The Role of Geostatistics in Environmental Studies
Introduction
Geostatistics is a branch of statistics focusing on spatial or spatiotemporal datasets. Developed originally to predict probability distributions of ore grades for mining operations, it is currently applied in diverse disciplines including petroleum geology, hydrogeology, hydrology, meteorology, oceanography, geochemistry, geometallurgy, geography, forestry, environmental control, landscape ecology, soil science, and agriculture (crop yield estimation). In environmental studies, geostatistics plays a crucial role in analyzing spatially correlated data, predicting environmental variables at unobserved locations, and quantifying uncertainty associated with these predictions.
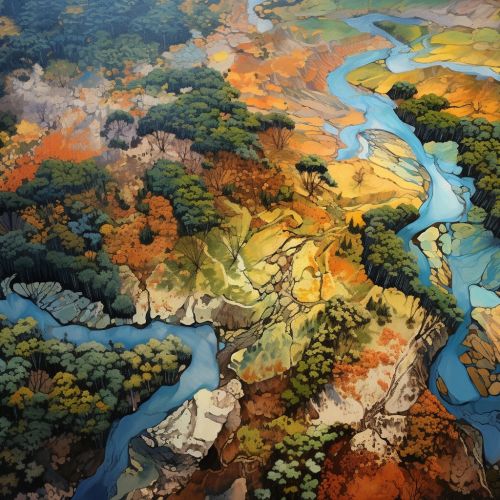
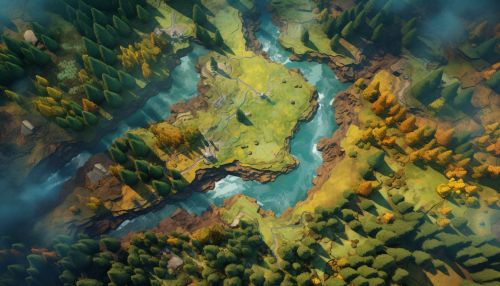
Principles of Geostatistics
Geostatistics operates under the premise that spatially distributed phenomena are not random and can be statistically quantified. This is based on the principle of spatial autocorrelation, which states that measurements taken at locations closer together are more likely to be similar than those taken farther apart. This principle is often referred to as the first law of geography.
The key concepts in geostatistics include spatial autocorrelation, spatial heterogeneity, spatial dependence, and spatial variability. These concepts are used to describe and quantify the spatial patterns of environmental variables.
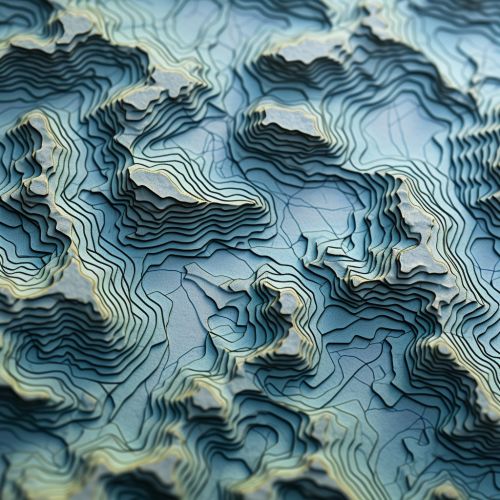
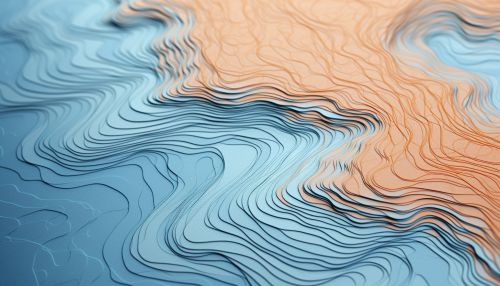
Geostatistical Techniques
Geostatistical techniques are mathematical methods used to analyze spatially correlated data. These techniques include kriging, spatial interpolation, variogram modeling, and geostatistical simulation.
Kriging, for instance, is a method of interpolation that takes into account the spatial autocorrelation of data. It is often used in environmental studies to predict values at unobserved locations based on observed data. Variogram modeling, on the other hand, is used to quantify the spatial autocorrelation of data.
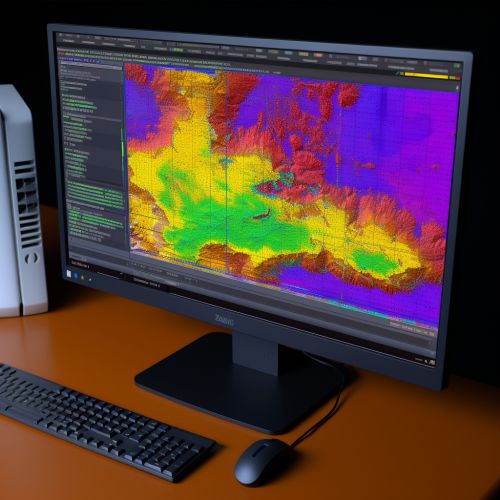
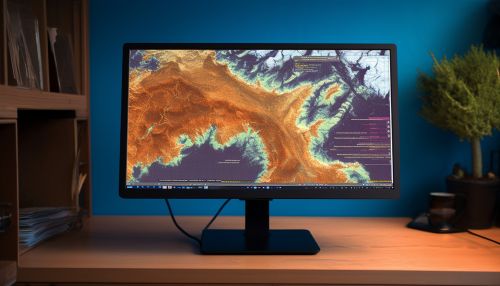
Application in Environmental Studies
Geostatistics is widely applied in environmental studies to analyze and predict the spatial distribution of environmental variables. These variables may include air and water quality parameters, soil properties, vegetation patterns, and animal distributions, among others.
