Predictive Analytics
Overview
Predictive analytics is a branch of advanced analytics that uses both new and historical data to forecast activity, behavior, and trends. It involves applying statistical analysis techniques, analytical queries, and automated machine learning algorithms to data sets to create predictive models that place a numerical value, or score, on the likelihood of a particular event happening. Predictive analytics is used in a variety of fields, including insurance, telecommunications, retail, travel, healthcare, pharmaceuticals, and oil and gas, among others.
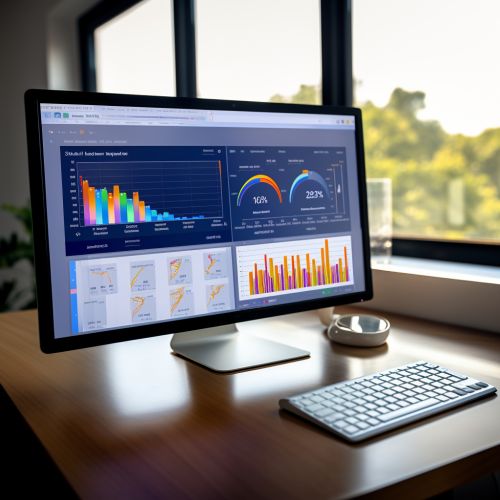
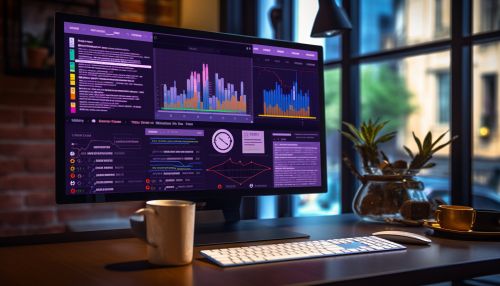
History
The concept of predictive analytics dates back to the 1940s, when statisticians began to use mathematical models to predict the outcomes of various activities. However, it wasn't until the advent of modern computing in the 1960s that predictive analytics began to take shape as a distinct discipline. The development of machine learning in the 1980s and 1990s, and the subsequent rise of data mining, further propelled the field forward. Today, predictive analytics is a key component of business intelligence and big data analytics, and is widely used to inform decision-making in a variety of industries.
Methodology
Predictive analytics involves a number of steps, each of which contributes to the creation of a predictive model. These steps include:
- Data Collection: The first step in predictive analytics is to collect data. This can come from a variety of sources, including transactional data, customer surveys, social media, and external data sources.
- Data Analysis: Once the data has been collected, it is analyzed to identify patterns and relationships. This can involve a variety of statistical techniques, including regression analysis, time series analysis, and cluster analysis.
- Modeling: The next step is to create a predictive model. This involves selecting an appropriate algorithm, such as a decision tree, neural network, or logistic regression, and training it on the data.
- Validation: Once the model has been created, it is validated using a separate set of data. This helps to ensure that the model is accurate and reliable.
- Deployment: Once the model has been validated, it can be deployed and used to make predictions.
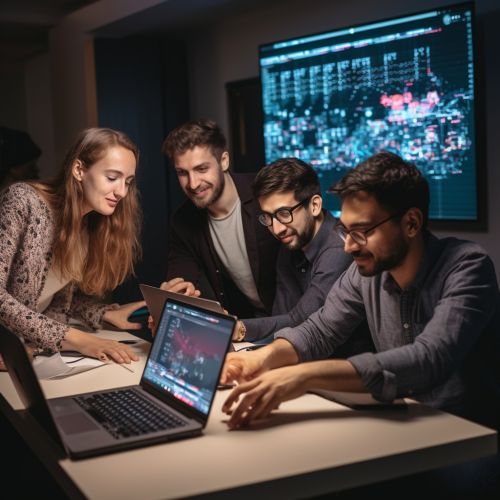
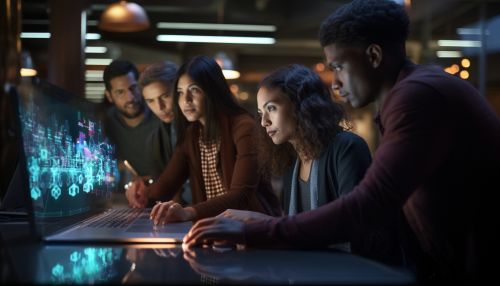
Applications
Predictive analytics has a wide range of applications across various industries. Some of the most common uses include:
- Risk Management: In the financial services industry, predictive analytics is often used to assess the risk of loan defaults or credit card fraud.
- Marketing: Marketers use predictive analytics to identify potential customers, predict customer behavior, and optimize marketing campaigns.
