Sampling Bias
Introduction
Sampling bias is a statistical bias that occurs when a sample is collected in such a way that some members of the intended population have a lower or higher sampling probability than others. It results in a biased sample, a non-random sample of a population (or non-human factors) in which all individuals, or instances, were not equally likely to have been selected. If this bias is not taken into account, then certain conclusions may be reached that are not accurate reflections of the full population.
Types of Sampling Bias
There are several types of sampling bias, including selection bias, nonresponse bias, and undercoverage bias.
Selection Bias
Selection bias, also known as selection effect, is the bias introduced by the selection of individuals, groups or data for analysis in such a way that proper randomization is not achieved, thereby ensuring that the sample obtained is not representative of the population intended to be analyzed. It is sometimes referred to as the selection effect. The phrase "selection bias" most often refers to the distortion of a statistical analysis, resulting from the method of collecting samples.
Nonresponse Bias
Nonresponse bias occurs when some respondents included in the sample do not respond. The non-respondents may differ in meaningful ways from those who have responded, thus biasing the estimates. For instance, in a survey on eating habits, if people with poor diets are less likely to respond, then this could bias the estimate of the number of people with poor diets upwards.
Undercoverage Bias
Undercoverage bias occurs when some groups of the population are inadequately represented in the sample. A classic example of undercoverage is the Literary Digest voter survey, which predicted that Alfred Landon would beat Franklin Roosevelt in the 1936 Presidential Election. The survey sample suffered from undercoverage of low-income voters who tended to be Democrats.
Causes of Sampling Bias
Sampling bias can be caused by a variety of factors, ranging from the method used to select the sample to the way in which data is collected. Some of the most common causes include:
- Non-random sample: If the sample is not selected randomly, it may not be representative of the population. This can lead to bias in the results.
- Self-selection: This occurs when individuals select themselves into a group, causing a biased sample with nonprobability sampling. It is commonly seen in surveys or polls that are distributed to a large population where it is left to the individuals to decide whether to respond.
- Time period: The time period in which the sample is collected can also introduce bias. For example, a survey conducted on a Monday morning may not be representative of the population as a whole.
- Pre-screening or advertising: This can lead to a non-representative sample of people being surveyed. For instance, if a company wants to survey people who have seen their advertisement, the sample will not be representative of the general population.
Effects of Sampling Bias
Sampling bias can significantly affect the results of a study or survey. It can lead to inaccurate results and conclusions, and can therefore mislead researchers or policy makers. Some potential effects include:
- Inaccurate estimation of population parameters: If the sample is not representative of the population, the estimates of population parameters such as mean, proportion, etc., may be inaccurate.
- Misleading conclusions: If the sample is biased, the conclusions drawn from the study may be misleading. For example, if a study on smoking and lung cancer only includes smokers, it may incorrectly conclude that all smokers will develop lung cancer.
- Reduced generalizability: If the sample is not representative of the population, the findings of the study may not be generalizable to the population.
Mitigating Sampling Bias
There are several strategies that researchers can use to mitigate the effects of sampling bias. These include:
- Random sampling: This is the best way to avoid sampling bias. In a random sample, every member of the population has an equal chance of being selected.
- Stratified sampling: In this method, the population is divided into subgroups, or strata, and a random sample is taken from each stratum. This can ensure that the sample is representative of the population.
- Oversampling: This involves sampling a larger proportion of a minority group to ensure that they are adequately represented in the sample.
- Weighting: This involves adjusting the results of a study to compensate for patterns of nonresponse or undercoverage.
Conclusion
Sampling bias is a common issue that can significantly affect the results of a study or survey. It is important for researchers to be aware of the potential for sampling bias and to take steps to mitigate its effects. By using strategies such as random sampling, stratified sampling, oversampling, and weighting, researchers can help ensure that their samples are representative of the populations they are studying, leading to more accurate and reliable results.
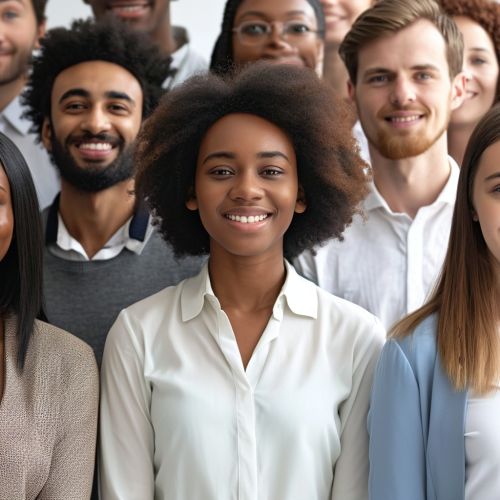
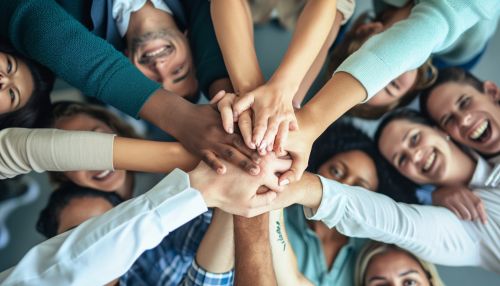
See Also
- Survey Methodology - Statistical Bias - Observational Study