Weak AI
Introduction
Weak AI, also known as narrow AI, is a form of artificial intelligence that is designed to perform a narrow task such as voice recognition or driving a car. Unlike strong AI, which is designed to perform any intellectual task that a human being can do, weak AI systems are limited to the specific tasks for which they are programmed[^1^].
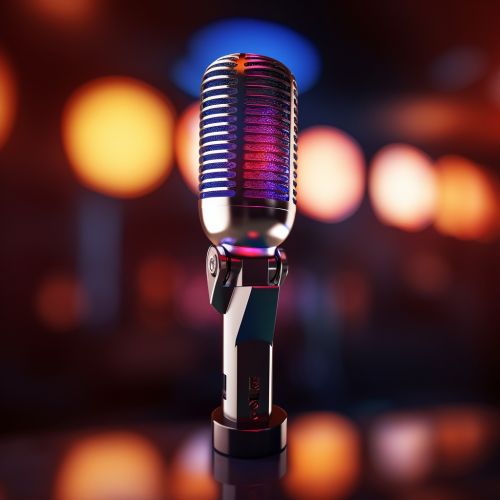
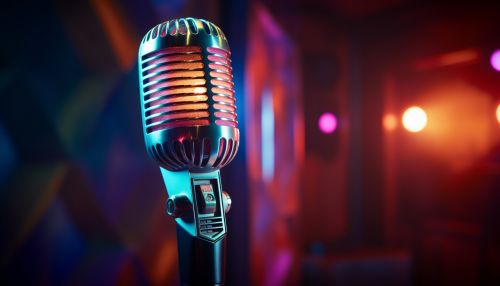
History and Development
The concept of weak AI was first introduced by the philosopher John Searle in his Chinese Room Argument[^2^]. He used the term to differentiate between AI systems that simply simulate human cognition (weak AI) and those that actually have the ability to understand and learn in the same way that humans do (strong AI).
The development of weak AI systems has been driven by advancements in machine learning and data analysis techniques. These systems are often based on algorithms that can learn from and make predictions or decisions based on data[^3^].
Characteristics
Weak AI systems are characterized by their ability to perform specific tasks in a way that appears intelligent. However, these systems do not possess true understanding or consciousness. They are designed to solve a specific problem and their functionality is confined to that particular problem[^4^].
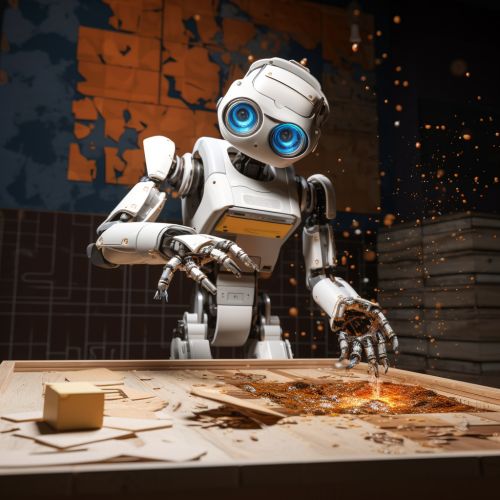
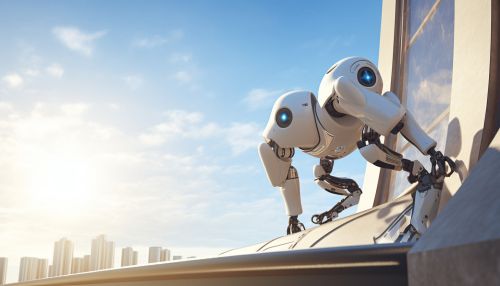
Applications
Weak AI has a wide range of applications in various fields. Some of the most common applications include:
- Speech recognition: This is used in devices like Amazon's Alexa and Apple's Siri to understand and respond to voice commands[^5^].
- Image recognition: This is used in applications like Google Photos to identify and categorize images[^6^].
- Natural language processing: This is used in applications like Google Translate to understand and translate text[^7^].
