Sampling Error
Introduction
Sampling error refers to the discrepancy between a sample statistic used to estimate a population parameter and the actual but unknown value of the parameter. In statistics, this error is an inherent part of the sampling process and can only be reduced by increasing the sample size or applying a more appropriate sampling method.
Understanding Sampling Error
Sampling error occurs because the characteristics of the individuals in the sample do not perfectly reflect the characteristics of the individuals in the population. For example, if we were to take a sample of individuals from a larger population, the mean age of the sample might not be the same as the mean age of the population. This discrepancy is the sampling error.
Sources of Sampling Error
Sampling error can arise from a variety of sources. Some of the most common include:
Non-Response
Non-response occurs when some subjects do not have the opportunity to participate in the survey. This can occur if the subjects are not contacted or refuse to respond.
Selection Bias
Selection bias occurs when the method of selecting subjects into the sample is such that a biased sample is obtained.
Sampling Frame Error
Sampling frame error occurs when the wrong sub-population is used to select a sample.
Measuring Sampling Error
The standard error is a commonly used measure of sampling error. It is the standard deviation of the sampling distribution of a statistic, usually the mean. The standard error decreases as the sample size increases, as the extent of chance variation is reduced.
Reducing Sampling Error
There are several ways to reduce sampling error. Some of the most common methods include increasing the sample size, using stratified sampling, and improving the response rate.
Conclusion
While sampling error is an inherent part of the sampling process, understanding its sources and how to measure it can help researchers to minimize its impact and improve the accuracy of their results.
See Also
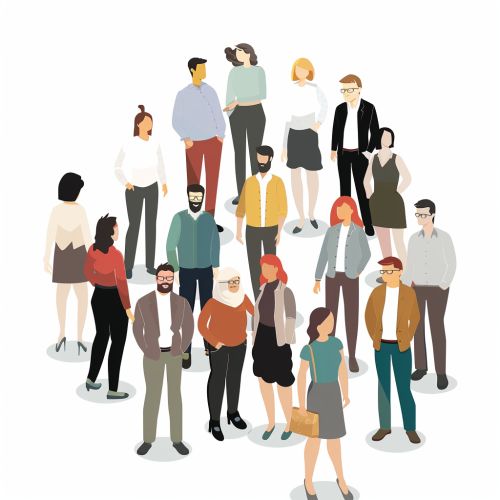
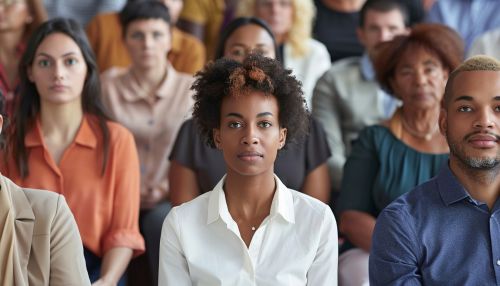