For example, in air quality studies, geostatistics can be used to predict the spatial distribution of air pollutants based on monitoring data. In soil studies, it can be used to analyze the spatial variability of soil properties and predict their values at unobserved locations. In ecology, geostatistics can be used to analyze the spatial patterns of species distributions.
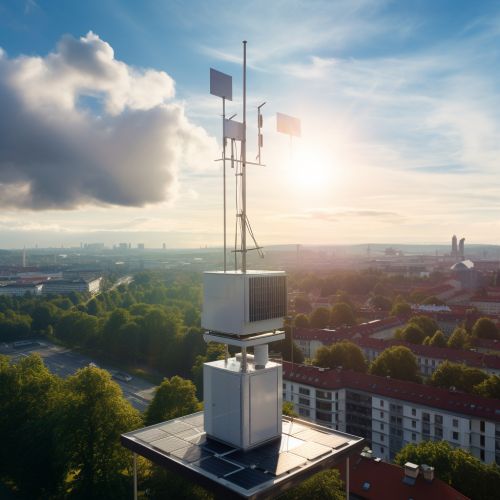
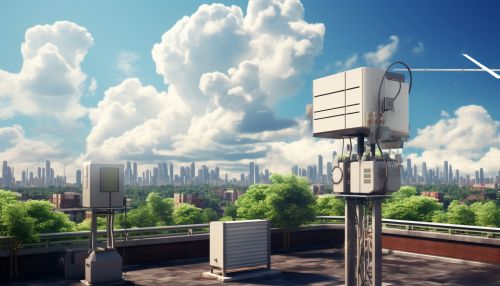
Limitations and Challenges
While geostatistics provides powerful tools for analyzing spatially correlated data, it also has its limitations and challenges. These include the assumption of stationarity, the difficulty in determining the appropriate spatial scale, and the complexity of geostatistical models.
The assumption of stationarity, which assumes that the statistical properties of a variable do not change over space, is often violated in environmental studies due to the inherent variability of environmental variables. Determining the appropriate spatial scale for analysis is also challenging, as environmental variables can exhibit different patterns at different scales. Furthermore, geostatistical models can be complex and require a high level of statistical expertise to implement and interpret.
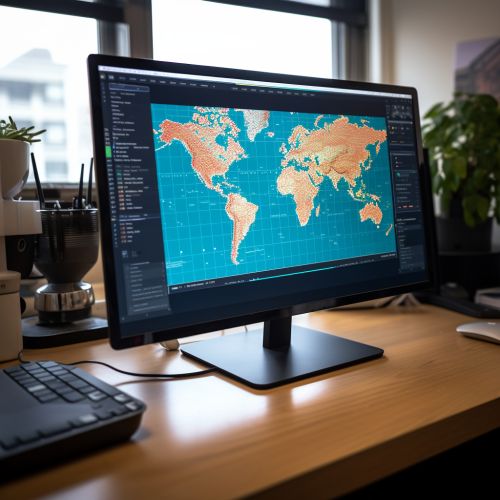
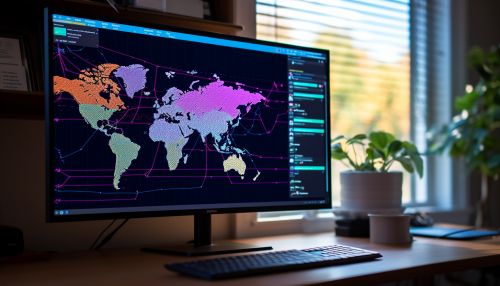
Conclusion
Despite its limitations and challenges, geostatistics remains a valuable tool in environmental studies. Its ability to analyze spatially correlated data and predict values at unobserved locations makes it indispensable in the study and management of environmental variables. As technology advances and more spatial data becomes available, the role of geostatistics in environmental studies is expected to become even more significant.
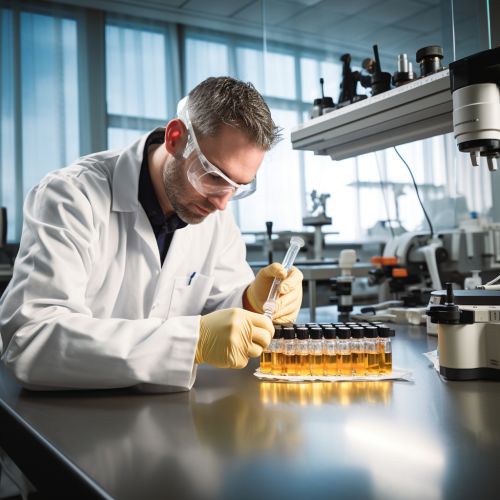
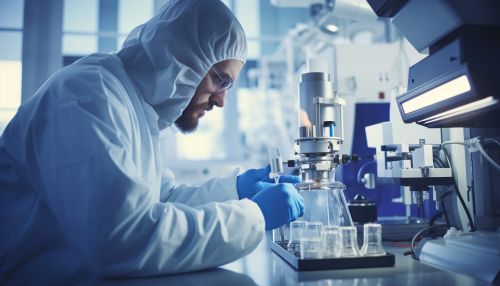