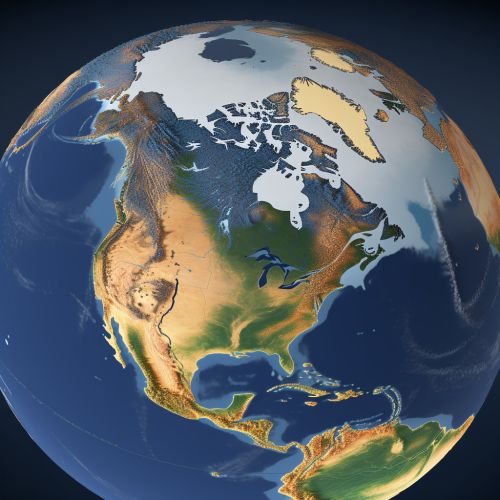
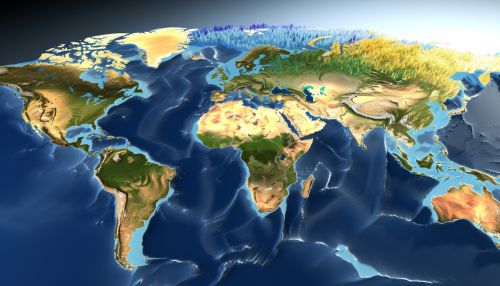
Geostatistical Methods in Climate Change Research
Several geostatistical methods are commonly used in climate change research. These include kriging, spatial regression, and geographically weighted regression.
Kriging is a method of interpolation that uses spatial autocorrelation to predict values at unobserved locations. In the context of climate change research, kriging can be used to predict temperature or precipitation levels at locations where no measurements have been taken.
Spatial regression is a statistical technique that incorporates spatial autocorrelation into regression models. This allows researchers to account for the spatial structure of climate data, leading to more accurate predictions.
Geographically weighted regression (GWR) is a local version of spatial regression that allows the relationship between the dependent and independent variables to vary across space. This makes GWR particularly useful for modeling complex phenomena like climate change, where the impacts can vary widely from one location to another.
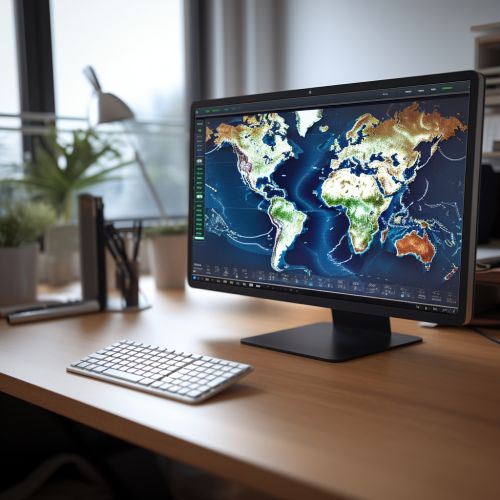
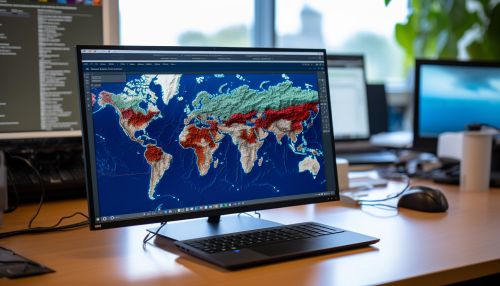
Challenges and Opportunities
While geostatistics offers powerful tools for predicting the impacts of climate change, it also presents several challenges. One of the main challenges is the need for high-quality spatial data. Without accurate and detailed data on climate variables, geostatistical models cannot make accurate predictions.
Despite these challenges, the use of geostatistics in climate change research is likely to increase in the future. As more high-quality spatial data becomes available, and as geostatistical methods become more sophisticated, the ability to predict the impacts of climate change will continue to improve.
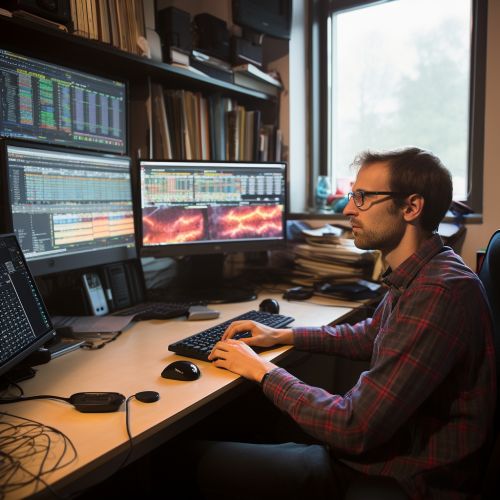
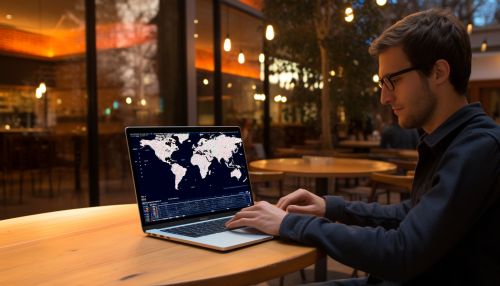
Conclusion
Geostatistics plays a crucial role in predicting the impacts of climate change. By accounting for spatial autocorrelation, geostatistical methods allow researchers to make more accurate predictions about how climate change will affect different geographical areas. Despite the challenges associated with using geostatistics, the field offers significant opportunities for improving our understanding of climate change and its impacts.