Visualizers
Introduction
Visualizers, also known as visualization software, are tools that transform raw data into visual or graphical representations. They are widely used in various fields, such as data science, information technology, business intelligence, and education, to interpret complex data sets and convey information in a more understandable and accessible format.
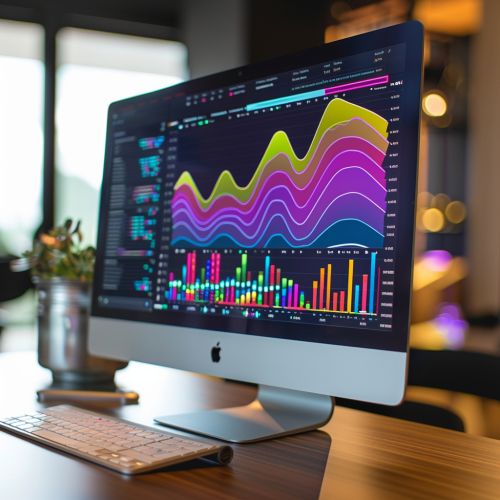
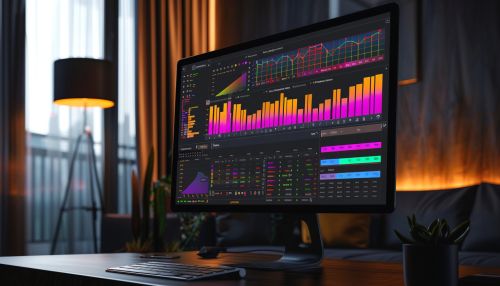
History of Visualizers
The history of visualizers traces back to the 17th century when René Descartes introduced the Cartesian coordinate system. This system, which uses two or three numerical coordinates to uniquely determine the position of a point or geometric element, laid the foundation for data visualization. Over the centuries, the field of data visualization has evolved, leading to the development of modern visualizers.
Types of Visualizers
There are various types of visualizers, each designed to represent different types of data and serve different purposes. They can be broadly classified into static visualizers and interactive visualizers.
Static Visualizers
Static visualizers generate fixed images that represent data. These images do not change or interact with the user. Examples of static visualizers include bar graphs, pie charts, scatter plots, and line graphs. They are typically used in reports, presentations, and publications where interaction is not required.
Interactive Visualizers
Interactive visualizers, on the other hand, allow users to manipulate the visualization and explore different views of the data. They often include features such as zooming, panning, filtering, and highlighting, which enable users to interact with the data and gain deeper insights. Examples of interactive visualizers include Tableau, Power BI, and D3.js.
Uses of Visualizers
Visualizers are used in a wide range of fields for various purposes, including data analysis, decision-making, education, and communication.
Data Analysis
In data analysis, visualizers are used to explore and interpret complex data sets. They help analysts identify patterns, trends, and outliers in the data, which may not be apparent in raw, numerical data.
Decision-Making
In decision-making, visualizers are used to present data in a way that is easy to understand, enabling decision-makers to make informed decisions based on the data. They help simplify complex data and present it in a visually appealing way, making it easier for decision-makers to grasp the information and make decisions accordingly.
Education
In education, visualizers are used to teach complex concepts and ideas. They help students understand abstract concepts by presenting them in a visual format, making learning more engaging and effective.
Communication
In communication, visualizers are used to convey information in a clear and concise manner. They help presenters communicate complex information in a simple, visual format, making it easier for the audience to understand and remember the information.
Design Principles of Visualizers
The design of visualizers is guided by several principles to ensure that the visualizations are effective and user-friendly. These principles include simplicity, clarity, consistency, and relevance.
Simplicity
Simplicity refers to the principle that visualizations should be as simple as possible, without sacrificing the necessary information. This means avoiding unnecessary elements and complexity that could confuse the user or distract from the data.
Clarity
Clarity refers to the principle that visualizations should be clear and easy to understand. This means using clear labels, legends, and titles, and choosing colors and shapes that are easy to distinguish.
Consistency
Consistency refers to the principle that visualizations should be consistent in their design and use of elements. This means using the same colors, shapes, and sizes for the same types of data, and keeping the design consistent across different visualizations.
Relevance
Relevance refers to the principle that visualizations should be relevant to the data and the user's needs. This means choosing the right type of visualization for the data, and customizing the visualization to suit the user's needs and preferences.
Future of Visualizers
The future of visualizers looks promising, with advancements in technology and data science paving the way for more sophisticated and interactive visualizations. With the rise of big data and machine learning, visualizers are expected to become more powerful and capable of handling larger and more complex data sets. Moreover, with the increasing demand for data-driven decision-making, the use of visualizers is likely to become more prevalent in various fields.