- Autonomous vehicles: This is used in self-driving cars to navigate and make decisions based on the surrounding environment[^8^].
Limitations
While weak AI systems can perform specific tasks very effectively, they have several limitations. These systems lack the ability to understand context outside of their specific task, and they cannot make decisions beyond their programming[^9^]. Additionally, they are unable to learn or adapt to new situations without being reprogrammed[^10^].
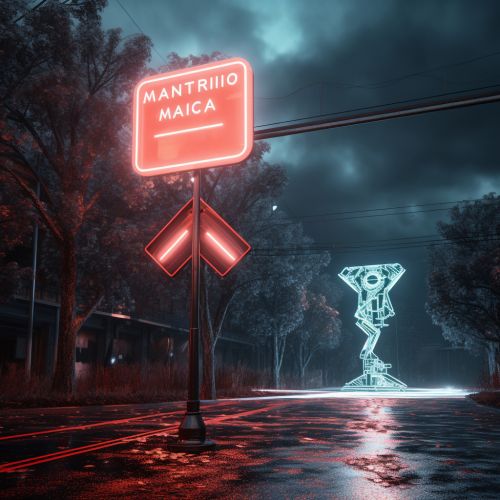
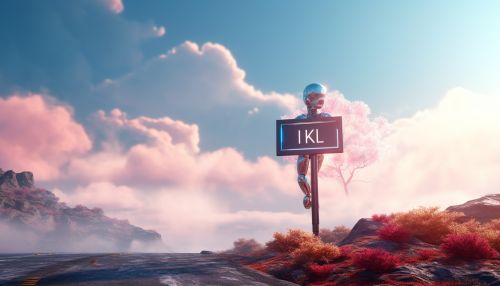
Future of Weak AI
The future of weak AI is likely to involve advancements in machine learning and data analysis techniques, which will enable these systems to perform their specific tasks with greater accuracy and efficiency[^11^]. However, the development of strong AI systems that can understand, learn, and adapt in the same way that humans do remains a significant challenge[^12^].
See Also
References
[^1^]: Russell, S., & Norvig, P. (2016). Artificial Intelligence: A Modern Approach. Pearson. [^2^]: Searle, J. (1980). Minds, brains, and programs. Behavioral and brain sciences, 3(3), 417-424. [^3^]: Bishop, C. M. (2006). Pattern Recognition and Machine Learning. Springer. [^4^]: Poole, D., & Mackworth, A. (2010). Artificial Intelligence: foundations of computational agents. Cambridge University Press. [^5^]: Hinton, G., Deng, L., Yu, D., Dahl, G. E., Mohamed, A. R., Jaitly, N., ... & Kingsbury, B. (2012). Deep neural networks for acoustic modeling in speech recognition: The shared views of four research groups. IEEE Signal Processing Magazine, 29(6), 82-97. [^6^]: Krizhevsky, A., Sutskever, I., & Hinton, G. E. (2012). ImageNet classification with deep convolutional neural networks. In Advances in neural information processing systems (pp. 1097-1105). [^7^]: Sutskever, I., Vinyals, O., & Le, Q. V. (2014). Sequence to sequence learning with neural networks. In Advances in neural information processing systems (pp. 3104-3112). [^8^]: Bojarski, M., Del Testa, D., Dworakowski, D., Firner, B., Flepp, B., Goyal, P., ... & Zhang, X. (2016). End to end learning for self-driving cars. arXiv preprint arXiv:1604.07316. [^9^]: Bostrom, N. (2014). Superintelligence: Paths, dangers, strategies. OUP Oxford. [^10^]: Domingos, P. (2015). The master algorithm: How the quest for the ultimate learning machine will remake our world. Basic Books. [^11^]: Goodfellow, I., Bengio, Y., & Courville, A. (2016). Deep learning. MIT press. [^12^]: Searle, J. (1980). Minds, brains, and programs. Behavioral and brain sciences, 3(3), 417-424.