Quantitative Data
Introduction
Quantitative data refers to the type of data that can be counted, measured, and expressed using numbers. This type of data is often collected in research, surveys, and statistical studies where the results can be presented in tables, charts, graphs, or other visual representations. Quantitative data provides a numerical basis for decision-making, predictions, and cause-and-effect analysis in various fields such as economics, sociology, psychology, and data science.
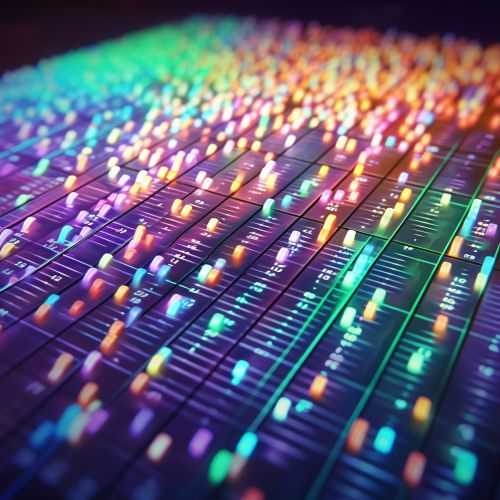
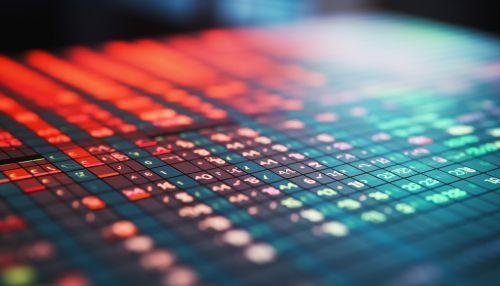
Types of Quantitative Data
Quantitative data can be classified into two main types: discrete and continuous.
Discrete Data
Discrete data, also known as categorical data, represents information that can be divided into distinct, separate values. Examples of discrete data include the number of students in a class, the number of cars in a parking lot, or the number of books in a library.
Continuous Data
Continuous data represents measurements and therefore their values can take on any value within a finite or infinite interval. Examples of continuous data include height, weight, temperature, or time.
Collection of Quantitative Data
Quantitative data is typically collected through methods that provide structured, numerical data. These methods can include:
Surveys
Surveys are a common method for collecting quantitative data. They often include questions that require numerical responses, such as rating scales or multiple-choice questions.
Experiments
Experiments are another common method for collecting quantitative data. In an experiment, the researcher manipulates one variable to determine its effect on another variable.
Observations
Observations can also yield quantitative data. For example, a researcher might count the number of times a specific behavior occurs within a set period.
Analysis of Quantitative Data
Quantitative data analysis involves the use of statistical methods to interpret data. This can include descriptive statistics, inferential statistics, or predictive analytics.
Descriptive Statistics
Descriptive statistics summarize and organize characteristics of a data set. Measures of central tendency (mean, median, mode) and measures of dispersion (range, variance, standard deviation) are common descriptive statistics.
Inferential Statistics
Inferential statistics use a random sample of data from a population to describe and make inferences about the population. Common inferential statistics include regression analysis, analysis of variance (ANOVA), and hypothesis testing.
Predictive Analytics
Predictive analytics use statistical techniques to predict future outcomes based on historical data. This can include machine learning algorithms, regression models, and time series analysis.
Applications of Quantitative Data
Quantitative data is used in a variety of fields, including:
Business
In business, quantitative data can be used to measure performance, analyze market trends, and make financial forecasts.
Healthcare
In healthcare, quantitative data can be used to track patient outcomes, analyze treatment effectiveness, and predict disease spread.
Education
In education, quantitative data can be used to measure student performance, analyze teaching effectiveness, and evaluate program success.
Limitations of Quantitative Data
While quantitative data provides valuable numerical insights, it also has limitations. These include:
Lack of Context
Quantitative data often lacks the context that qualitative data provides. It can tell you how many, how much, or how often, but it may not explain why or how.
Difficulty in Capturing Complexity
Quantitative data may not fully capture complex, nuanced phenomena. For example, a survey might quantify how many people feel a certain way, but it might not capture the depth and complexity of those feelings.
Potential for Misinterpretation
Without proper context or understanding, quantitative data can be misinterpreted. For example, a high correlation between two variables does not necessarily imply causation.
Conclusion
Quantitative data plays a crucial role in many fields, providing a numerical basis for decision-making, predictions, and cause-and-effect analysis. While it has its limitations, the insights it provides are invaluable in our data-driven world.