Statistical Science
Introduction
Statistical science, also known as statistical analysis or statistical theory, is a branch of mathematics dealing with the collection, analysis, interpretation, presentation, and organization of data. In applying statistics to a scientific, industrial, or social problem, it is conventional to begin with a statistical population or a statistical model to be studied.
History of Statistical Science
Statistical science as a discipline has its roots in the work of statisticians in the 17th and 18th centuries. The term "statistics" was first used in the late 18th century, and the field has grown and evolved significantly since then. Early statistical methods were used to analyze population data for governmental purposes. Over time, the field expanded to include the collection and analysis of data in many different areas, including agriculture, economics, and the physical sciences.
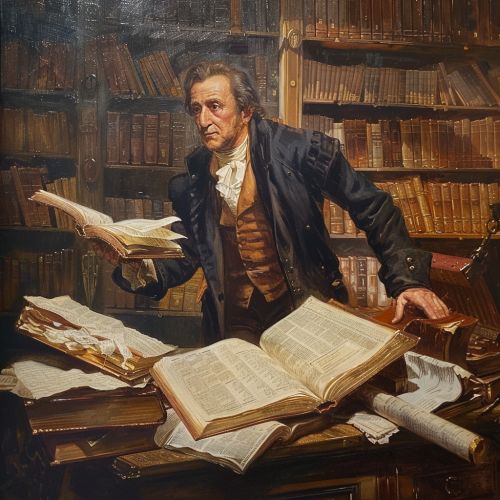
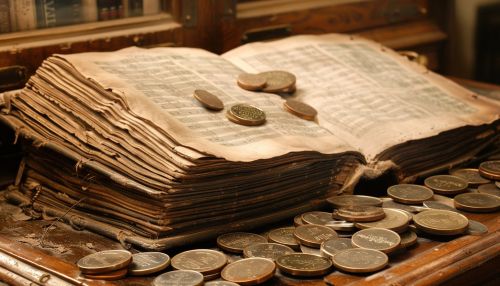
Fundamental Concepts
Statistical science is based on several fundamental concepts, including:
- Probability Theory: This is the branch of mathematics that deals with the analysis of random phenomena. It forms the theoretical basis for statistical science.
- Random Variables: These are variables whose values are subject to variations due to chance. A random variable can take on a set of possible different values (similarly to other mathematical variables), each with an associated probability.
- Statistical Populations: This is a set of similar items or events which is of interest for some question or experiment.
- Statistical Hypothesis Testing: This is a method of making decisions using data, whether from a controlled experiment or an observational study (not controlled).
- Regression Analysis: This is a set of statistical processes for estimating the relationships among variables.
Statistical Methods
Statistical methods are mathematical techniques used for the analysis of large quantities of data. They are used to summarize and describe data, as well as to estimate population parameters and test hypotheses. Some of the most commonly used statistical methods include:
- Descriptive Statistics: These are used to describe the basic features of data in a study. They provide simple summaries about the sample and the measures.
- Inferential Statistics: These are techniques that allow us to use samples to make generalizations about the populations from which the samples were drawn.
- Bayesian Statistics: This is a mathematical procedure that applies probabilities to statistical problems. It provides people the tools to update their beliefs in the evidence of new data.
- Non-Parametric Statistics: These are methods where the data is not required to fit a specified distribution.
Applications of Statistical Science
Statistical science is used in a wide range of fields, including:
- Economic Analysis: Economists use statistical methods to collect and analyze data on economic variables, such as income, employment, and inflation.
- Medical Research: In medicine, statistical techniques are used to evaluate the effectiveness of treatments, understand the causes of disease, and improve health care delivery.
- Psychological Assessment: Psychologists use statistics to understand and interpret the data they collect about human behavior.
- Engineering Design: Engineers use statistical methods to optimize designs and control processes.
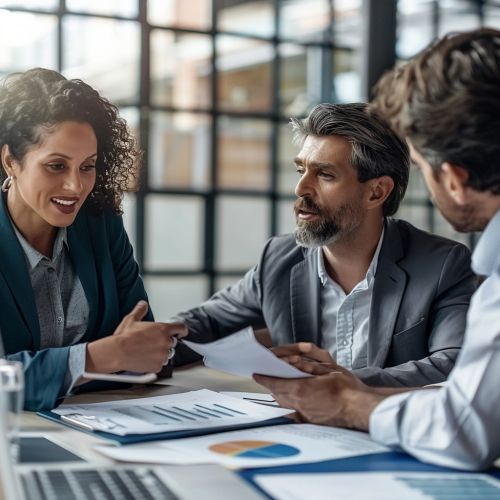
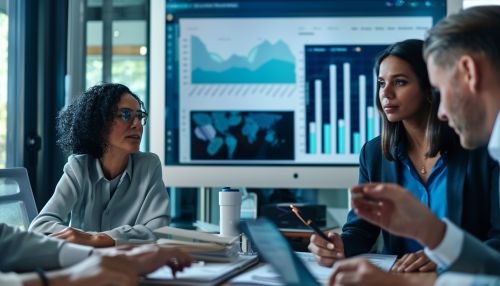
Challenges and Criticisms
Despite its many applications, statistical science has also faced criticism. Some critics argue that statistical methods are often misused, leading to misleading results. Others argue that statistical science is too reliant on assumptions that may not hold true in all cases. Additionally, the increasing complexity of statistical methods has led to concerns about the accessibility of statistical science to non-experts.
Future of Statistical Science
The future of statistical science is likely to be shaped by advances in technology, particularly in the areas of computing power and data collection. These advances are expected to enable the development of new statistical methods and the refinement of existing ones. Furthermore, the increasing availability of large datasets is likely to drive demand for skilled statisticians who can analyze and interpret this data.