Kolmogorov-Smirnov test
Introduction
The Kolmogorov-Smirnov test (K-S test) is a nonparametric method used in statistical analysis to compare a sample with a reference probability distribution (one-sample K-S test), or to compare two samples (two-sample K-S test). Named after Andrey Kolmogorov and Nikolai Smirnov, it quantifies a distance between the empirical distribution function of the sample and the cumulative distribution function of the reference distribution, or between the empirical distribution functions of two samples.
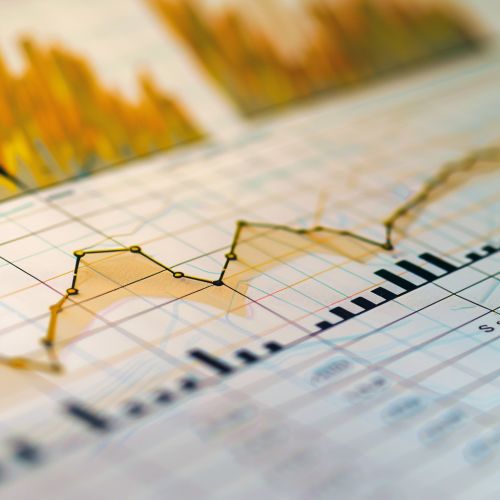
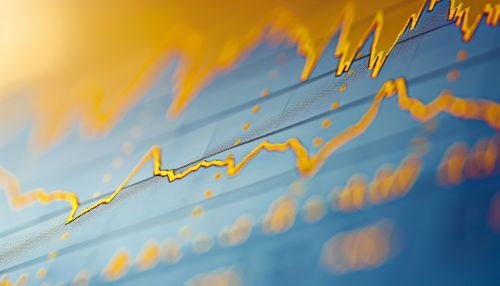
Theoretical Background
The Kolmogorov-Smirnov test is based on the empirical distribution function (EDF). Given an ordered sample (x1, x2, ..., xn), the EDF at a point x is defined as the proportion of sample points less than or equal to x. The Kolmogorov-Smirnov statistic quantifies a distance between the empirical distribution function of the sample and the cumulative distribution function of the reference distribution, or between the empirical distribution functions of two samples.
One-sample K-S Test
The one-sample K-S test compares the empirical distribution function of a sample with the cumulative distribution function of a reference distribution. The null hypothesis is that the sample is drawn from the reference distribution. In the case of a known distribution function, the K-S test is exact. In the case of an estimated distribution function, critical values are not available, and the estimated distribution function must be compared with the Kolmogorov distribution.
Two-sample K-S Test
The two-sample K-S test is one of the most useful and general nonparametric methods for comparing two samples, as it is sensitive to differences in both location and shape of the empirical cumulative distribution functions of the two samples. The null hypothesis is that the samples are drawn from the same distribution (they are identical).
Assumptions and Limitations
The K-S test has several assumptions and limitations that must be considered when it's used. The test is most powerful when used on continuous, unimodal distributions that do not have a significant number of repeated data points. The K-S test is not valid for ordinal data or for data that includes repeated measurements.
Applications
The K-S test is widely used in fields such as physics, earth science, manufacturing, finance, and research. It is a valuable tool for comparing theoretical models with empirical data, or comparing empirical data from different experiments or different conditions.