- Healthcare: In healthcare, predictive analytics can be used to predict patient outcomes, identify high-risk patients, and optimize treatment plans.
- Supply Chain Management: Predictive analytics can help companies optimize their supply chains by predicting demand, identifying potential supply chain disruptions, and optimizing inventory levels.
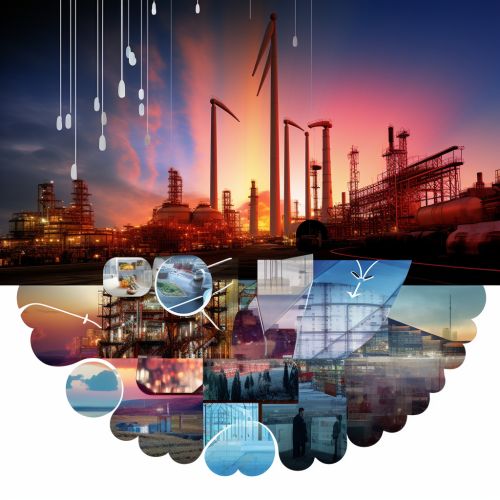
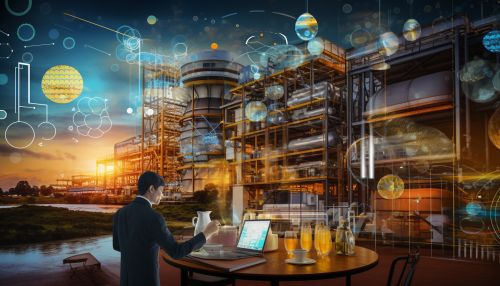
Challenges
While predictive analytics offers many benefits, it also presents a number of challenges. These include:
- Data Quality: The accuracy of predictive models depends on the quality of the data they are trained on. If the data is incomplete, outdated, or biased, the predictions may be inaccurate.
- Model Complexity: Predictive models can be complex and difficult to interpret. This can make it challenging for non-technical stakeholders to understand the predictions and make informed decisions.
- Ethical Considerations: Predictive analytics can raise a number of ethical issues, particularly when it comes to privacy and discrimination. Companies must be careful to use predictive analytics responsibly and in compliance with relevant laws and regulations.
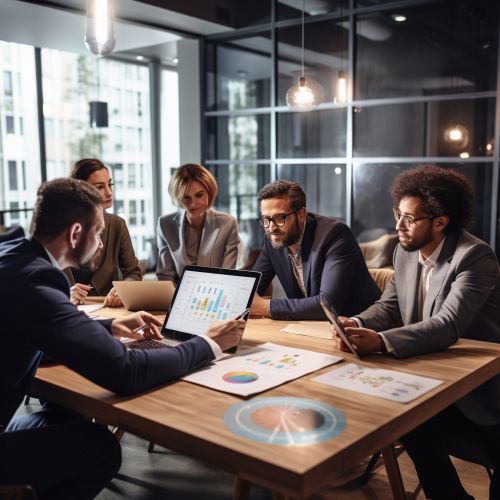
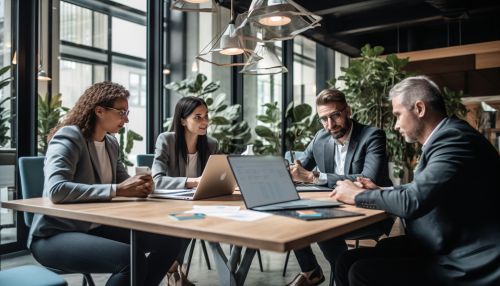
Future Trends
The field of predictive analytics is constantly evolving, driven by advances in technology and changes in the business environment. Some of the key trends shaping the future of predictive analytics include:
- Artificial Intelligence and Machine Learning: Advances in AI and machine learning are making it possible to create more sophisticated predictive models that can handle larger data sets and more complex relationships.
- Big Data: The growth of big data is providing companies with more data to feed into their predictive models, improving their accuracy and reliability.
- Real-Time Analytics: As companies become more digital, they are increasingly able to collect and analyze data in real time, enabling them to make predictions and take action more quickly.

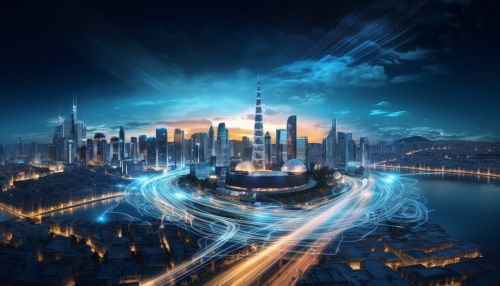