Point process
Introduction
A point process, also known as a point pattern, is a mathematical model used to describe phenomena that can be represented as points randomly scattered in a space. The space can be one-dimensional, two-dimensional, three-dimensional, or even higher dimensional, depending on the application. Point processes are widely used in fields such as statistics, physics, geography, computer science, and telecommunications, among others.
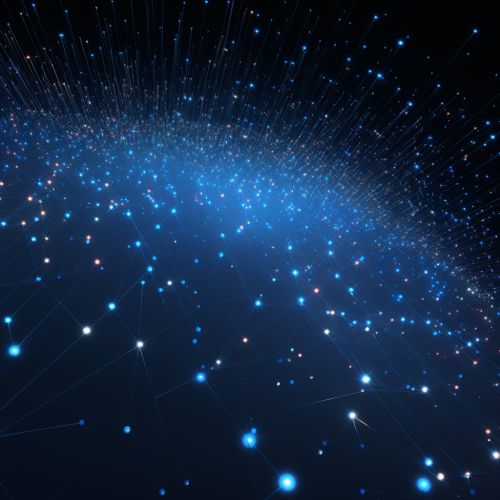

Definition
Formally, a point process is a collection of random points in a mathematical space, such as the real line or Euclidean space. Each point in the process is typically associated with an event, and the location of the point represents the time or place where the event occurred. The number of points in a given region of the space is a random variable, and the distribution of these points forms the basis of the point process.
Types of Point Processes
There are several types of point processes, each with its own characteristics and applications. Some of the most common types include:
Poisson Point Process
The Poisson point process is one of the most fundamental and widely used point processes. It is named after the French mathematician Siméon Denis Poisson, who first introduced the concept in the 19th century. The Poisson point process is characterized by its homogeneity and independence. Homogeneity means that the points are uniformly distributed in space, while independence means that the location of each point is independent of the locations of all other points.
Cox Point Process
The Cox point process, also known as a doubly stochastic Poisson process, is a generalization of the Poisson point process. In a Cox process, the intensity function, which determines the density of points in the space, is itself a random function. This allows for more flexibility in modeling point patterns that exhibit variability in their intensity.
Neyman-Scott Point Process
The Neyman-Scott point process is a type of cluster process, where points tend to group together in clusters. This type of process is often used to model phenomena where events occur in groups, such as the locations of trees in a forest or the distribution of stars in the sky.
Applications of Point Processes
Point processes have a wide range of applications in various fields. Here are a few examples:
In Telecommunications
In the field of telecommunications, point processes are used to model the locations of transmitters and receivers in a wireless network. This can help in the design and analysis of communication protocols, as well as in the prediction of network performance.
In Physics
In physics, point processes can be used to model the locations of particles in a gas or the stars in a galaxy. This can provide insights into the underlying physical processes that govern these systems.
In Environmental Science
In environmental science, point processes can be used to model the locations of trees in a forest, the distribution of animals in a habitat, or the occurrence of earthquakes in a region. This can aid in the study of ecological dynamics and the prediction of natural disasters.
Conclusion
Point processes are a powerful mathematical tool for modeling and analyzing spatial patterns. They provide a flexible framework for representing and studying a wide variety of phenomena, from the distribution of stars in the sky to the locations of mobile phone users in a city. Despite their apparent complexity, point processes are based on simple and intuitive concepts, making them accessible to researchers and practitioners in many different fields.