Chi-Squared Test
Introduction
The Chi-Squared Test is a statistical hypothesis test that is used to determine whether there is a significant association between two categorical variables in a sample. Named after its creator, Karl Pearson, the test is based on the use of the Chi-squared distribution, which is a theoretical probability distribution of a sum of the squares of independent standard normal random variables.
Background and History
The Chi-Squared Test was first introduced by Karl Pearson in the early 20th century. Pearson, a prominent figure in the field of statistics, developed the test as a method to assess the goodness of fit of observed data to a theoretical model. The test has since become a fundamental tool in statistical analysis, widely used in fields such as biology, psychology, sociology, and market research.
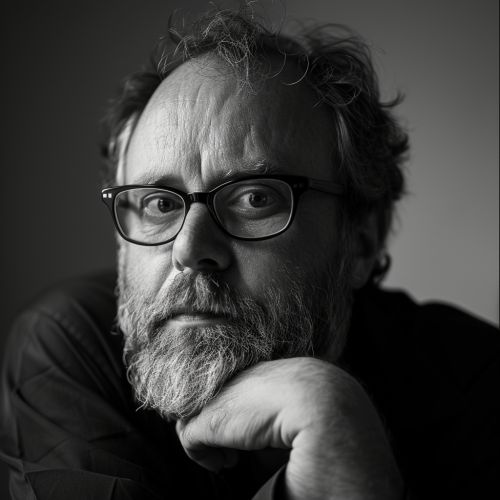
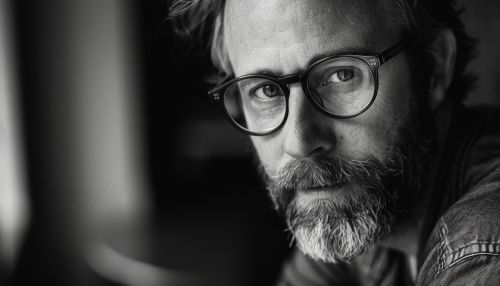
Theory and Calculation
The Chi-Squared Test is based on the comparison of observed frequencies (O) and expected frequencies (E) in a contingency table. The test statistic, denoted as X², is calculated using the formula:
X² = Σ [ (O - E)² / E ]
where the sum is over all cells in the contingency table. The expected frequencies are calculated under the assumption of the null hypothesis, which states that the variables are independent. If the observed frequencies significantly deviate from the expected frequencies, the null hypothesis is rejected, indicating a significant association between the variables.
Assumptions and Conditions
The Chi-Squared Test relies on certain assumptions and conditions for its validity. These include:
1. The data are categorical, not numerical. 2. The observations are independent, meaning that the outcome of one observation does not influence the outcome of another. 3. The sample size is sufficiently large. A common rule of thumb is that all expected frequencies should be at least 5.
Violation of these assumptions can lead to misleading results. Therefore, it is important to ensure these conditions are met before applying the Chi-Squared Test.
Applications and Examples
The Chi-Squared Test is widely used in various fields of study. In biology, it is often used to test the fit of observed genetic frequencies to expected frequencies according to Mendelian inheritance laws. In psychology and sociology, the test is used to examine the relationship between different categorical variables, such as gender and career choice. In market research, the test is used to analyze consumer preferences and behavior.
Limitations and Criticisms
Despite its widespread use, the Chi-Squared Test has been subject to several criticisms. One limitation is that it can only be used with categorical data, not numerical data. Another criticism is that the test is sensitive to sample size. With large samples, even small deviations from the expected frequencies can result in rejection of the null hypothesis, potentially leading to false positives.