The Role of Geostatistics in Predicting Air Pollution
Introduction
Geostatistics, a branch of statistics focusing on spatial or spatiotemporal datasets, plays a crucial role in predicting air pollution. This branch of statistics emerged in the mid-20th century, primarily through the work of the French mathematician Georges Matheron, who developed the foundational principles of geostatistical theory. Geostatistics is used in a variety of fields, including environmental science, where it is instrumental in modeling and predicting air pollution.
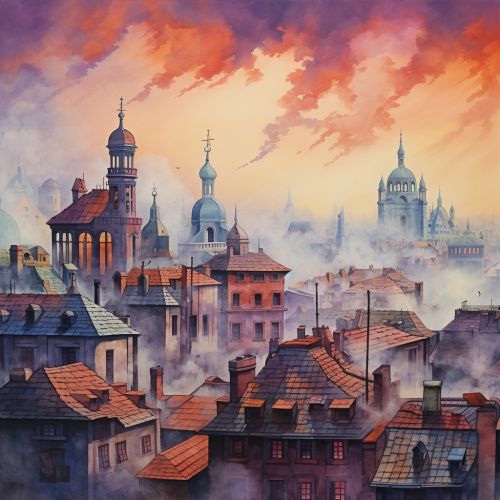
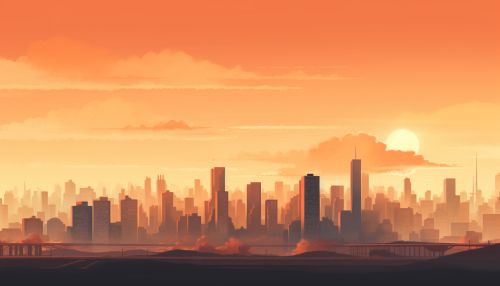
Geostatistics: An Overview
Geostatistics is a discipline that deals with the statistical analysis of spatial or spatiotemporal phenomena. It involves the collection, analysis, and interpretation of data that are associated with geographic locations. The primary objective of geostatistics is to predict the values of a random field at unobserved locations based on observations at known locations. This is achieved through a variety of statistical techniques, including kriging, variogram analysis, and spatial autocorrelation.
The Problem of Air Pollution
Air pollution is a significant environmental issue that affects both the natural environment and human health. It is caused by the introduction of harmful substances into the Earth's atmosphere, primarily from human activities such as the burning of fossil fuels, industrial processes, and vehicle emissions. Air pollution can have severe impacts on human health, including respiratory diseases, cardiovascular diseases, and premature death. It also contributes to climate change and the degradation of ecosystems.
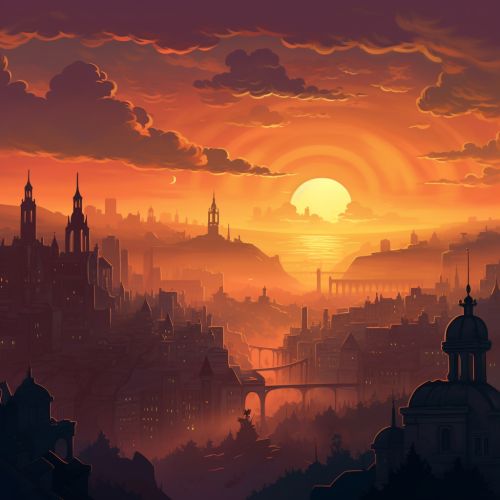
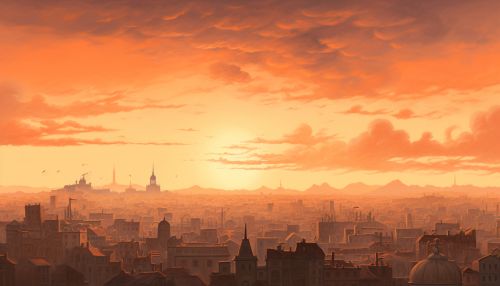
Geostatistical Techniques in Predicting Air Pollution
Geostatistical techniques are widely used in the prediction of air pollution. These techniques allow for the estimation and prediction of pollutant concentrations at unobserved locations based on observed data. This is particularly useful in areas where air quality monitoring stations are sparse or non-existent.
