Interval data
Interval Data
Interval data is a type of quantitative data that is measured along a scale, in which each point is placed at an equal distance from one another. This type of data is essential in various fields such as statistics, psychology, and the natural sciences, as it allows for the measurement of the difference between values. Unlike ordinal data, interval data not only indicates the order but also the exact difference between the values.
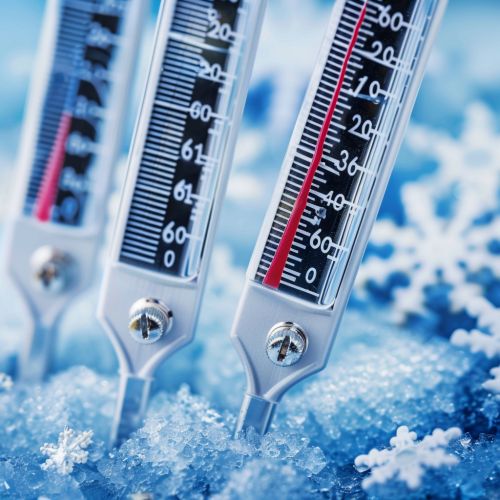
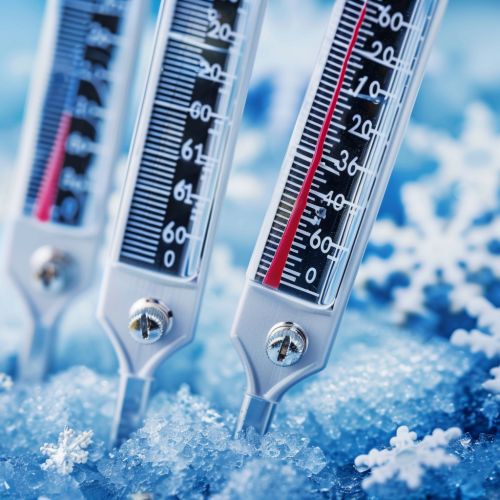
Characteristics of Interval Data
Interval data possesses several unique characteristics that distinguish it from other types of data:
1. **Equal Intervals**: The intervals between the values are consistent. For example, the difference between 10 and 20 degrees Celsius is the same as the difference between 20 and 30 degrees Celsius. 2. **Arbitrary Zero Point**: Interval scales have an arbitrary zero point, meaning that zero does not indicate the absence of the quantity being measured. For instance, 0 degrees Celsius does not mean there is no temperature; it is simply a point on the scale. 3. **Addition and Subtraction**: These operations are meaningful with interval data. You can add or subtract values to determine the difference between them. 4. **No True Zero**: Unlike ratio data, interval data does not have a true zero point which means that ratios of interval data are not meaningful. For example, 20 degrees Celsius is not twice as hot as 10 degrees Celsius.
Examples of Interval Data
Interval data can be found in various domains:
- **Temperature**: Measured in degrees Celsius or Fahrenheit, temperature is a classic example of interval data. - **IQ Scores**: Intelligence Quotient (IQ) scores are measured on an interval scale. - **Calendar Dates**: Dates are measured on an interval scale where the difference between dates is consistent.
Statistical Analysis of Interval Data
Interval data allows for a wide range of statistical analyses. Some of the common methods include:
1. **Descriptive Statistics**: Measures such as mean, median, and standard deviation can be calculated. 2. **Inferential Statistics**: Techniques such as t-tests and ANOVA can be applied to interval data. 3. **Correlation and Regression**: Pearson correlation and linear regression analyses are appropriate for interval data.
Measurement and Data Collection
Collecting interval data requires precise measurement tools that ensure equal intervals between values. Instruments such as thermometers, standardized tests, and calibrated scales are commonly used.
Applications of Interval Data
Interval data is crucial in various fields:
- **Psychology**: Used in psychological testing and assessments. - **Education**: Standardized test scores are often interval data. - **Meteorology**: Temperature measurements are essential for weather forecasting.
Limitations of Interval Data
While interval data is highly useful, it does have limitations:
- **No True Zero**: The lack of a true zero point limits the types of analyses that can be performed. - **Interpretation**: Care must be taken in interpreting results, as the arbitrary zero point can lead to misunderstandings.