Factorial Design
Introduction
Factorial design is a statistical model used in experiments where the impact of different variables is considered. It is a type of experimental design that allows researchers to measure the effect of two or more inputs, or factors, on an outcome. The factorial design also allows for the investigation of the interactions between different factors, which can provide valuable insights into the complex relationships between variables.
Overview
Factorial designs are widely used in a range of scientific fields, including psychology, biology, engineering, and social sciences. They are particularly useful in situations where it is necessary to study the effect of more than one independent variable simultaneously, and where the effects of different variables may interact with each other in complex ways.
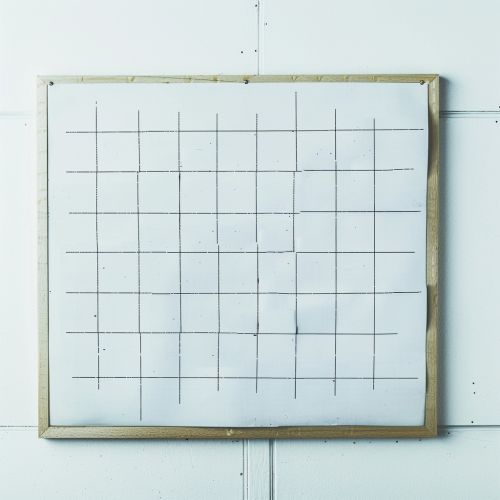
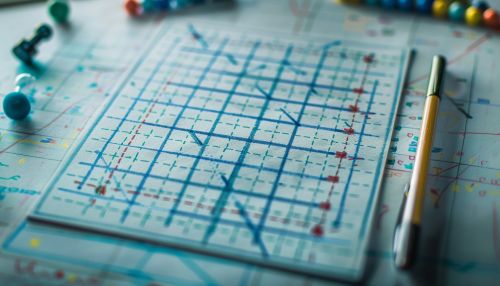
Types of Factorial Design
There are several types of factorial designs, including full factorial designs, fractional factorial designs, and mixed factorial designs. Each of these designs has its own strengths and weaknesses, and the choice of design depends on the specific research question and the resources available.
Full Factorial Design
A full factorial design is one in which all possible combinations of the levels of the factors are investigated. For example, in a 2x2 factorial design with two factors each at two levels, there would be four experimental conditions. This type of design is very comprehensive, but can be resource-intensive if there are many factors or levels.
Fractional Factorial Design
A fractional factorial design is a subset of a full factorial design, in which only a fraction of the possible combinations of factors are investigated. This type of design can be more efficient than a full factorial design, particularly when there are many factors, but it may not provide as much information about the interactions between factors.
Mixed Factorial Design
A mixed factorial design is one in which some factors are manipulated as within-subjects factors (i.e., each participant is exposed to all levels of the factor), while others are manipulated as between-subjects factors (i.e., each participant is exposed to only one level of the factor). This type of design can provide a balance between the comprehensiveness of a full factorial design and the efficiency of a fractional factorial design.
Applications
Factorial designs are used in a wide range of fields and contexts. For example, in psychology, they might be used to investigate the effects of different types of therapy and different durations of therapy on patient outcomes. In engineering, they might be used to optimize the performance of a system by varying different parameters. In social sciences, they might be used to study the effects of different social interventions on various outcomes.
Advantages and Disadvantages
Factorial designs have several advantages. They allow for the investigation of the effects of multiple factors simultaneously, and they can provide information about the interactions between factors. They are also efficient, in the sense that they allow for the investigation of multiple factors with fewer participants or experimental units than would be required with separate experiments for each factor.
However, factorial designs also have some disadvantages. They can be complex and difficult to analyze, particularly when there are many factors or when the interactions between factors are complex. They can also be resource-intensive, particularly for full factorial designs with many factors or levels.
Conclusion
Factorial design is a powerful tool for experimental research, allowing for the simultaneous investigation of multiple factors and their interactions. While they can be complex and resource-intensive, they provide valuable insights into the complex relationships between variables, and are widely used in a range of scientific fields.