Data Science Applications in Energy Management
Introduction
Data science has emerged as a transformative force in various industries, and its applications in energy management are particularly noteworthy. By leveraging advanced analytics, machine learning, and big data technologies, data science enables more efficient, reliable, and sustainable energy systems. This article delves into the sophisticated applications of data science in energy management, exploring the methodologies, technologies, and real-world implementations that are shaping the future of the energy sector.
Historical Context
The integration of data science in energy management is a relatively recent development, driven by the advent of Big Data and the proliferation of IoT devices. Historically, energy management relied on manual data collection and rudimentary analysis. The transition to digital and automated systems began in the late 20th century, with the introduction of SCADA systems. However, it wasn't until the 21st century that data science began to play a pivotal role, thanks to advancements in computational power and data storage.
Key Concepts in Data Science for Energy Management
Machine Learning and Predictive Analytics
Machine learning algorithms are at the core of data science applications in energy management. These algorithms analyze historical data to predict future energy consumption patterns, optimize energy distribution, and identify potential system failures. Techniques such as regression, time series analysis, and neural networks are commonly employed.
Big Data Analytics
The energy sector generates vast amounts of data from various sources, including smart meters, sensors, and grid infrastructure. Big data analytics enables the processing and analysis of this data to extract valuable insights. Techniques such as data mining and cluster analysis are used to identify patterns and trends that inform decision-making processes.
IoT and Smart Grids
The integration of IoT devices in energy management systems has led to the development of smart grids. These grids use real-time data from connected devices to optimize energy distribution, reduce losses, and enhance reliability. Data science plays a crucial role in analyzing the data collected from IoT devices to improve grid performance.
Applications in Energy Management
Demand Forecasting
Accurate demand forecasting is essential for efficient energy management. Data science techniques, such as machine learning and statistical modeling, are used to predict energy demand based on historical consumption data, weather patterns, and economic indicators. This enables energy providers to plan and allocate resources more effectively.
Energy Efficiency Optimization
Data science helps identify opportunities for improving energy efficiency in industrial, commercial, and residential settings. By analyzing energy consumption data, data scientists can pinpoint inefficiencies and recommend corrective actions. Techniques such as energy audits and benchmarking are commonly used.
Renewable Energy Integration
The integration of renewable energy sources, such as solar and wind, into the grid presents unique challenges. Data science aids in predicting the variability of renewable energy generation and optimizing its integration into the grid. Forecasting models and optimization algorithms are employed to balance supply and demand.
Fault Detection and Maintenance
Predictive maintenance is a critical application of data science in energy management. By analyzing data from sensors and equipment, data scientists can predict potential failures and schedule maintenance before issues arise. Techniques such as anomaly detection and predictive modeling are used to enhance system reliability.
Energy Trading and Market Analysis
Data science is also used in energy trading and market analysis. By analyzing market data, weather forecasts, and consumption patterns, data scientists can develop trading strategies and optimize market participation. Techniques such as algorithmic trading and sentiment analysis are employed.
Case Studies
Smart Grid Implementation in Europe
Several European countries have implemented smart grids that leverage data science for enhanced energy management. For example, the UK has deployed smart meters across the country, enabling real-time monitoring and analysis of energy consumption. This has led to significant improvements in energy efficiency and reliability.
Renewable Energy Forecasting in the United States
In the United States, data science is used extensively for forecasting renewable energy generation. Companies like Google and IBM have developed advanced forecasting models that predict solar and wind energy production with high accuracy. These models help grid operators manage the variability of renewable energy sources.
Predictive Maintenance in Asia
In Asia, predictive maintenance is a key focus area for energy companies. For instance, China's State Grid Corporation uses data science to monitor and maintain its vast network of transmission lines and substations. This has resulted in reduced downtime and improved system reliability.
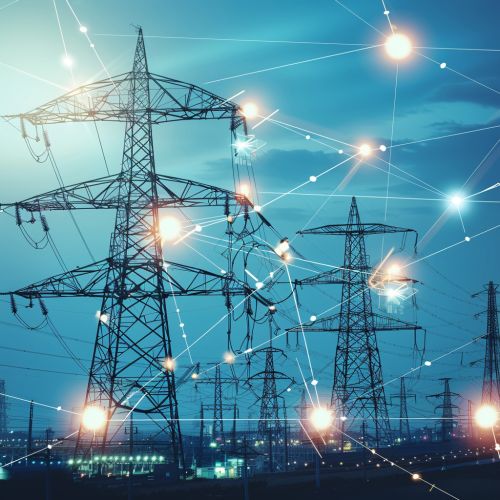
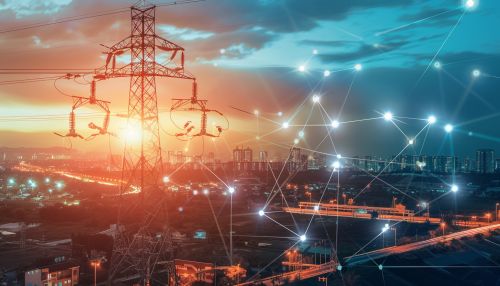
Challenges and Future Directions
Data Quality and Integration
One of the primary challenges in applying data science to energy management is ensuring data quality and integration. Inconsistent or incomplete data can lead to inaccurate analyses and suboptimal decisions. Efforts are underway to standardize data collection and improve data integration across different systems.
Cybersecurity
The increasing reliance on data and connected devices in energy management raises cybersecurity concerns. Protecting sensitive data and ensuring the security of critical infrastructure are paramount. Data science techniques, such as encryption and intrusion detection systems, are employed to enhance cybersecurity.
Scalability
Scalability is another challenge, particularly for large-scale energy systems. Data science solutions must be scalable to handle the vast amounts of data generated by modern energy systems. Advances in cloud computing and distributed computing are helping address this challenge.
Future Directions
The future of data science in energy management is promising, with ongoing research and development aimed at enhancing existing applications and exploring new ones. Areas of interest include artificial intelligence, blockchain for energy trading, and quantum computing for complex optimization problems.
Conclusion
Data science is revolutionizing energy management by enabling more efficient, reliable, and sustainable energy systems. Through advanced analytics, machine learning, and big data technologies, data science provides valuable insights that drive decision-making and optimize energy operations. As the energy sector continues to evolve, the role of data science will undoubtedly become even more critical.
See Also
- Machine Learning
- Big Data
- Smart Grid
- Renewable Energy
- Predictive Maintenance
- Energy Trading
- Cybersecurity