Probability Sampling
Overview
Probability sampling is a statistical method used in research to select a subset of individuals from a larger population. This method ensures that every individual within the population has an equal chance of being selected, which helps to eliminate bias and improve the reliability and validity of the results. The main types of probability sampling include simple random sampling, systematic sampling, stratified sampling, and cluster sampling.
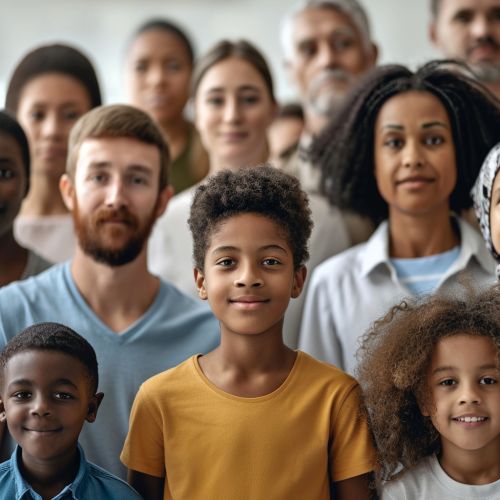
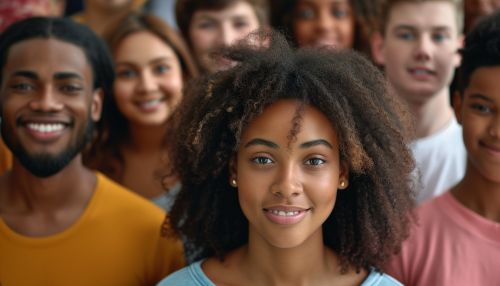
Principles of Probability Sampling
Probability sampling is based on the principles of statistical theory. It assumes that the population is large, diverse, and well-defined. The key principle is that each individual in the population has an equal chance of being selected. This is achieved by using a random selection process, which can be done manually or using a computer program. The randomness ensures that the sample is representative of the population, which allows the researcher to make generalizations about the population based on the sample data.
Types of Probability Sampling
There are several types of probability sampling, each with its own advantages and disadvantages. The choice of method depends on the nature of the population, the purpose of the research, and the resources available.
Simple Random Sampling
Simple random sampling is the most basic form of probability sampling. It involves selecting individuals from the population purely by chance. Each individual has an equal probability of being selected, and the selection of one individual does not affect the selection of others. This method is easy to understand and implement, but it may not be the most efficient or practical for large populations.
Systematic Sampling
Systematic sampling involves selecting every nth individual from the population. The starting point is chosen at random, and then every nth individual is selected. This method is more efficient than simple random sampling, especially for large populations. However, it may introduce bias if there is a pattern in the population that corresponds with the sampling interval.
Stratified Sampling
Stratified sampling involves dividing the population into homogeneous subgroups or strata, and then selecting a simple random sample from each stratum. This method ensures that all subgroups are adequately represented in the sample. It is more efficient and accurate than simple random sampling, but it requires detailed knowledge of the population and more complex data analysis.
Cluster Sampling
Cluster sampling involves dividing the population into heterogeneous subgroups or clusters, and then selecting a simple random sample of clusters. All individuals within the selected clusters are included in the sample. This method is efficient for large, geographically dispersed populations, but it may be less accurate than other methods due to intra-cluster correlation.
Advantages and Disadvantages of Probability Sampling
Probability sampling has several advantages. It eliminates bias in the selection process, ensures that the sample is representative of the population, and allows the researcher to estimate the sampling error and make statistical inferences about the population. However, it also has some disadvantages. It requires a complete list of the population, which may not always be available or feasible to obtain. It can be time-consuming and costly, especially for large populations. And it may not be practical or ethical for certain types of research, such as studies involving sensitive topics or vulnerable populations.
Applications of Probability Sampling
Probability sampling is widely used in various fields, including sociology, psychology, political science, public health, market research, and education. It is used in surveys, experiments, observational studies, and other types of research. It is also used in quality control, auditing, and other business applications.