Kurtosis
Introduction
Kurtosis is a statistical measure used to describe the distribution of observed data around the mean. It is a descriptor of the shape of a probability distribution and provides insights into the tails of the distribution. Specifically, kurtosis measures the "tailedness" of the data distribution, which indicates the presence of outliers and the extremity of deviations from the mean.
Definition and Formula
Kurtosis is mathematically defined as the fourth standardized moment of a distribution. The formula for kurtosis (β2) is:
\[ \beta_2 = \frac{\mu_4}{\sigma^4} \]
where: - \(\mu_4\) is the fourth central moment of the distribution. - \(\sigma\) is the standard deviation of the distribution.
The fourth central moment (\(\mu_4\)) is calculated as:
\[ \mu_4 = \frac{1}{N} \sum_{i=1}^{N} (X_i - \bar{X})^4 \]
where: - \(N\) is the number of observations. - \(X_i\) represents each individual observation. - \(\bar{X}\) is the mean of the observations.
Types of Kurtosis
Kurtosis can be classified into three main types based on the value of β2:
Mesokurtic
A distribution is considered mesokurtic if it has a kurtosis value close to 3. This type of distribution has a similar shape to the normal distribution, with moderate tails. The normal distribution itself is a classic example of a mesokurtic distribution.
Leptokurtic
A leptokurtic distribution has a kurtosis value greater than 3. This indicates that the distribution has heavier tails and a sharper peak compared to a normal distribution. Leptokurtic distributions are characterized by the presence of outliers and extreme values.
Platykurtic
A platykurtic distribution has a kurtosis value less than 3. This type of distribution has lighter tails and a flatter peak compared to a normal distribution. Platykurtic distributions indicate fewer outliers and less extreme deviations from the mean.
Interpretation of Kurtosis
Kurtosis provides valuable information about the tails and peak of a distribution. High kurtosis (leptokurtic) suggests that data have heavy tails or outliers, which can impact statistical analyses and model assumptions. Low kurtosis (platykurtic) indicates lighter tails and fewer outliers, suggesting a more uniform distribution of data.
Applications of Kurtosis
Kurtosis is used in various fields to understand data distribution and identify potential outliers. Some common applications include:
Finance
In finance, kurtosis is used to assess the risk and return of investment portfolios. High kurtosis in asset returns indicates a higher probability of extreme returns, which can impact risk management and investment strategies.
Quality Control
In quality control, kurtosis helps identify deviations from the expected process performance. High kurtosis in process data may indicate the presence of defects or irregularities that require attention.
Environmental Science
Environmental scientists use kurtosis to analyze the distribution of environmental data, such as pollutant concentrations. High kurtosis can indicate sporadic pollution events or extreme environmental conditions.
Calculation of Sample Kurtosis
Sample kurtosis is an estimate of population kurtosis and is calculated using the following formula:
\[ g_2 = \frac{N(N+1)}{(N-1)(N-2)(N-3)} \sum_{i=1}^{N} \left( \frac{(X_i - \bar{X})^4}{s^4} \right) - \frac{3(N-1)^2}{(N-2)(N-3)} \]
where: - \(g_2\) is the sample kurtosis. - \(N\) is the number of observations. - \(X_i\) represents each individual observation. - \(\bar{X}\) is the mean of the observations. - \(s\) is the sample standard deviation.
Kurtosis in Statistical Tests
Kurtosis is often used in statistical tests to assess the normality of data. Tests such as the Jarque-Bera test and the D'Agostino-Pearson test use kurtosis and skewness to determine if a dataset deviates from a normal distribution.
Limitations of Kurtosis
While kurtosis is a useful measure, it has limitations. It is sensitive to sample size and can be influenced by extreme values. Additionally, kurtosis alone does not provide a complete picture of data distribution and should be used in conjunction with other statistical measures.
Conclusion
Kurtosis is a crucial statistical measure that provides insights into the shape of data distributions. By understanding kurtosis, researchers and analysts can better interpret data, identify outliers, and make informed decisions in various fields.
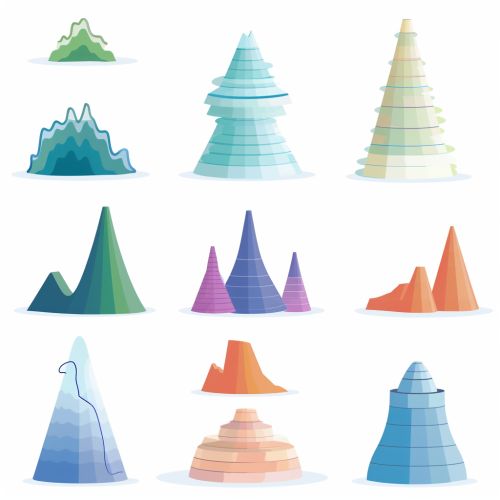
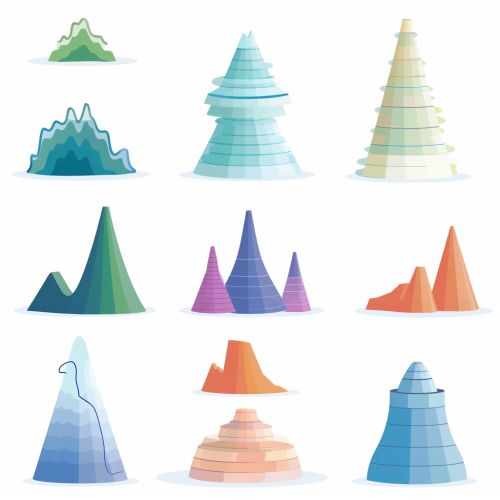