The Role of Artificial Intelligence in Predictive Analytics
Introduction
Artificial intelligence (AI) plays a crucial role in the field of predictive analytics, a branch of advanced analytics that uses both new and historical data to forecast future activity, behavior, and trends. Predictive analytics involves applying statistical algorithms and machine-learning techniques to data in order to determine future outcomes. The role of AI in predictive analytics is to automate and refine this process, enabling more accurate predictions and insights.
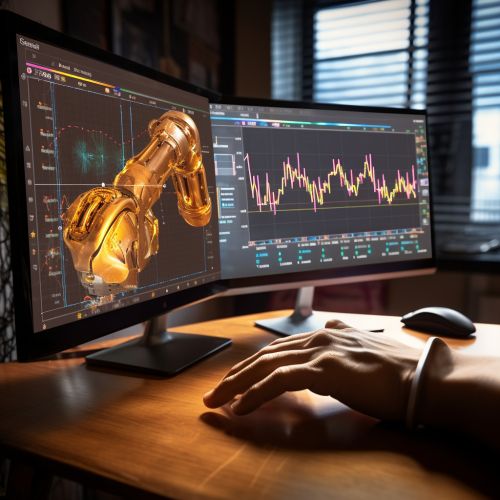

The Intersection of AI and Predictive Analytics
AI and predictive analytics intersect in their shared goal of extracting valuable information from data. Both fields aim to understand patterns and make predictions based on these patterns. AI, however, takes this a step further by not only identifying patterns but also learning from them, improving its predictive capabilities over time. This learning aspect of AI, known as machine learning, is particularly important in predictive analytics.
Role of AI in Predictive Analytics
AI plays several roles in predictive analytics, including data mining, statistical modeling, and machine learning. These roles are interconnected and often overlap, as they all contribute to the goal of making accurate predictions based on data.
Data Mining
Data mining is the process of discovering patterns in large data sets. In predictive analytics, AI uses data mining techniques to identify patterns and relationships in historical data that can be used to predict future outcomes. This involves the use of complex algorithms that can analyze large amounts of data quickly and accurately.
Statistical Modeling
Statistical modeling is the process of creating a mathematical representation of a real-world process or system. In predictive analytics, AI uses statistical models to represent the relationships between different variables in the data. These models can then be used to make predictions about future outcomes.
Machine Learning
Machine learning is a type of AI that enables computers to learn from data without being explicitly programmed. In predictive analytics, machine learning algorithms are used to learn from historical data and make predictions about future outcomes. These algorithms can adapt and improve over time, making them particularly effective for predictive analytics.
Benefits of Using AI in Predictive Analytics
The use of AI in predictive analytics offers several benefits, including increased accuracy, efficiency, and scalability.
Increased Accuracy
AI algorithms can analyze large amounts of data quickly and accurately, leading to more accurate predictions. This is particularly important in fields where accuracy is critical, such as healthcare and finance.
Efficiency
AI can automate the process of predictive analytics, reducing the time and effort required to analyze data and make predictions. This can lead to significant cost savings and increased productivity.
Scalability
AI can handle large amounts of data, making it scalable for large-scale predictive analytics projects. This is particularly important in the era of big data, where organizations often have to analyze massive amounts of data.
Challenges and Limitations of Using AI in Predictive Analytics
Despite its benefits, the use of AI in predictive analytics also presents several challenges and limitations.
Data Quality
The accuracy of AI predictions depends on the quality of the data it is trained on. If the data is incomplete, inaccurate, or biased, the predictions made by the AI will also be flawed.
Overfitting
Overfitting is a common problem in machine learning, where the model becomes too complex and starts to fit the noise in the data rather than the underlying pattern. This can lead to inaccurate predictions.
Transparency and Explainability
AI algorithms are often seen as "black boxes" that make predictions without explaining how they arrived at those predictions. This lack of transparency and explainability can be a problem in fields where understanding the reasoning behind a prediction is important.
Conclusion
AI plays a crucial role in predictive analytics, enabling more accurate and efficient predictions. However, the use of AI also presents several challenges and limitations, including issues with data quality, overfitting, and transparency. Despite these challenges, the benefits of using AI in predictive analytics are significant, and the field is likely to continue to evolve and improve as AI technology advances.