Poisson Distribution
Introduction
The Poisson distribution is a discrete probability distribution that expresses the probability of a given number of events occurring in a fixed interval of time or space if these events occur with a known constant mean rate and independently of the time since the last event. Named after French mathematician Siméon Denis Poisson, it is an important concept in statistics and probability theory.
Definition
The Poisson distribution can be defined mathematically in terms of the Poisson process. In a Poisson process, events occur continuously and independently at a constant average rate. The probability of a given number of events occurring in a fixed interval of time is given by the Poisson probability mass function:
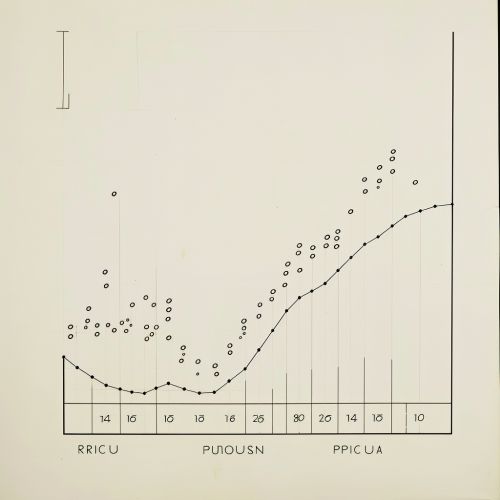
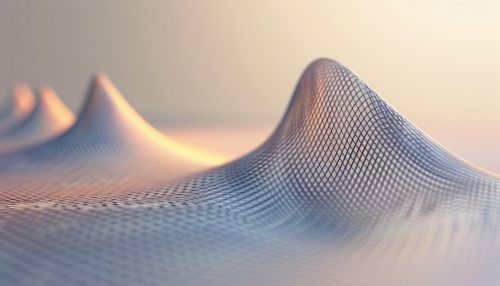
This formula calculates the probability of observing exactly k events in an interval, given the average rate of occurrence, λ. The symbol e is the base of the natural logarithm, and k! denotes the factorial of k.
Properties
The Poisson distribution has several important properties.
- Discreteness: The Poisson distribution is discrete, meaning that it only takes on integer values. This makes it suitable for modeling count data, such as the number of phone calls received in a call center per hour.
- Memorylessness: The Poisson distribution is memoryless, meaning that the probability of an event occurring in the future does not depend on the past. This property is shared with the exponential distribution, which is the continuous counterpart of the Poisson distribution.
- Skewness: The Poisson distribution is positively skewed, with the skewness decreasing as the mean increases. This means that for small mean values, the distribution is heavily skewed to the right, with a long tail of rare, high-count events.
- Variance equals mean: In a Poisson distribution, the variance is equal to the mean. This property is unique to the Poisson distribution and is a consequence of the independence of events in a Poisson process.
Applications
The Poisson distribution has wide applications in various fields.
- Telecommunications: The Poisson distribution is used in telecommunications to model the number of packets arriving at a router or the number of phone calls arriving at a call center.
- Biology: In biology, the Poisson distribution is used to model the number of mutations in a given stretch of DNA or the number of neurons firing in a particular brain region.
- Reliability Engineering: In reliability engineering, the Poisson distribution is used to model the number of failures of a system over a given period of time.
- Queueing Theory: In queueing theory, the Poisson distribution is used to model the arrival of customers to a service station.
Limitations
While the Poisson distribution is a powerful tool, it has its limitations. It assumes that events occur independently, which is not always the case in real-world scenarios. For example, the number of visitors to a website may depend on the time of day, or the number of failures of a machine may depend on its age. In such cases, other distributions such as the negative binomial distribution or the gamma distribution may be more appropriate.