Correspondence Analysis
Introduction
Correspondence Analysis (CA) is a statistical technique used in the field of data analysis and statistics. It is a method used to graphically represent the relationships among categorical variables in a dataset. The technique is particularly useful when dealing with large datasets with many variables, as it simplifies the data and makes it easier to interpret.
History
The concept of Correspondence Analysis was first introduced by the French mathematician Jean-Paul Benzécri in the 1960s. Benzécri was a pioneer in the field of multivariate statistical analysis and his work has had a significant impact on the field of data analysis.
Theory
Correspondence Analysis is based on the theory of principal component analysis (PCA). The main idea behind PCA is to reduce the dimensionality of a dataset while retaining as much of the variability in the data as possible. In the case of Correspondence Analysis, the data is represented in a low-dimensional space, typically two or three dimensions, which allows for a visual interpretation of the relationships among the variables.
Methodology
The first step in Correspondence Analysis is to construct a contingency table, which is a type of cross-tabulation that shows the frequency distribution of the variables. The rows of the table represent one variable, and the columns represent another variable. The cells in the table contain the frequencies of each combination of variable levels.
The next step is to compute the row and column totals, and the grand total of the table. These totals are used to calculate the expected frequencies under the assumption of independence. The difference between the observed and expected frequencies is then used to compute the chi-square statistic, which measures the degree of association between the variables.
The chi-square statistic is then decomposed into a set of orthogonal (uncorrelated) factors, each of which represents a dimension in the data. The factors are ordered by their contribution to the total chi-square statistic, with the first factor accounting for the largest proportion of the total.
The final step is to plot the row and column points in the factor space. The distance between points in this space represents the degree of association between the variables. Points that are close together are more strongly associated than points that are far apart.

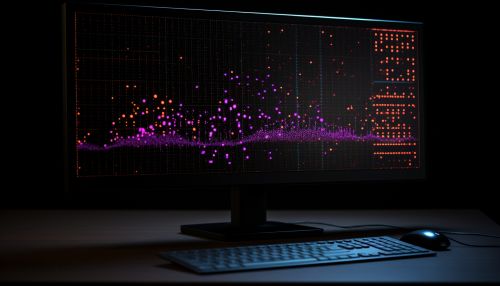
Applications
Correspondence Analysis is widely used in many fields, including sociology, marketing, ecology, and genomics. It is particularly useful in exploratory data analysis, where the goal is to uncover patterns and relationships in the data.
In sociology, for example, Correspondence Analysis can be used to analyze survey data and identify patterns of social behavior. In marketing, it can be used to analyze consumer purchase data and identify patterns of consumer behavior. In ecology, it can be used to analyze species occurrence data and identify patterns of species distribution. And in genomics, it can be used to analyze gene expression data and identify patterns of gene activity.
Advantages and Disadvantages
One of the main advantages of Correspondence Analysis is that it provides a visual representation of the data, which can make it easier to interpret. It is also a flexible method that can be used with any type of categorical data.
However, Correspondence Analysis also has some disadvantages. One of the main disadvantages is that it can be difficult to interpret the results, especially when dealing with large datasets with many variables. It can also be sensitive to outliers, which can distort the results.