Poisson Process
Introduction
The Poisson process is a fundamental concept in probability theory and statistics, named after the French mathematician Siméon Denis Poisson. It is a type of stochastic process that models a series of events occurring randomly in time and space. The Poisson process is characterized by the property that the number of events occurring in non-overlapping intervals are independent.
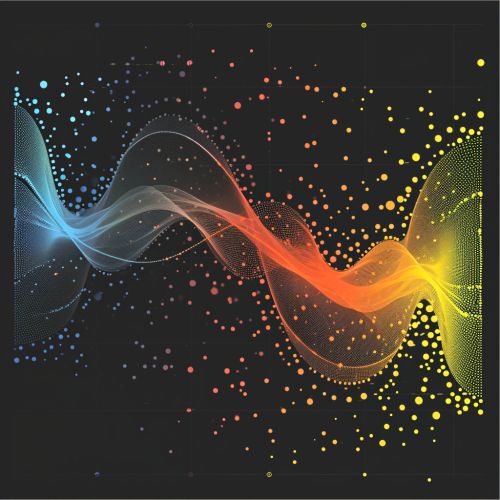

Definition
Formally, a Poisson process is a counting process, a stochastic process that represents a series of events, such that the following conditions are met:
1. The number of events in non-overlapping intervals are independent. This is known as the independence property. 2. The probability of an event occurring in a small interval of length h is proportional to h. This is known as the stationarity property. 3. The probability of more than one event occurring in an interval of length h goes to zero as h goes to zero. This is known as the regularity property.
These properties define the Poisson process and differentiate it from other types of stochastic processes.
Properties
The Poisson process has several important properties that make it a useful model in many fields. These include:
1. Stationarity: The number of events in any interval of time or space depends only on the length of the interval, not on the location of the interval. This property is also known as homogeneity. 2. Independence: The number of events in non-overlapping intervals are independent. This means that knowing the number of events in one interval does not provide any information about the number of events in another interval. 3. Rare events: The probability of two or more events occurring in a very small interval is essentially zero. This property reflects the idea that the events modeled by a Poisson process are rare.
Applications
The Poisson process is widely used in various fields of study due to its mathematical tractability and conceptual simplicity. Some of the fields where the Poisson process finds application include:
1. Queueing theory: The Poisson process is used to model the arrival of customers in a queueing system. 2. Telecommunications: In telecommunications, the Poisson process models the arrival of packets in a network. 3. Reliability engineering: The Poisson process is used to model the failure of components in a system. 4. Biology: In biology, the Poisson process is used to model random events such as mutation and birth processes.
Extensions and Generalizations
The Poisson process has been extended and generalized in various ways to model more complex situations. Some of these extensions include:
1. Nonhomogeneous Poisson process: This is a generalization of the Poisson process where the rate of event occurrence can vary with time or space. 2. Compound Poisson process: This is a Poisson process where each event is associated with a random amount. 3. Poisson cluster process: This is a Poisson process where events tend to cluster together. 4. Marked Poisson process: This is a Poisson process where each event is marked with additional information.
Conclusion
The Poisson process is a fundamental concept in probability theory and statistics, with wide-ranging applications in various fields. Its properties of stationarity, independence, and rare events make it a useful model for many types of random events. Furthermore, the Poisson process has been extended and generalized in various ways to model more complex situations.