Kriging
Kriging is a key geostatistical technique used in the prediction of air pollution. Named after the South African mining engineer Danie G. Krige, kriging is a method of interpolation that predicts the value of a variable at an unobserved location based on the values at observed locations. Kriging takes into account the spatial correlation between observations, which is often ignored by other interpolation methods.
Variogram Analysis
Variogram analysis is another important geostatistical technique used in air pollution prediction. A variogram is a function that describes the degree of spatial dependence of a spatial random field or stochastic process. Variogram analysis allows for the characterization of spatial patterns and the identification of spatial trends in air pollution data.
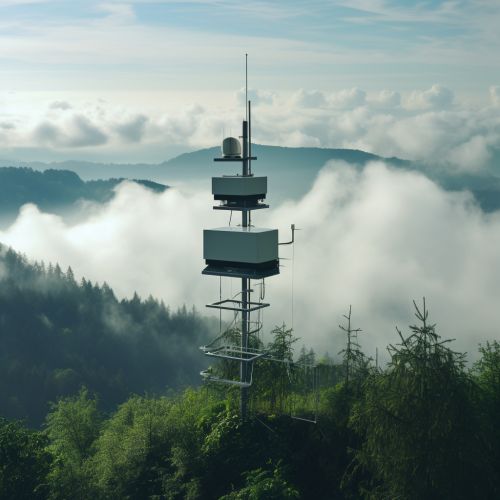
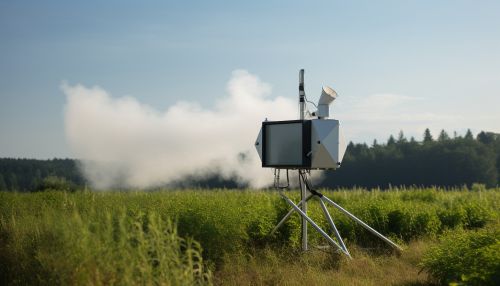
Spatial Autocorrelation
Spatial autocorrelation is a measure of the degree to which a set of spatial features and their associated values tend to be clustered together in space. In the context of air pollution, spatial autocorrelation can be used to identify areas of high pollution concentration.
Applications of Geostatistics in Air Pollution Prediction
Geostatistics has been applied in numerous studies to predict air pollution. For instance, geostatistical techniques have been used to estimate and map the spatial distribution of pollutants such as particulate matter (PM), nitrogen dioxide (NO2), and ozone (O3) in various geographic areas. These studies have provided valuable insights into the spatial patterns of air pollution and have informed the development of air quality management strategies.
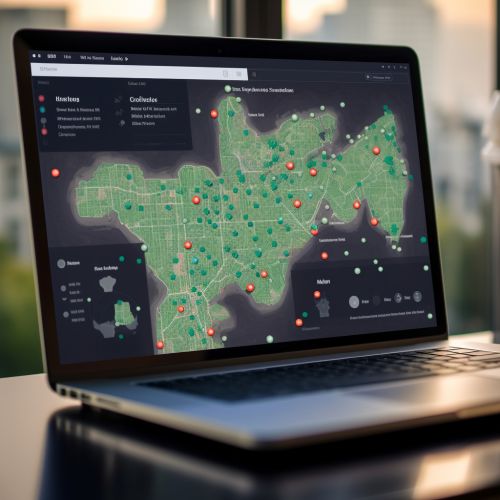
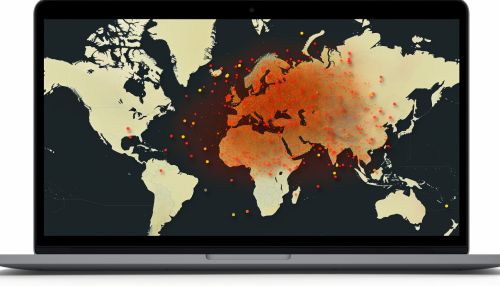
Limitations and Future Directions
While geostatistics provides powerful tools for predicting air pollution, it also has its limitations. For instance, geostatistical models often assume stationarity, which means that the statistical properties of the data do not change over space. However, this assumption is often violated in environmental data, which can lead to inaccurate predictions.
Despite these limitations, the field of geostatistics continues to evolve, with new methods and techniques being developed to address these challenges. Future directions in geostatistics may include the development of non-stationary models, the integration of geostatistics with other data science techniques, and the application of geostatistics in the prediction of other environmental phenomena.