The Role of Geostatistics in Geothermal Energy Exploration
Introduction
Geostatistics is a branch of statistics that deals with spatial or spatiotemporal datasets. Developed originally to predict probability distributions of ore grades for mining operations, it is currently applied in diverse disciplines including petroleum geology, hydrogeology, hydrology, meteorology, oceanography, geochemistry, geometallurgy, geography, forestry, environmental control, landscape ecology, soil science, and agriculture (crop yield estimation). In the context of geothermal energy exploration, geostatistics plays a crucial role in the identification, assessment, and prediction of geothermal resources.
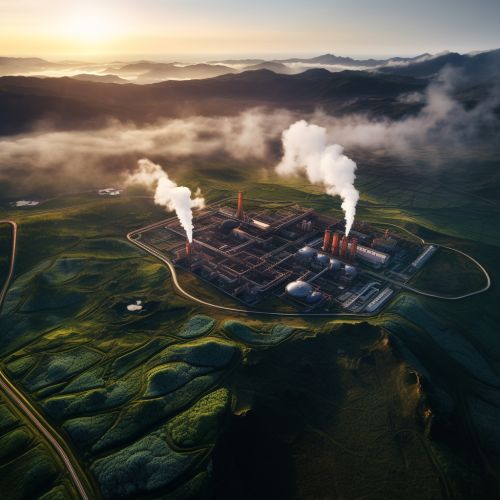
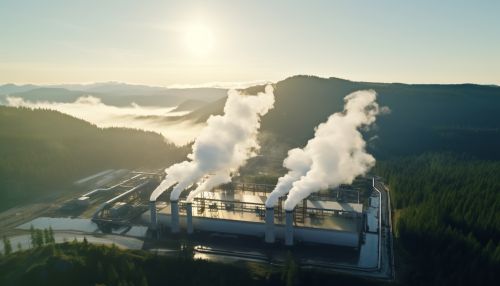
Geostatistics and Geothermal Energy
Geothermal energy is the heat derived within the sub-surface of the earth. It is a sustainable and clean energy source that can provide power for electricity generation and heating purposes. The exploration of geothermal energy involves the study of geological conditions, such as the presence of geothermal reservoirs, the heat flow in the earth's crust, and the geothermal gradient. Geostatistics aids in the exploration process by providing statistical tools to analyze the spatial distribution of these geological variables.
The primary goal of geostatistics in geothermal energy exploration is to predict the spatial distribution of geological variables based on a limited number of observations. This is achieved through the use of statistical methods that take into account the spatial correlation between observations. The spatial correlation is quantified by the semivariogram, a function that describes the degree of spatial dependence of a variable.
Geostatistical Methods in Geothermal Energy Exploration
There are several geostatistical methods used in geothermal energy exploration. These include:
Kriging
Kriging is a method of interpolation that predicts the value of a variable at an unobserved location based on observed values at nearby locations. In geothermal energy exploration, kriging can be used to predict the temperature at a given depth in the earth's crust based on temperature measurements at other depths.
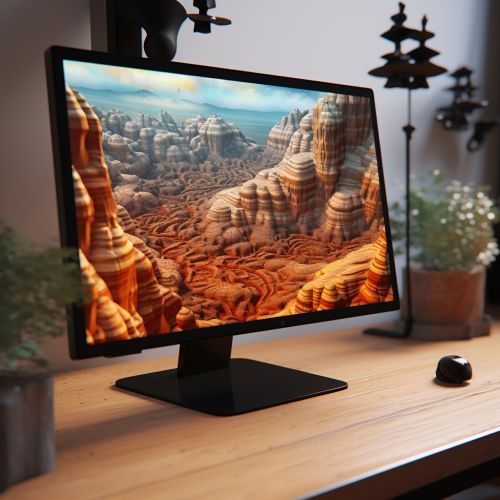
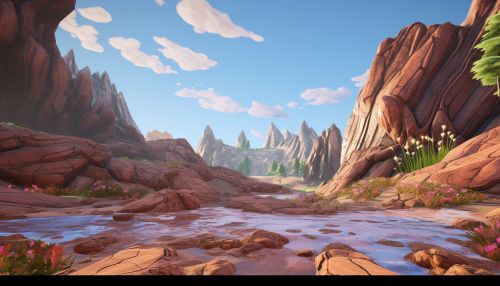
Sequential Gaussian Simulation
Sequential Gaussian Simulation (SGS) is a geostatistical method used to generate multiple equally probable realizations of a geological variable. In geothermal energy exploration, SGS can be used to assess the uncertainty in the prediction of the spatial distribution of temperature or permeability in a geothermal reservoir.
Co-Kriging
Co-kriging is a method of interpolation that uses the correlation between two or more variables to improve the prediction of one variable based on the observed values of the other variables. In geothermal energy exploration, co-kriging can be used to predict the temperature at a given depth based on temperature measurements at other depths and the observed values of other geological variables, such as rock type or porosity.
Applications of Geostatistics in Geothermal Energy Exploration
Geostatistics is used in various stages of geothermal energy exploration, including:
Resource Identification
Geostatistics can be used to identify potential geothermal resources by predicting the spatial distribution of geological variables that indicate the presence of a geothermal reservoir. For example, kriging can be used to interpolate the temperature at a given depth based on temperature measurements at other depths, helping to identify areas with high geothermal potential.
Resource Assessment
Once a potential geothermal resource has been identified, geostatistics can be used to assess the resource. This involves predicting the spatial distribution of geological variables, such as temperature and permeability, in the geothermal reservoir. Sequential Gaussian Simulation can be used to generate multiple realizations of these variables, providing a measure of the uncertainty in the resource assessment.
Resource Prediction
Geostatistics can also be used to predict the future performance of a geothermal reservoir. This involves predicting the spatial distribution of geological variables over time, taking into account the extraction of heat from the reservoir. Co-kriging can be used to improve the prediction of temperature based on the observed values of other variables, such as pressure or flow rate.
Conclusion
Geostatistics plays a vital role in geothermal energy exploration by providing statistical tools to analyze and predict the spatial distribution of geological variables. Its applications range from resource identification and assessment to resource prediction, helping to optimize the exploration and extraction of geothermal energy. Despite its complexity, the use of geostatistics in geothermal energy exploration is essential for the sustainable and efficient use of this renewable energy source.
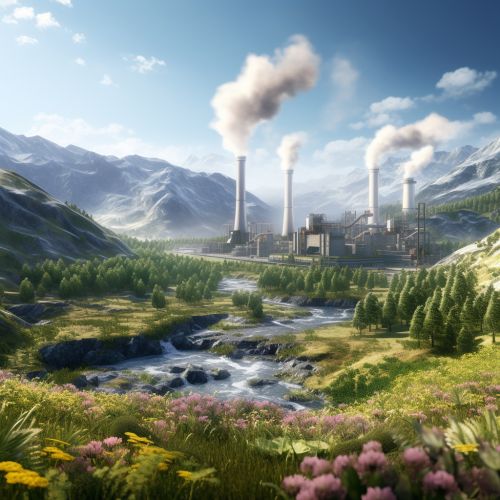
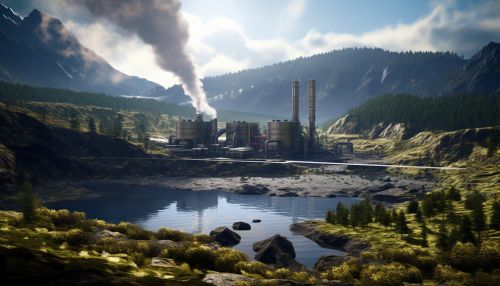